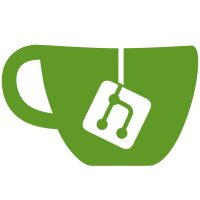
37 changed files with 8040 additions and 0 deletions
@ -0,0 +1,29 @@
|
||||
# Hastic server |
||||
|
||||
Implementation of basic pattern recognition and unsupervised learning for anomamaly detection. |
||||
|
||||
Implementation of analytic unit for Hastic. |
||||
see [REST API](REST.md) |
||||
|
||||
## Build & run |
||||
|
||||
### Analytic unit |
||||
|
||||
Python3 project |
||||
|
||||
``` |
||||
pip3 install pandas |
||||
pip3 install influxdb |
||||
|
||||
``` |
||||
|
||||
### Server |
||||
|
||||
Node.js project |
||||
|
||||
``` |
||||
cd server |
||||
npm install |
||||
npm run build |
||||
npm start |
||||
``` |
@ -0,0 +1,198 @@
|
||||
# Hastic server REST API |
||||
|
||||
## /anomalies |
||||
|
||||
### Get anomalies |
||||
`GET /anomalies?id=<anomaly_id>[&name=<anomaly_name>]` |
||||
|
||||
NOTE: `name` param is deprecated, use `id` instead |
||||
|
||||
Return data format: |
||||
|
||||
``` |
||||
{ |
||||
"name": "<anomaly_name>", |
||||
"metric": "<metric_id>", |
||||
"status": "<str>" |
||||
} |
||||
``` |
||||
|
||||
status field can be one of: |
||||
|
||||
- `learning` |
||||
- `ready` |
||||
- `failed` |
||||
|
||||
### Get anomaly status |
||||
`GET /anomalies/status?id=<anomaly_id>[&name=<anomaly_name>]` |
||||
|
||||
NOTE: `name` param is deprecated, use `id` instead |
||||
|
||||
Return data format: |
||||
|
||||
``` |
||||
{ |
||||
"status": <str> |
||||
} |
||||
``` |
||||
|
||||
status field can be one of: |
||||
|
||||
- `learning` |
||||
- `ready` |
||||
- `failed` |
||||
|
||||
### Add anomaly |
||||
|
||||
`POST /anomalies` |
||||
|
||||
Data format: |
||||
|
||||
``` |
||||
{ |
||||
"name": "cpu_utilization_supervised", |
||||
"metric": { |
||||
"datasource": "influx accelerometer", |
||||
"targets": [ |
||||
<targets> |
||||
] |
||||
}, |
||||
"panelUrl": "http://grafana.example.com/d/oNZ35bWiz/new-dashboard-copy?panelId=2&fullscreen" |
||||
} |
||||
``` |
||||
|
||||
`targets` example: |
||||
|
||||
``` |
||||
{ |
||||
"alias": "command", |
||||
"groupBy": [], |
||||
"measurement": "data", |
||||
"orderByTime": "ASC", |
||||
"policy": "default", |
||||
"refId": "A", |
||||
"resultFormat": "time_series", |
||||
"select": [ |
||||
[ |
||||
{ |
||||
"params": [ |
||||
"command" |
||||
], |
||||
"type": "field" |
||||
} |
||||
] |
||||
], |
||||
"tags": [] |
||||
} |
||||
``` |
||||
|
||||
Return data format: |
||||
|
||||
``` |
||||
{ |
||||
"anomaly_id": "<anomaly_id>" |
||||
} |
||||
``` |
||||
|
||||
### Delete anpmalies |
||||
`DELETE /anomalies` |
||||
|
||||
Data format: |
||||
|
||||
``` |
||||
{ |
||||
"id": "<anomaly_id>", |
||||
"name": "<anomaly_name>" // deprecated, use id instead |
||||
} |
||||
``` |
||||
|
||||
Return data format: |
||||
|
||||
``` |
||||
Success |
||||
``` |
||||
|
||||
## /segments |
||||
|
||||
### Get segments |
||||
`GET /segments?anomaly_id=<anomaly_id>[&last_segment=<id>][&from=<time_from>][&to=<time_to>]` |
||||
|
||||
Return data format: |
||||
|
||||
``` |
||||
{ |
||||
"segments": [ |
||||
{ |
||||
"id": 0, |
||||
"start": 1392765184318, |
||||
"finish": 1397243699000, |
||||
"labeled": true |
||||
}, |
||||
... |
||||
] |
||||
} |
||||
``` |
||||
|
||||
### Update segments |
||||
|
||||
`PATCH /segments` |
||||
|
||||
Data format: |
||||
|
||||
``` |
||||
{ |
||||
"anomaly_id": "<anomaly_id>", |
||||
"name": "<anomaly_name>", // deprecated, use id instead |
||||
"added_segments": [ |
||||
{ |
||||
"start": 1397164656000, |
||||
"finish": 1397243699000 |
||||
}, |
||||
... |
||||
], |
||||
"removed_segments": [3, 9] |
||||
} |
||||
``` |
||||
|
||||
Return data format: |
||||
|
||||
``` |
||||
{ |
||||
"added_ids": [12, ...] |
||||
} |
||||
``` |
||||
|
||||
## /alerts |
||||
|
||||
### Check if alert is enabled for anomaly |
||||
|
||||
`GET /alerts?anomaly_id=<anomaly_id>` |
||||
|
||||
Return data format: |
||||
|
||||
``` |
||||
{ |
||||
"enable": true |
||||
} |
||||
``` |
||||
|
||||
### Enable / disable alert for anomaly |
||||
|
||||
`POST /alerts` |
||||
|
||||
Data format: |
||||
|
||||
``` |
||||
{ |
||||
"anomaly_id": "<anomaly_id>", |
||||
"enable": true |
||||
} |
||||
``` |
||||
|
||||
Return data format: |
||||
|
||||
``` |
||||
{ |
||||
"status": "Ok" |
||||
} |
||||
``` |
@ -0,0 +1,27 @@
|
||||
# Hastic server |
||||
|
||||
REST server for managing data for analytics. |
||||
|
||||
Running on 8000 port. |
||||
|
||||
# Build |
||||
|
||||
``` |
||||
npm install |
||||
npm run build |
||||
``` |
||||
|
||||
# Run |
||||
|
||||
``` |
||||
npm start |
||||
``` |
||||
|
||||
# Development |
||||
|
||||
You should have `nodemon` module installed to run development server. |
||||
|
||||
``` |
||||
npm i -g nodemon |
||||
npm run dev |
||||
``` |
@ -0,0 +1,10 @@
|
||||
const { spawn } = require('child_process'); |
||||
|
||||
const webpack = spawn('webpack', ['--config', 'build/webpack.dev.conf.js'], { |
||||
stdio: 'inherit', |
||||
shell: true |
||||
}); |
||||
//webpack.stdout.pipe(process.stdout);
|
||||
|
||||
const nodemon = spawn('nodemon', ['../dist/server', '--watch', 'server.js']); |
||||
nodemon.stdout.pipe(process.stdout); |
@ -0,0 +1,52 @@
|
||||
const path = require('path'); |
||||
const fs = require('fs'); |
||||
|
||||
const webpack = require('webpack'); |
||||
|
||||
|
||||
function resolve(p) { |
||||
return path.join(__dirname, '/../', p); |
