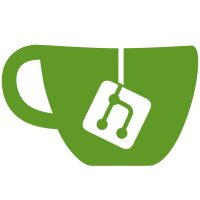
9 changed files with 139 additions and 338 deletions
@ -1,5 +1,5 @@ |
|||||||
from detectors.general_detector import GeneralDetector |
from detectors.general_detector import GeneralDetector |
||||||
from detectors.pattern_detection_model import PatternDetectionModel |
from detectors.pattern_detector import PatternDetector |
||||||
from detectors.peaks_detector import PeaksDetector |
from detectors.peaks_detector import PeaksDetector |
||||||
from detectors.step_detector import StepDetector |
from detectors.step_detector import StepDetector |
||||||
from detectors.jump_detector import Jumpdetector |
from detectors.jump_detector import Jumpdetector |
||||||
|
@ -1,231 +0,0 @@ |
|||||||
|
|
||||||
""" |
|
||||||
Thomas Kahn |
|
||||||
thomas.b.kahn@gmail.com |
|
||||||
""" |
|
||||||
from __future__ import absolute_import |
|
||||||
from math import sqrt |
|
||||||
import multiprocessing as mp |
|
||||||
import numpy as np |
|
||||||
from six.moves import range |
|
||||||
from six.moves import zip |
|
||||||
|
|
||||||
|
|
||||||
def t_scan(L, window = 1e3, num_workers = -1): |
|
||||||
""" |
|
||||||
Computes t statistic for i to i+window points versus i-window to i |
|
||||||
points for each point i in input array. Uses multiple processes to |
|
||||||
do this calculation asynchronously. Array is decomposed into window |
|
||||||
number of frames, each consisting of points spaced at window |
|
||||||
intervals. This optimizes the calculation, as the drone function |
|
||||||
need only compute the mean and variance for each set once. |
|
||||||
Parameters |
|
||||||
---------- |
|
||||||
L : numpy array |
|
||||||
1 dimensional array that represents time series of datapoints |
|
||||||
window : int / float |
|
||||||
Number of points that comprise the windows of data that are |
|
||||||
compared |
|
||||||
num_workers : int |
|
||||||
Number of worker processes for multithreaded t_stat computation |
|
||||||
Defult value uses num_cpu - 1 workers |
|
||||||
Returns |
|
||||||
------- |
|
||||||
t_stat : numpy array |
|
||||||
Array which holds t statistic values for each point. The first |
|
||||||
and last (window) points are replaced with zero, since the t |
|
||||||
statistic calculation cannot be performed in that case. |
|
||||||
""" |
|
||||||
size = L.size |
|
||||||
window = int(window) |
|
||||||
frames = list(range(window)) |
|
||||||
n_cols = (size // window) - 1 |
|
||||||
|
|
||||||
t_stat = np.zeros((window, n_cols)) |
|
||||||
|
|
||||||
if num_workers == 1: |
|
||||||
results = [_t_scan_drone(L, n_cols, frame, window) for frame in frames] |
|
||||||
else: |
|
||||||
if num_workers == -1: |
|
||||||
num_workers = mp.cpu_count() - 1 |
|
||||||
pool = mp.Pool(processes = num_workers) |
|
||||||
results = [pool.apply_async(_t_scan_drone, args=(L, n_cols, frame, window)) for frame in frames] |
|
||||||
results = [r.get() for r in results] |
|
||||||
pool.close() |
|
||||||
|
|
||||||
for index, row in results: |
|
||||||
t_stat[index] = row |
|
||||||
|
|
||||||
t_stat = np.concatenate(( |
|
||||||
np.zeros(window), |
|
||||||
t_stat.transpose().ravel(order='C'), |
|
||||||
np.zeros(size % window) |
|
||||||
)) |
|
||||||
|
|
