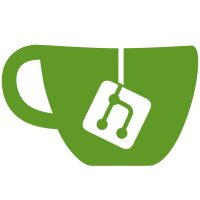
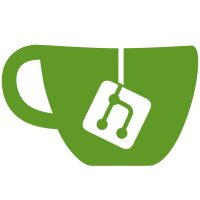
4 changed files with 45 additions and 22 deletions
@ -1,14 +1,33 @@ |
|||||||
|
from typing import Optional |
||||||
|
|
||||||
import pandas as pd |
import pandas as pd |
||||||
|
|
||||||
|
|
||||||
class DataBucket: |
class DataBucket: |
||||||
|
|
||||||
def __init__(self): |
def __init__(self, max_size: Optional[int] = None): |
||||||
|
self.set_max_size(max_size) |
||||||
self.data = pd.DataFrame([], columns=['timestamp', 'value']) |
self.data = pd.DataFrame([], columns=['timestamp', 'value']) |
||||||
|
|
||||||
def receive_data(self, data: pd.DataFrame): |
def append_data(self, data: pd.DataFrame) -> None: |
||||||
self.data = self.data.append(data, ignore_index=True) |
self.data = self.data.append(data, ignore_index=True) |
||||||
|
|
||||||
def drop_data(self, count: int): |
if self.max_size == None: |
||||||
|
return |
||||||
|
|
||||||
|
bucket_size = self.get_current_size() |
||||||
|
if bucket_size > self.max_size: |
||||||
|
extra_data_count = bucket_size - self.max_size |
||||||
|
self.drop_data(extra_data_count) |
||||||
|
|
||||||
|
def drop_data(self, count: int) -> None: |
||||||
if count > 0: |
if count > 0: |
||||||
self.data = self.data.iloc[count:] |
self.data = self.data.iloc[count:] |
||||||
|
|
||||||
|
def set_max_size(self, max_size: Optional[int] = None) -> None: |
||||||
|
if max_size is not None and max_size < 0: |
||||||
|
raise Exception(f'Can`t set negative max size for bucket: {max_size}') |
||||||
|
self.max_size = max_size |
||||||
|
|
||||||
|
def get_current_size(self) -> int: |
||||||
|
return len(self.data) |
||||||
|
Loading…
Reference in new issue