||||
} |
||||
|
||||
module.exports = { |
||||
target: 'node', |
||||
node: { |
||||
__dirname: false, |
||||
__filename: false, |
||||
}, |
||||
context: resolve('./src'), |
||||
entry: './index', |
||||
devtool: 'inline-source-map', |
||||
output: { |
||||
filename: "server.js", |
||||
path: resolve('dist') |
||||
}, |
||||
externals: [ |
||||
function(context, request, callback) { |
||||
if(request[0] == '.') { |
||||
callback(); |
||||
} else { |
||||
callback(null, "require('" + request + "')"); |
||||
} |
||||
} |
||||
], |
||||
plugins: [ |
||||
new webpack.optimize.OccurrenceOrderPlugin(), |
||||
new webpack.HotModuleReplacementPlugin(), |
||||
new webpack.DefinePlugin({ |
||||
'process.env.NODE_ENV': JSON.stringify('development') |
||||
}) |
||||
], |
||||
resolve: { |
||||
extensions: [".ts", ".js"] |
||||
}, |
||||
module: { |
||||
rules: [ |
||||
{ |
||||
test: /\.ts$/, |
||||
loader: "ts-loader", |
||||
exclude: /node_modules/ |
||||
} |
||||
] |
||||
} |
||||
} |
@ -0,0 +1,4 @@
|
||||
var base = require('./webpack.base.conf'); |
||||
|
||||
base.watch = true; |
||||
module.exports = base; |
@ -0,0 +1,3 @@
|
||||
var base = require('./webpack.base.conf'); |
||||
|
||||
module.exports = base; |
File diff suppressed because it is too large
Load Diff
@ -0,0 +1,32 @@
|
||||
{ |
||||
"name": "hastic-server", |
||||
"version": "1.0.0", |
||||
"description": "REST server for managing data for analytics", |
||||
"scripts": { |
||||
"start": "node dist/server.js", |
||||
"dev": "node build/dev-server.js", |
||||
"build": "webpack --config build/webpack.prod.conf.js" |
||||
}, |
||||
"repository": { |
||||
"type": "git", |
||||
"url": "git+https://github.com/hastic/hastic-server.git" |
||||
}, |
||||
"author": "CorpGlory", |
||||
"license": "ISC", |
||||
"bugs": { |
||||
"url": "https://github.com/hastic/hastic-server/issues" |
||||
}, |
||||
"homepage": "https://github.com/hastic/hastic-server#readme", |
||||
"dependencies": { |
||||
"express": "^4.16.3", |
||||
"fast-csv": "^2.4.1", |
||||
"telegraf": "^3.21.0" |
||||
}, |
||||
"devDependencies": { |
||||
"@types/express": "^4.11.1", |
||||
"nodemon": "^1.17.3", |
||||
"ts-loader": "^3.5.0", |
||||
"typescript": "^2.8.3", |
||||
"webpack": "^3.5.6" |
||||
} |
||||
} |
@ -0,0 +1,9 @@
|
||||
import * as path from 'path'; |
||||
|
||||
const DATA_PATH = path.join(__dirname, '../data'); |
||||
const ANALYTICS_PATH = path.join(__dirname, '../../src'); |
||||
const ANOMALIES_PATH = path.join(ANALYTICS_PATH, 'anomalies'); |
||||
const SEGMENTS_PATH = path.join(ANALYTICS_PATH, 'segments'); |
||||
const METRICS_PATH = path.join(ANALYTICS_PATH, 'metrics'); |
||||
|
||||
export { DATA_PATH, ANALYTICS_PATH, ANOMALIES_PATH, SEGMENTS_PATH, METRICS_PATH } |
@ -0,0 +1,31 @@
|
||||
import * as express from 'express'; |
||||
import * as bodyParser from 'body-parser'; |
||||
|
||||
import { router as anomaliesRouter } from './routes/anomalies'; |
||||
import { router as segmentsRouter } from './routes/segments'; |
||||
import { router as alertsRouter } from './routes/alerts'; |
||||
import { tgBotInit } from './services/notification'; |
||||
|
||||
const app = express(); |
||||
const PORT = 8000; |
||||
|
||||
app.use(bodyParser.json()); |
||||
app.use(bodyParser.urlencoded({ extended: true })); |
||||
|
||||
app.use(function (req, res, next) { |
||||
res.header('Access-Control-Allow-Origin', '*'); |
||||
res.header('Access-Control-Allow-Methods', 'GET, POST, PUT, DELETE, PATCH, OPTIONS'); |
||||
res.header('Access-Control-Allow-Headers', 'Origin, X-Requested-With, Content-Type, Accept'); |
||||
next(); |
||||
}); |
||||
|
||||
app.use('/anomalies', anomaliesRouter); |
||||
app.use('/segments', segmentsRouter); |
||||
app.use('/alerts', alertsRouter); |
||||
app.use('/', (req, res) => { res.send('Analytic unit works') }); |
||||
|
||||
app.listen(PORT, () => { |
||||
console.log(`Server is running on :${PORT}`) |
||||
}); |
||||
|
||||
tgBotInit(); |
@ -0,0 +1,62 @@
|
||||
import * as express from 'express'; |
||||
import {AnomalyId, getAnomalyIdByName, loadAnomalyById} from '../services/anomalyType'; |
||||
import { getAlertsAnomalies, saveAlertsAnomalies } from '../services/alerts'; |
||||
|
||||
function getAlert(req, res) { |
||||
try { |
||||
let anomalyId: AnomalyId = req.query.anomaly_id; |
||||
let anomaly = loadAnomalyById(anomalyId) |
||||
if (anomaly == null) { |
||||
anomalyId = getAnomalyIdByName(anomalyId.toLowerCase()); |
||||
} |
||||
|
||||
let alertsAnomalies = getAlertsAnomalies(); |
||||
let pos = alertsAnomalies.indexOf(anomalyId); |
||||
|
||||
let enable: boolean = (pos !== -1); |
||||
res.status(200).send({ |
||||
enable |
||||
}); |
||||
} catch(e) { |
||||
res.status(500).send({ |
||||
code: 500, |
||||
message: 'Internal error' |
||||
}); |
||||
} |
||||
} |
||||
|
||||
function changeAlert(req, res) { |
||||
try { |
||||
let anomalyId: AnomalyId = req.body.anomaly_id; |
||||
let enable: boolean = req.body.enable; |
||||
|
||||
let anomaly = loadAnomalyById(anomalyId) |
||||
if (anomaly == null) { |
||||
anomalyId = getAnomalyIdByName(anomalyId.toLowerCase()); |
||||
} |
||||
|
||||
let alertsAnomalies = getAlertsAnomalies(); |
||||
let pos: number = alertsAnomalies.indexOf(anomalyId); |
||||
if(enable && pos == -1) { |
||||
alertsAnomalies.push(anomalyId); |
||||
saveAlertsAnomalies(alertsAnomalies); |
||||