||||||
return t_stat |
|
||||||
|
|
||||||
|
|
||||||
def _t_scan_drone(L, n_cols, frame, window=1e3): |
|
||||||
""" |
|
||||||
Drone function for t_scan. Not Intended to be called manually. |
|
||||||
Computes t_scan for the designated frame, and returns result as |
|
||||||
array along with an integer tag for proper placement in the |
|
||||||
aggregate array |
|
||||||
""" |
|
||||||
size = L.size |
|
||||||
window = int(window) |
|
||||||
root_n = sqrt(window) |
|
||||||
|
|
||||||
output = np.zeros(n_cols) |
|
||||||
b = L[frame:window+frame] |
|
||||||
b_mean = b.mean() |
|
||||||
b_var = b.var() |
|
||||||
for i in range(window+frame, size-window, window): |
|
||||||
a = L[i:i+window] |
|
||||||
a_mean = a.mean() |
|
||||||
a_var = a.var() |
|
||||||
output[i // window - 1] = root_n * (a_mean - b_mean) / sqrt(a_var + b_var) |
|
||||||
b_mean, b_var = a_mean, a_var |
|
||||||
|
|
||||||
return frame, output |
|
||||||
|
|
||||||
|
|
||||||
def mz_fwt(x, n=2): |
|
||||||
""" |
|
||||||
Computes the multiscale product of the Mallat-Zhong discrete forward |
|
||||||
wavelet transform up to and including scale n for the input data x. |
|
||||||
If n is even, the spikes in the signal will be positive. If n is odd |
|
||||||
the spikes will match the polarity of the step (positive for steps |
|
||||||
up, negative for steps down). |
|
||||||
This function is essentially a direct translation of the MATLAB code |
|
||||||
provided by Sadler and Swami in section A.4 of the following: |
|
||||||
http://www.dtic.mil/dtic/tr/fulltext/u2/a351960.pdf |
|
||||||
Parameters |
|
||||||
---------- |
|
||||||
x : numpy array |
|
||||||
1 dimensional array that represents time series of data points |
|
||||||
n : int |
|
||||||
Highest scale to multiply to |
|
||||||
Returns |
|
||||||
------- |
|
||||||
prod : numpy array |
|
||||||
The multiscale product for x |
|
||||||
""" |
|
||||||
N_pnts = x.size |
|
||||||
lambda_j = [1.5, 1.12, 1.03, 1.01][0:n] |
|
||||||
if n > 4: |
|
||||||
lambda_j += [1.0]*(n-4) |
|
||||||
|
|
||||||
H = np.array([0.125, 0.375, 0.375, 0.125]) |
|
||||||
G = np.array([2.0, -2.0]) |
|
||||||
|
|
||||||
Gn = [2] |
|
||||||
Hn = [3] |
|
||||||
for j in range(1,n): |
|
||||||
q = 2**(j-1) |
|
||||||
Gn.append(q+1) |
|
||||||
Hn.append(3*q+1) |
|
||||||
|
|
||||||
S = np.concatenate((x[::-1], x)) |
|
||||||
S = np.concatenate((S, x[::-1])) |
|
||||||
prod = np.ones(N_pnts) |
|
||||||
for j in range(n): |
|
||||||
n_zeros = 2**j - 1 |
|
||||||
Gz = _insert_zeros(G, n_zeros) |
|
||||||
Hz = _insert_zeros(H, n_zeros) |
|
||||||
current = (1.0/lambda_j[j])*np.convolve(S,Gz) |
|
||||||
current = current[N_pnts+Gn[j]:2*N_pnts+Gn[j]] |
|
||||||
prod *= current |
|
||||||
if j == n-1: |
|
||||||
break |
|
||||||
S_new = np.convolve(S, Hz) |
|
||||||
S_new = S_new[N_pnts+Hn[j]:2*N_pnts+Hn[j]] |
|
||||||
S = np.concatenate((S_new[::-1], S_new)) |
|
||||||
S = np.concatenate((S, S_new[::-1])) |
|
||||||
return prod |
|
||||||
|
|
||||||
|
|
||||||
def _insert_zeros(x, n): |
|
||||||
""" |
|
||||||
Helper function for mz_fwt. Splits input array and adds n zeros |
|
||||||
between values. |
|
||||||
""" |
|
||||||
newlen = (n+1)*x.size |
|
||||||
out = np.zeros(newlen) |
|
