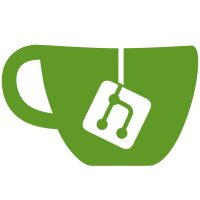
6 changed files with 81 additions and 98 deletions
@ -0,0 +1,61 @@
|
||||
import numpy as np |
||||
|
||||
|
||||
def is_intersect(target_segment, segments): |
||||
for segment in segments: |
||||
start = max(segment['start'], target_segment[0]) |
||||
finish = min(segment['finish'], target_segment[1]) |
||||
if start <= finish: |
||||
return True |
||||
return False |
||||
|
||||
def exponential_smoothing(series, alpha): |
||||
result = [series[0]] |
||||
for n in range(1, len(series)): |
||||
result.append(alpha * series[n] + (1 - alpha) * result[n - 1]) |
||||
return result |
||||
|
||||
def find_steps(array, threshold): |
||||
""" |
||||
Finds local maxima by segmenting array based on positions at which |
||||
the threshold value is crossed. Note that this thresholding is |
||||
applied after the absolute value of the array is taken. Thus, |
||||
the distinction between upward and downward steps is lost. However, |
||||
get_step_sizes can be used to determine directionality after the |
||||
fact. |
||||
Parameters |
||||
---------- |
||||
array : numpy array |
||||
1 dimensional array that represents time series of data points |
||||
threshold : int / float |
||||
Threshold value that defines a step |
||||
Returns |
||||
------- |
||||
steps : list |
||||
List of indices of the detected steps |
||||
""" |
||||
steps = [] |
||||
array = np.abs(array) |
||||
above_points = np.where(array > threshold, 1, 0) |
||||
ap_dif = np.diff(above_points) |
||||
cross_ups = np.where(ap_dif == 1)[0] |
||||
cross_dns = np.where(ap_dif == -1)[0] |
||||
for upi, dni in zip(cross_ups,cross_dns): |
||||
steps.append(np.argmax(array[upi:dni]) + upi) |
||||
return steps |
||||
|
||||
def anomalies_to_timestamp(anomalies): |
||||
for anomaly in anomalies: |
||||
anomaly['start'] = int(anomaly['start'].timestamp() * 1000) |
||||
anomaly['finish'] = int(anomaly['finish'].timestamp() * 1000) |
||||
return anomalies |
||||
|
||||
def segments_box(segments): |
||||
max_time = 0 |
||||
min_time = float("inf") |
||||
for segment in segments: |
||||
min_time = min(min_time, segment['start']) |
||||
max_time = max(max_time, segment['finish']) |
||||
min_time = pd.to_datetime(min_time, unit='ms') |
||||
max_time = pd.to_datetime(max_time, unit='ms') |
||||
return min_time, max_time |
Loading…
Reference in new issue