} else if(!enable && pos > -1) { |
||||
alertsAnomalies.splice(pos, 1); |
||||
saveAlertsAnomalies(alertsAnomalies); |
||||
} |
||||
res.status(200).send({ |
||||
status: 'Ok' |
||||
}); |
||||
} catch(e) { |
||||
res.status(500).send({ |
||||
code: 500, |
||||
message: 'Internal error' |
||||
}); |
||||
} |
||||
} |
||||
|
||||
export const router = express.Router(); |
||||
|
||||
router.get('/', getAlert); |
||||
router.post('/', changeAlert); |
||||
|
@ -0,0 +1,136 @@
|
||||
import * as express from 'express'; |
||||
|
||||
import { |
||||
Metric, |
||||
Anomaly, |
||||
saveAnomaly, |
||||
insertAnomaly, removeAnomaly, loadAnomalyByName, loadAnomalyById, getAnomalyIdByName |
||||
} from '../services/anomalyType'; |
||||
import { runLearning } from '../services/analytics' |
||||
import { saveTargets } from '../services/metrics'; |
||||
|
||||
async function sendAnomalyTypeStatus(req, res) { |
||||
let id = req.query.id; |
||||
let name = req.query.name; |
||||
try { |
||||
let anomaly: Anomaly; |
||||
if(id !== undefined) { |
||||
anomaly = loadAnomalyById(id); |
||||
} else { |
||||
anomaly = loadAnomalyByName(name); |
||||
} |
||||
if(anomaly === null) { |
||||
res.status(404).send({ |
||||
code: 404, |
||||
message: 'Not found' |
||||
}); |
||||
return; |
||||
} |
||||
if(anomaly.status === undefined) { |
||||
throw new Error('No status for ' + name); |
||||
} |
||||
res.status(200).send({ status: anomaly.status }); |
||||
} catch(e) { |
||||
console.error(e); |
||||
// TODO: better send 404 when we know than isn`t found
|
||||
res.status(500).send({ error: 'Can`t return anything' }); |
||||
} |
||||
|
||||
} |
||||
|
||||
async function getAnomaly(req, res) { |
||||
try { |
||||
let id = req.query.id; |
||||
let name = req.query.name; |
||||
|
||||
let anomaly:Anomaly; |
||||
if(id !== undefined) { |
||||
anomaly = loadAnomalyById(id); |
||||
} else { |
||||
anomaly = loadAnomalyByName(name.toLowerCase()); |
||||
} |
||||
if(anomaly === null) { |
||||
res.status(404).send({ |
||||
code: 404, |
||||
message: 'Not found' |
||||
}); |
||||
return; |
||||
} |
||||
|
||||
let payload = JSON.stringify({ |
||||
name: anomaly.name, |
||||
metric: anomaly.metric, |
||||
status: anomaly.status |
||||
}); |
||||
res.status(200).send(payload) |
||||
} catch(e) { |
||||
console.error(e); |
||||
// TODO: better send 404 when we know than isn`t found
|
||||
res.status(500).send('Can`t get anything'); |
||||
} |
||||
} |
||||
|
||||
async function createAnomaly(req, res) { |
||||
try { |
||||
const metric:Metric = { |
||||
datasource: req.body.metric.datasource, |
||||
targets: saveTargets(req.body.metric.targets) |
||||
}; |
||||
|
||||
const anomaly:Anomaly = { |
||||
name: req.body.name, |
||||
panelUrl: req.body.panelUrl, |
||||
metric: metric, |
||||
status: 'learning', |
||||
last_prediction_time: 0, |
||||
next_id: 0 |
||||
}; |
||||
let anomalyId = insertAnomaly(anomaly); |
||||
if(anomalyId === null) { |
||||
res.status(403).send({ |
||||
code: 403, |
||||
message: 'Already exists' |
||||
}); |
||||
} |
||||
|
||||
let payload = JSON.stringify({ anomaly_id: anomalyId }) |
||||
res.status(200).send(payload); |
||||
|
||||
runLearning(anomalyId); |
||||
} catch(e) { |
||||
res.status(500).send({ |
||||
code: 500, |
||||
message: 'Internal error' |
||||
}); |
||||
} |
||||
} |
||||
|
||||
function deleteAnomaly(req, res) { |
||||
try { |
||||
let id = req.query.id; |
||||
let name = req.query.name; |
||||
|
||||
if(id !== undefined) { |
||||
removeAnomaly(id); |
||||
} else { |
||||
removeAnomaly(name.toLowerCase()); |
||||
} |
||||
|
||||
res.status(200).send({ |
||||
code: 200, |
||||
message: 'Success' |
||||
}); |
||||
} catch(e) { |
||||
res.status(500).send({ |
||||
code: 500, |
||||
message: 'Internal error' |
||||
}); |
||||
} |
||||
} |
||||
|
||||
export const router = express.Router(); |
||||
|
||||
router.get('/status', sendAnomalyTypeStatus); |
||||
router.get('/', getAnomaly); |
||||
router.post('/', createAnomaly); |
||||
router.delete('/', deleteAnomaly); |
@ -0,0 +1,80 @@
|
||||
import * as express from 'express'; |
||||
import { |
||||
getLabeledSegments, |
||||
insertSegments, |
||||
removeSegments, |
||||
} from '../services/segments'; |
||||
import {runLearning} from '../services/analytics'; |
||||
import {Anomaly, AnomalyId, getAnomalyIdByName, loadAnomalyById} from '../services/anomalyType'; |
||||
|
||||
|
||||
async function sendSegments(req, res) { |
||||
try { |
||||
let anomalyId: AnomalyId = req.query.anomaly_id; |
||||
let anomaly:Anomaly = loadAnomalyById(anomalyId); |
||||
if(anomaly === null) { |
||||
anomalyId = getAnomalyIdByName(anomalyId); |
||||
} |
||||
|
||||
let lastSegmentId = req.query.last_segment; |
||||
let timeFrom = req.query.from; |
||||
let timeTo = req.query.to; |
||||
|
||||
let segments = getLabeledSegments(anomalyId); |
||||
|
||||
// Id filtering
|
||||
if(lastSegmentId !== undefined) { |
||||
segments = segments.filter(el => el.id > lastSegmentId); |
||||
} |
||||
|
||||
// Time filtering
|
||||
if(timeFrom !== undefined) { |
||||
segments = segments.filter(el => el.finish > timeFrom); |
||||
} |
||||
|
||||
if(timeTo !== undefined) { |
||||
segments = segments.filter(el => el.start < timeTo); |
||||
} |
||||
|
||||
let payload = JSON.stringify({ |
||||
segments |
||||
}); |
||||
res.status(200).send(payload); |
||||
} catch(e) { |
||||
res.status(500).send({ |