||||||
indices = list(range(0, newlen-n, n+1)) |
|
||||||
out[indices] = x |
|
||||||
return out |
|
||||||
|
|
||||||
|
|
||||||
def find_steps(array, threshold): |
|
||||||
""" |
|
||||||
Finds local maxima by segmenting array based on positions at which |
|
||||||
the threshold value is crossed. Note that this thresholding is |
|
||||||
applied after the absolute value of the array is taken. Thus, |
|
||||||
the distinction between upward and downward steps is lost. However, |
|
||||||
get_step_sizes can be used to determine directionality after the |
|
||||||
fact. |
|
||||||
Parameters |
|
||||||
---------- |
|
||||||
array : numpy array |
|
||||||
1 dimensional array that represents time series of data points |
|
||||||
threshold : int / float |
|
||||||
Threshold value that defines a step |
|
||||||
Returns |
|
||||||
------- |
|
||||||
steps : list |
|
||||||
List of indices of the detected steps |
|
||||||
""" |
|
||||||
steps = [] |
|
||||||
array = np.abs(array) |
|
||||||
above_points = np.where(array > threshold, 1, 0) |
|
||||||
ap_dif = np.diff(above_points) |
|
||||||
cross_ups = np.where(ap_dif == 1)[0] |
|
||||||
cross_dns = np.where(ap_dif == -1)[0] |
|
||||||
for upi, dni in zip(cross_ups,cross_dns): |
|
||||||
steps.append(np.argmax(array[upi:dni]) + upi) |
|
||||||
return steps |
|
||||||
|
|
||||||
|
|
||||||
def get_step_sizes(array, indices, window=1000): |
|
||||||
""" |
|
||||||
Calculates step size for each index within the supplied list. Step |
|
||||||
size is determined by averaging over a range of points (specified |
|
||||||
by the window parameter) before and after the index of step |
|
||||||
occurrence. The directionality of the step is reflected by the sign |
|
||||||
of the step size (i.e. a positive value indicates an upward step, |
|
||||||
and a negative value indicates a downward step). The combined |
|
||||||
standard deviation of both measurements (as a measure of uncertainty |
|
||||||
in step calculation) is also provided. |
|
||||||
Parameters |
|
||||||
---------- |
|
||||||
array : numpy array |
|
||||||
1 dimensional array that represents time series of data points |
|
||||||
indices : list |
|
||||||
List of indices of the detected steps (as provided by |
|
||||||
find_steps, for example) |
|
||||||
window : int, optional |
|
||||||
Number of points to average over to determine baseline levels |
|
||||||
before and after step. |
|
||||||
Returns |
|
||||||
------- |
|
||||||
step_sizes : list |
|
||||||
List of the calculated sizes of each step |
|
||||||
step_error : list |
|
||||||
""" |
|
||||||
step_sizes = [] |
|
||||||
step_error = [] |
|
||||||
indices = sorted(indices) |
|
||||||
last = len(indices) - 1 |
|
||||||
for i, index in enumerate(indices): |
|
||||||
if i == 0: |
|
||||||
q = min(window, indices[i+1]-index) |
|
||||||
elif i == last: |
|
||||||
q = min(window, index - indices[i-1]) |
|
||||||
else: |
|
||||||
q = min(window, index-indices[i-1], indices[i+1]-index) |
|
||||||
a = array[index:index+q] |
|
||||||
b = array[index-q:index] |
|
||||||
step_sizes.append(a.mean() - b.mean()) |
|
||||||
step_error.append(sqrt(a.var()+b.var())) |
|
||||||
return step_sizes, step_error |
|
Loading…
Reference in new issue