||||
code: 500, |
||||
message: 'Internal error' |
||||
}); |
||||
} |
||||
} |
||||
|
||||
async function updateSegments(req, res) { |
||||
try { |
||||
let segmentsUpdate = req.body; |
||||
|
||||
let anomalyId = segmentsUpdate.anomaly_id; |
||||
let anomalyName = segmentsUpdate.name; |
||||
|
||||
if(anomalyId === undefined) { |
||||
anomalyId = getAnomalyIdByName(anomalyName.toLowerCase()); |
||||
} |
||||
|
||||
let addedIds = insertSegments(anomalyId, segmentsUpdate.added_segments, true); |
||||
removeSegments(anomalyId, segmentsUpdate.removed_segments); |
||||
|
||||
let payload = JSON.stringify({ added_ids: addedIds }); |
||||
res.status(200).send(payload); |
||||
|
||||
runLearning(anomalyId); |
||||
} catch(e) { |
||||
res.status(500).send({ |
||||
code: 500, |
||||
message: 'Internal error' |
||||
}); |
||||
} |
||||
} |
||||
|
||||
export const router = express.Router(); |
||||
|
||||
router.get('/', sendSegments); |
||||
router.patch('/', updateSegments); |
@ -0,0 +1,58 @@
|
||||
import { getJsonDataSync, writeJsonDataSync } from './json'; |
||||
import * as path from 'path'; |
||||
import { AnomalyId } from './anomalyType'; |
||||
import { ANOMALIES_PATH } from '../config'; |
||||
import { runPredict } from './analytics'; |
||||
import { sendNotification } from './notification'; |
||||
import { getLabeledSegments } from './segments'; |
||||
|
||||
function getAlertsAnomalies() : AnomalyId[] { |
||||
return getJsonDataSync(path.join(ANOMALIES_PATH, `alerts_anomalies.json`)); |
||||
} |
||||
|
||||
function saveAlertsAnomalies(anomalies: AnomalyId[]) { |
||||
return writeJsonDataSync(path.join(ANOMALIES_PATH, `alerts_anomalies.json`), anomalies); |
||||
} |
||||
|
||||
function processAlerts(anomalyId) { |
||||
let segments = getLabeledSegments(anomalyId); |
||||
|
||||
const currentTime = new Date().getTime(); |
||||
const activeAlert = activeAlerts.has(anomalyId); |
||||
let newActiveAlert = false; |
||||
|
||||
if(segments.length > 0) { |
||||
let lastSegment = segments[segments.length - 1]; |
||||
if(lastSegment.finish >= currentTime - alertTimeout) { |
||||
newActiveAlert = true; |
||||
} |
||||
} |
||||
|
||||
if(!activeAlert && newActiveAlert) { |
||||
activeAlerts.add(anomalyId); |
||||
sendNotification(anomalyId, true); |
||||
} else if(activeAlert && !newActiveAlert) { |
||||
activeAlerts.delete(anomalyId); |
||||
sendNotification(anomalyId, false); |
||||
} |
||||
} |
||||
|
||||
async function alertsTick() { |
||||
let alertsAnomalies = getAlertsAnomalies(); |
||||
for (let anomalyId of alertsAnomalies) { |
||||
try { |
||||
await runPredict(anomalyId); |
||||
processAlerts(anomalyId); |
||||
} catch (e) { |
||||
console.error(e); |
||||
} |
||||
} |
||||
setTimeout(alertsTick, 5000); |
||||
} |
||||
|
||||
const alertTimeout = 60000; // ms
|
||||
const activeAlerts = new Set<string>(); |
||||
setTimeout(alertsTick, 5000); |
||||
|
||||
|
||||
export { getAlertsAnomalies, saveAlertsAnomalies } |
@ -0,0 +1,141 @@
|
||||
import { spawn } from 'child_process' |
||||
import { ANALYTICS_PATH } from '../config' |
||||
import { |
||||
Anomaly, |
||||
AnomalyId, getAnomalyTypeInfo, |
||||
loadAnomalyById, |
||||
setAnomalyPredictionTime, |
||||
setAnomalyStatus |
||||
} from './anomalyType' |
||||
import { getTarget } from './metrics'; |
||||
import { getLabeledSegments, insertSegments, removeSegments } from './segments'; |
||||
import { split, map, mapSync } from 'event-stream' |
||||
|
||||
const learnWorker = spawn('python3', ['worker.py'], { cwd: ANALYTICS_PATH }) |
||||
learnWorker.stdout.pipe(split()) |
||||
.pipe( |
||||
mapSync(function(line){ |
||||
console.log(line) |
||||
onMessage(line) |
||||
}) |
||||
); |
||||
|
||||
learnWorker.stderr.on('data', data => console.error(`worker stderr: ${data}`)); |
||||
|
||||
const taskMap = {}; |
||||
let nextTaskId = 0; |
||||
|
||||
function onMessage(data) { |
||||
let response = JSON.parse(data); |
||||
let taskId = response.__task_id; |
||||
// let anomalyName = response.anomaly_name;
|
||||
// let task = response.task;
|
||||
let status = response.status; |
||||
|
||||
if(status === 'success' || status === 'failed') { |
||||
if(taskId in taskMap) { |
||||
let resolver = taskMap[taskId]; |
||||
resolver(response); |
||||
delete taskMap[taskId]; |
||||
} |
||||
} |
||||
} |
||||
|
||||
function runTask(task) : Promise<any> { |
||||
let anomaly:Anomaly = loadAnomalyById(task.anomaly_id); |
||||
task.metric = { |
||||
datasource: anomaly.metric.datasource, |
||||
targets: anomaly.metric.targets.map(t => getTarget(t)) |
||||
}; |
||||
|
||||
task.__task_id = nextTaskId++; |
||||
let command = JSON.stringify(task) |
||||
learnWorker.stdin.write(`${command}\n`); |
||||
return new Promise<Object>((resolve, reject) => { |
||||
taskMap[task.__task_id] = resolve |
||||
}) |
||||
} |
||||
|
||||
async function runLearning(anomalyId:AnomalyId) { |
||||
let segments = getLabeledSegments(anomalyId); |
||||
setAnomalyStatus(anomalyId, 'learning'); |
||||
let anomaly:Anomaly = loadAnomalyById(anomalyId); |
||||
let analyticsType = "anomalies"; |
||||
let preset = undefined; |
||||
if (anomaly.name.includes("jumps")) { |
||||
analyticsType = "patterns"; |
||||
preset = "steps" |
||||
} |
||||
if (anomaly.name.includes("cliffs") || anomaly.name.includes("drops")) { |
||||
analyticsType = "patterns"; |
||||
preset = "cliffs" |
||||
} |
||||
if (anomaly.name.includes("peaks")) { |
||||
analyticsType = "patterns"; |
||||
preset = "peaks" |
||||
} |
||||
let task = { |
||||
type: 'learn', |
||||
anomaly_id: anomalyId, |
||||
analytics_type: analyticsType, |
||||
preset, |
||||
segments: segments |
||||
}; |
||||
|
||||
let result = await runTask(task); |
||||
|
||||
if (result.status === 'success') { |
||||
setAnomalyStatus(anomalyId, 'ready'); |
||||
insertSegments(anomalyId, result.segments, false); |
||||
setAnomalyPredictionTime(anomalyId, result.last_prediction_time); |
||||
} else { |
||||
setAnomalyStatus(anomalyId, 'failed'); |
||||
} |
||||
} |
||||
|
||||
async function runPredict(anomalyId:AnomalyId) { |
||||
let anomaly:Anomaly = loadAnomalyById(anomalyId); |
||||
let analyticsType = "anomalies"; |
||||
let preset = undefined; |
||||
if (anomaly.name.includes("jump")) { |
||||
analyticsType = "patterns"; |
||||
preset = "steps" |
||||
} |
||||
if (anomaly.name.includes("cliffs") || anomaly.name.includes("drops")) { |
||||
analyticsType = "patterns"; |
||||
preset = "cliffs" |
||||
} |
||||
if (anomaly.name.includes("peaks")) { |
||||
analyticsType = "patterns"; |
||||
preset = "peaks" |
||||
} |
||||
let task = { |
||||
type: 'predict', |
||||
anomaly_id: anomalyId, |
||||
analytics_type: analyticsType, |
||||
preset, |
||||
last_prediction_time: anomaly.last_prediction_time |
||||
}; |
||||
let result = await runTask(task); |
||||
|
||||
if(result.status === 'failed') { |
||||
return []; |
||||
} |
||||
// Merging segments
|
||||
let segments = getLabeledSegments(anomalyId); |
||||
if(segments.length > 0 && result.segments.length > 0) { |
||||
let lastOldSegment = segments[segments.length - 1]; |
||||
let firstNewSegment = result.segments[0]; |
||||
|
||||
if(firstNewSegment.start <= lastOldSegment.finish) { |
||||
result.segments[0].start = lastOldSegment.start; |
||||
removeSegments(anomalyId, [lastOldSegment.id]); |
||||
} |
||||
} |
||||
|
||||
insertSegments(anomalyId, result.segments, false); |
||||
setAnomalyPredictionTime(anomalyId, result.last_prediction_time); |
||||
return result.segments; |
||||
} |
||||
|
||||
export { runLearning, runPredict } |
@ -0,0 +1,117 @@
|
||||
import * as path from 'path' |
||||
import { getJsonDataSync, writeJsonDataSync } from './json' |
||||
import { ANOMALIES_PATH } from '../config' |
||||
import * as fs from 'fs' |
||||
import * as crypto from 'crypto'; |
||||
|
||||
export type Metric = { |
||||
datasource: string, |
||||
targets: string[] |
||||
} |
||||
|
||||
export type Anomaly = { |
||||
name: string, |
||||
|
||||
panelUrl: string, |
||||
|
||||
metric: Metric, |
||||
status: string, |
||||
|
||||
last_prediction_time: number, |
||||
next_id: number |
||||
} |
||||
|
||||
export type AnomalyId = string; |
||||
|
||||
let anomaliesNameToIdMap = {}; |
||||
|
||||
function loadAnomaliesMap() { |
||||
let filename = path.join(ANOMALIES_PATH, `all_anomalies.json`); |
||||
anomaliesNameToIdMap = getJsonDataSync(filename); |
||||
} |
||||
|
||||
function saveAnomaliesMap() { |
||||
let filename = path.join(ANOMALIES_PATH, `all_anomalies.json`); |
||||
writeJsonDataSync(filename, anomaliesNameToIdMap); |
||||
} |
||||
|
||||
function getAnomalyIdByName(anomalyName:string) : AnomalyId { |
||||
loadAnomaliesMap(); |
||||
anomalyName = anomalyName.toLowerCase(); |
||||
if(anomalyName in anomaliesNameToIdMap) { |
||||
return anomaliesNameToIdMap[anomalyName]; |
||||
} |
||||
return anomalyName; |
||||
} |
||||
|
||||
function insertAnomaly(anomaly: Anomaly) : AnomalyId { |
||||
const hashString = anomaly.name + (new Date()).toString(); |
||||
const anomalyId:AnomalyId = crypto.createHash('md5').update(hashString).digest('hex'); |
||||
anomaliesNameToIdMap[anomaly.name] = anomalyId; |
||||
saveAnomaliesMap(); |
||||
// return anomalyId
|
||||
// const anomalyId:AnomalyId = anomaly.name;
|
||||
let filename = path.join(ANOMALIES_PATH, `${anomalyId}.json`); |
||||
if(fs.existsSync(filename)) { |
||||
return null; |
||||
} |
||||
saveAnomaly(anomalyId, anomaly); |
||||
return anomalyId; |
||||
} |
||||
|
||||
function removeAnomaly(anomalyId:AnomalyId) { |
||||
let filename = path.join(ANOMALIES_PATH, `${anomalyId}.json`); |
||||
fs.unlinkSync(filename); |
||||
} |
||||
|
||||
function saveAnomaly(anomalyId: AnomalyId, anomaly: Anomaly) { |
||||
let filename = path.join(ANOMALIES_PATH, `${anomalyId}.json`); |
||||
return writeJsonDataSync(filename, anomaly); |
||||
} |
||||
|
||||
function loadAnomalyById(anomalyId: AnomalyId) : Anomaly { |
||||
let filename = path.join(ANOMALIES_PATH, `${anomalyId}.json`); |
||||
if(!fs.existsSync(filename)) { |
||||
return null; |
||||
} |
||||
return getJsonDataSync(filename); |
||||
} |
||||
|
||||
function loadAnomalyByName(anomalyName: string) : Anomaly { |
||||
let anomalyId = getAnomalyIdByName(anomalyName); |
||||
return loadAnomalyById(anomalyId); |
||||
} |
||||
|
||||
function saveAnomalyTypeInfo(info) { |
||||
console.log('Saving'); |
||||
let filename = path.join(ANOMALIES_PATH, `${info.name}.json`); |
||||
if(info.next_id === undefined) { |
||||
info.next_id = 0; |
||||
} |
||||
if(info.last_prediction_time === undefined) { |
||||
info.last_prediction_time = 0; |
||||
} |
||||
|
||||
return writeJsonDataSync(filename, info); |
||||
} |
||||
|
||||
function getAnomalyTypeInfo(name) { |
||||
return getJsonDataSync(path.join(ANOMALIES_PATH, `${name}.json`)); |
||||
} |
||||
|
||||
function setAnomalyStatus(anomalyId:AnomalyId, status:string) { |
||||
let info = loadAnomalyById(anomalyId); |
||||
info.status = status; |
||||
saveAnomaly(anomalyId, info); |
||||
} |
||||
|
||||
function setAnomalyPredictionTime(anomalyId:AnomalyId, lastPredictionTime:number) { |
||||
let info = loadAnomalyById(anomalyId); |
||||
info.last_prediction_time = lastPredictionTime; |
||||
saveAnomaly(anomalyId, info); |
||||
} |
||||
|
||||
export { |
||||
saveAnomaly, loadAnomalyById, loadAnomalyByName, insertAnomaly, removeAnomaly, saveAnomalyTypeInfo, |
||||
getAnomalyTypeInfo, getAnomalyIdByName, setAnomalyStatus, setAnomalyPredictionTime |
||||
} |
@ -0,0 +1,55 @@
|
||||
import * as fs from 'fs'; |
||||
|
||||
async function getJsonData(filename: string): Promise<Object> { |
||||
var data = await new Promise<string>((resolve, reject) => { |
||||
fs.readFile(filename, 'utf8', (err, data) => { |
||||
if(err) { |
||||
console.error(err); |
||||
reject('Can`t read file'); |
||||
} else { |
||||
resolve(data); |
||||
} |
||||
}); |
||||
}); |
||||
|
||||
try { |
||||
return JSON.parse(data); |
||||
} catch(e) { |
||||
console.error(e); |
||||
throw new Error('Wrong file format'); |
||||
} |
||||
} |
||||
|
||||
function writeJsonData(filename: string, data: Object) { |
||||
return new Promise((resolve, reject) => { |
||||
fs.writeFile(filename, JSON.stringify(data), 'utf8', (err) => { |
||||
if(err) { |
||||
console.error(err); |
||||
reject('Cat`t write file'); |
||||
} else { |
||||
resolve(); |
||||
} |
||||
}); |
||||
}) |
||||
} |
||||
|
||||
function getJsonDataSync(filename: string) { |
||||
let data = fs.readFileSync(filename, 'utf8'); |
||||
try { |
||||
return JSON.parse(data); |
||||
} catch(e) { |
||||
console.error(e); |
||||
throw new Error('Wrong file format'); |
||||
} |
||||
} |
||||
|
||||
function writeJsonDataSync(filename: string, data: Object) { |
||||
fs.writeFileSync(filename, JSON.stringify(data)); |
||||
} |
||||
|
||||
export { |
||||
getJsonData, |
||||
writeJsonData, |
||||
getJsonDataSync, |
||||
writeJsonDataSync |
||||
} |
@ -0,0 +1,27 @@
|
||||
import * as path from 'path'; |
||||
import { getJsonDataSync, writeJsonDataSync } from './json'; |
||||
import { METRICS_PATH } from '../config'; |
||||
import * as crypto from 'crypto'; |
||||
|
||||
function saveTargets(targets) { |
||||
let metrics = []; |
||||
for (let target of targets) { |
||||
metrics.push(saveTarget(target)); |
||||
} |
||||
return metrics; |
||||
} |
||||
|
||||
function saveTarget(target) { |
||||
//const md5 = crypto.createHash('md5')
|
||||
const targetId = crypto.createHash('md5').update(JSON.stringify(target)).digest('hex'); |
||||
let filename = path.join(METRICS_PATH, `${targetId}.json`); |
||||
writeJsonDataSync(filename, target); |
||||
return targetId; |
||||
} |
||||
|
||||
function getTarget(targetId) { |
||||
let filename = path.join(METRICS_PATH, `${targetId}.json`); |
||||
return getJsonDataSync(filename); |
||||
} |
||||
|
||||
export { saveTargets, getTarget } |
@ -0,0 +1,140 @@
|
||||
//import * as Telegraf from 'telegraf'
|
||||
import * as path from 'path'; |
||||
import { DATA_PATH } from '../config'; |
||||
import { getJsonDataSync, writeJsonDataSync } from './json'; |
||||
import { AnomalyId } from './anomalyType'; |
||||
|
||||
|
||||
type SubscriberId = string; |
||||
type SubscribersMap = Map< AnomalyId, SubscriberId[] >; |
||||
|
||||
type BotConfig = { |
||||
token: string, |
||||
subscriptions: SubscribersMap |
||||
}; |
||||
|
||||
function sendNotification(anomalyName, active) { |
||||
console.log('Notification ' + anomalyName); |
||||
if(anomalyName in botConfig.subscriptions) { |
||||
let notificationMessage; |
||||
if(active) { |
||||
notificationMessage = 'Alert! Anomaly type ' + anomalyName; |
||||
} else { |
||||
notificationMessage = 'Ok! Anomaly type ' + anomalyName; |
||||
} |
||||
|
||||
for (let SubscriberId of botConfig.subscriptions[anomalyName]) { |
||||
bot.telegram.sendMessage(SubscriberId, notificationMessage); |
||||
} |
||||
} |
||||
} |
||||
|
||||
function loadBotConfig() : BotConfig { |
||||
let filename = path.join(DATA_PATH, `bot_config.json`); |
||||
let jsonData; |
||||
try { |
||||
jsonData = getJsonDataSync(filename); |
||||
} catch(e) { |
||||
console.error(e.message); |
||||
jsonData = []; |
||||
} |
||||
return jsonData; |
||||
} |
||||
|
||||
function saveBotConfig(botConfig: BotConfig) { |
||||
let filename = path.join(DATA_PATH, `bot_config.json`); |
||||
try { |
||||
writeJsonDataSync(filename, botConfig); |
||||
} catch(e) { |
||||
console.error(e.message); |
||||
} |
||||
} |
||||
|
||||
const commandArgs = (ctx, next) => { |
||||
try { |
||||
if(ctx.updateType === 'message') { |
||||
const text = ctx.update.message.text; |
||||
if(text !== undefined && text.startsWith('/')) { |
||||
const match = text.match(/^\/([^\s]+)\s?(.+)?/); |
||||
let args = []; |
||||
let command; |
||||
if(match !== null) { |
||||
if(match[1]) { |
||||
command = match[1]; |
||||
} |
||||
if(match[2]) { |
||||
args = match[2].split(' '); |
||||
} |
||||
} |
||||
ctx.state.command = { |
||||
raw: text, |
||||
command, |
||||
args, |
||||
}; |
||||
} |
||||
} |
||||
return next(ctx); |
||||
} catch (e) { |
||||
|
||||
} |
||||
}; |
||||
|
||||
function addNotification(ctx) { |
||||
console.log('addNotification') |
||||
let command = ctx.state.command; |
||||
let chatId = ctx.chat.id; |
||||
if(command.args.length > 0) { |
||||
for (let anomalyName of command.args) { |
||||
if(!(anomalyName in botConfig.subscriptions)) { |
||||
botConfig.subscriptions[anomalyName] = [] |
||||
} |
||||
if(botConfig.subscriptions[anomalyName].includes(chatId)) { |
||||
return ctx.reply('You are already subscribed on alerts from anomaly ' + command.args) |
||||
} else { |
||||
botConfig.subscriptions[anomalyName].push(chatId); |
||||
saveBotConfig(botConfig); |
||||
} |
||||
} |
||||
return ctx.reply('You have been successfully subscribed on alerts from anomaly ' + command.args) |
||||
} else { |
||||
return ctx.reply('You should use syntax: \/addNotification <anomaly_name>') |
||||
} |
||||
} |
||||
|
||||
function removeNotification(ctx) { |
||||
let command = ctx.state.command; |
||||
let chatId = ctx.chat.id; |
||||
if(command.args.length > 0) { |
||||
for (let anomalyName of command.args) { |
||||
if(anomalyName in botConfig.subscriptions) { |
||||
botConfig.subscriptions[anomalyName] = botConfig.subscriptions[anomalyName].filter(el => el !== chatId); |
||||
saveBotConfig(botConfig); |
||||
} |
||||
} |
||||
return ctx.reply('You have been successfully unsubscribed from alerts from ' + command.args); |
||||
} else { |
||||
return ctx.reply('You should use syntax: \/removeNotification <anomaly_name>'); |
||||
} |
||||
} |
||||
|
||||
const Telegraf = require('telegraf'); |
||||
let botConfig: BotConfig; |
||||
let bot; |
||||
|
||||
function tgBotInit() { |
||||
try { |
||||
botConfig = loadBotConfig(); |
||||
bot = new Telegraf(botConfig.token); |
||||
|
||||
bot.use(commandArgs); |
||||
|
||||
bot.command('addNotification', addNotification); |
||||
bot.command('removeNotification', removeNotification); |
||||
|
||||
bot.startPolling(); |
||||
} catch(e) { |
||||
// TODO: handle exception
|
||||
} |
||||
} |
||||
|
||||
export { sendNotification, tgBotInit } |
@ -0,0 +1,75 @@
|
||||
import * as path from 'path'; |
||||
import { getJsonDataSync, writeJsonDataSync } from './json'; |
||||
import { SEGMENTS_PATH } from '../config'; |
||||
import { AnomalyId, loadAnomalyById, saveAnomaly } from './anomalyType'; |
||||
|
||||
function getLabeledSegments(anomalyId: AnomalyId) { |
||||
let filename = path.join(SEGMENTS_PATH, `${anomalyId}_labeled.json`); |
||||
|
||||
let segments = []; |
||||
try { |
||||
segments = getJsonDataSync(filename); |
||||
for (let segment of segments) { |
||||
if (segment.labeled === undefined) { |
||||
segment.labeled = false; |
||||
} |
||||
} |
||||
} catch (e) { |
||||
console.error(e.message); |
||||
} |
||||
return segments; |
||||
} |
||||
|
||||
function getPredictedSegments(anomalyId: AnomalyId) { |
||||
let filename = path.join(SEGMENTS_PATH, `${anomalyId}_segments.json`); |
||||
|
||||
let jsonData; |
||||
try { |
||||
jsonData = getJsonDataSync(filename); |
||||
} catch(e) { |
||||
console.error(e.message); |
||||
jsonData = []; |
||||
} |
||||
return jsonData; |
||||
} |
||||
|
||||
function saveSegments(anomalyId: AnomalyId, segments) { |
||||
let filename = path.join(SEGMENTS_PATH, `${anomalyId}_labeled.json`); |
||||
|
||||
try { |
||||
return writeJsonDataSync(filename, segments); |
||||
} catch(e) { |
||||
console.error(e.message); |
||||
throw new Error('Can`t write to db'); |
||||
} |
||||
} |
||||
|
||||
function insertSegments(anomalyId: AnomalyId, addedSegments, labeled:boolean) { |
||||
// Set status
|
||||
let info = loadAnomalyById(anomalyId); |
||||
let segments = getLabeledSegments(anomalyId); |
||||
|
||||
let nextId = info.next_id; |
||||
let addedIds = [] |
||||
for (let segment of addedSegments) { |
||||
segment.id = nextId; |
||||
segment.labeled = labeled; |
||||
addedIds.push(nextId); |
||||
nextId++; |
||||
segments.push(segment); |
||||
} |
||||
info.next_id = nextId; |
||||
saveSegments(anomalyId, segments); |
||||
saveAnomaly(anomalyId, info); |
||||
return addedIds; |
||||
} |
||||
|
||||
function removeSegments(anomalyId: AnomalyId, removedSegments) { |
||||
let segments = getLabeledSegments(anomalyId); |
||||
for (let segmentId of removedSegments) { |
||||
segments = segments.filter(el => el.id !== segmentId); |
||||
} |
||||
saveSegments(anomalyId, segments); |
||||
} |
||||
|
||||
export { getLabeledSegments, getPredictedSegments, saveSegments, insertSegments, removeSegments } |
@ -0,0 +1,10 @@
|
||||
{ |
||||
"compilerOptions": { |
||||
"outDir": "./dist/", |
||||
"sourceMap": true, |
||||
"noImplicitAny": false, |
||||
"module": "commonjs", |
||||
"target": "es2015", |
||||
"allowJs": true |
||||
} |
||||
} |
@ -0,0 +1,11 @@
|
||||
anomalies/ |
||||
segments/ |
||||
datasets/ |
||||
datasources/ |
||||
models/ |
||||
metrics/ |
||||
__pycache__/ |
||||
*.pyc |
||||
*.txt |
||||
*.log |
||||
tasks.csv |
@ -0,0 +1,5 @@
|
||||
from worker import worker |
||||
|
||||
if __name__ == "__main__": |
||||
w = worker() |
||||
w.do_task({"type": "learn", "anomaly_name": "cpu_utilization_supervised", "segments": []}) |
@ -0,0 +1,157 @@
|
||||
import os.path |
||||
from data_provider import DataProvider |
||||
from data_preprocessor import data_preprocessor |
||||
import json |
||||
import pandas as pd |
||||
import logging |
||||
|
||||
datasource_folder = "datasources/" |
||||
dataset_folder = "datasets/" |
||||
anomalies_folder = "anomalies/" |
||||
models_folder = "models/" |
||||
metrics_folder = "metrics/" |
||||
logger = logging.getLogger('analytic_toolset') |
||||
|
||||
|
||||
def anomalies_to_timestamp(anomalies): |
||||
for anomaly in anomalies: |
||||
anomaly['start'] = int(anomaly['start'].timestamp() * 1000) |
||||
anomaly['finish'] = int(anomaly['finish'].timestamp() * 1000) |
||||
return anomalies |
||||
|
||||
|
||||
class AnomalyModel: |
||||
|
||||
def __init__(self, anomaly_name): |
||||
self.anomaly_name = anomaly_name |
||||
self.load_anomaly_config() |
||||
|
||||
datasource = self.anomaly_config['metric']['datasource'] |
||||
metric_name = self.anomaly_config['metric']['targets'][0] |
||||
|
||||
dbconfig_filename = os.path.join(datasource_folder, datasource + ".json") |
||||
target_filename = os.path.join(metrics_folder, metric_name + ".json") |
||||
|
||||
dataset_filename = os.path.join(dataset_folder, metric_name + ".csv") |
||||
augmented_path = os.path.join(dataset_folder, metric_name + "_augmented.csv") |
||||
|
||||
with open(dbconfig_filename, 'r') as config_file: |
||||
dbconfig = json.load(config_file) |
||||
|
||||
with open(target_filename, 'r') as file: |
||||
target = json.load(file) |
||||
|
||||
self.data_prov = DataProvider(dbconfig, target, dataset_filename) |
||||
self.preprocessor = data_preprocessor(self.data_prov, augmented_path) |
||||
self.model = None |
||||
|
||||
self.__load_model() |
||||
|
||||
def anomalies_box(self, anomalies): |
||||
max_time = 0 |
||||
min_time = float("inf") |
||||
for anomaly in anomalies: |
||||
max_time = max(max_time, anomaly['finish']) |
||||
min_time = min(min_time, anomaly['start']) |
||||
min_time = pd.to_datetime(min_time, unit='ms') |
||||
max_time = pd.to_datetime(max_time, unit='ms') |
||||
return min_time, max_time |
||||
|
||||
def learn(self, anomalies): |
||||
logger.info("Start to learn for anomaly_name='%s'" % self.anomaly_name) |
||||
|
||||
confidence = 0.02 |
||||
dataframe = self.data_prov.get_dataframe() |
||||
start_index, stop_index = 0, len(dataframe) |
||||
if len(anomalies) > 0: |
||||
confidence = 0.0 |
||||
min_time, max_time = self.anomalies_box(anomalies) |
||||
start_index = dataframe[dataframe['timestamp'] >= min_time].index[0] |
||||
stop_index = dataframe[dataframe['timestamp'] > max_time].index[0] |
||||
start_index, stop_index = self.preprocessor.expand_indexes(start_index, stop_index) |
||||
dataframe = dataframe[start_index:stop_index] |
||||
|
||||
train_augmented = self.preprocessor.get_augmented_data( |
||||
start_index, |
||||
stop_index, |
||||
anomalies |
||||
) |
||||
|
||||
self.model = self.create_algorithm() |
||||
self.model.fit(train_augmented, confidence) |
||||
if len(anomalies) > 0: |
||||
last_dataframe_time = dataframe.iloc[- 1]['timestamp'] |
||||
last_prediction_time = int(last_dataframe_time.timestamp() * 1000) |
||||
else: |
||||
last_prediction_time = 0 |
||||
|
||||
self.__save_model() |
||||
logger.info("Learning is finished for anomaly_name='%s'" % self.anomaly_name) |
||||
return last_prediction_time |
||||
|
||||
def predict(self, last_prediction_time): |
||||
logger.info("Start to predict for anomaly type='%s'" % self.anomaly_name) |
||||
last_prediction_time = pd.to_datetime(last_prediction_time, unit='ms') |
||||
|
||||
start_index = self.data_prov.get_upper_bound(last_prediction_time) |
||||
stop_index = self.data_prov.size() |
||||
|
||||
# last_prediction_time = pd.to_datetime(last_prediction_time, unit='ms') |
||||
# dataframe = dataframe[dataframe['timestamp'] > last_prediction_time] |
||||
last_prediction_time = int(last_prediction_time.timestamp() * 1000) |
||||
|
||||
predicted_anomalies = [] |
||||
if start_index < stop_index: |
||||
max_chunk_size = 50000 |
||||
predicted = pd.Series() |
||||
for index in range(start_index, stop_index, max_chunk_size): |
||||
chunk_start = index |
||||
chunk_finish = min(index + max_chunk_size, stop_index) |
||||
predict_augmented = self.preprocessor.get_augmented_data(chunk_start, chunk_finish) |
||||
|
||||
assert(len(predict_augmented) == chunk_finish - chunk_start) |
||||
|
||||
predicted_current = self.model.predict(predict_augmented) |
||||
predicted = pd.concat([predicted, predicted_current]) |
||||
predicted_anomalies = self.preprocessor.inverse_transform_anomalies(predicted) |
||||
|
||||
last_row = self.data_prov.get_data_range(stop_index - 1, stop_index) |
||||
|
||||
last_dataframe_time = last_row.iloc[0]['timestamp'] |
||||
predicted_anomalies = anomalies_to_timestamp(predicted_anomalies) |
||||
last_prediction_time = int(last_dataframe_time.timestamp() * 1000) |
||||
|
||||
logger.info("Predicting is finished for anomaly type='%s'" % self.anomaly_name) |
||||
return predicted_anomalies, last_prediction_time |
||||
|
||||
def synchronize_data(self): |
||||
self.data_prov.synchronize() |
||||
self.preprocessor.set_data_provider(self.data_prov) |
||||
self.preprocessor.synchronize() |
||||
|
||||
def load_anomaly_config(self): |
||||
with open(os.path.join(anomalies_folder, self.anomaly_name + ".json"), 'r') as config_file: |
||||
self.anomaly_config = json.load(config_file) |
||||
|
||||
def get_anomalies(self): |
||||
labeled_anomalies_file = os.path.join(anomalies_folder, self.anomaly_name + "_labeled.json") |
||||
if not os.path.exists(labeled_anomalies_file): |
||||
return [] |
||||
with open(labeled_anomalies_file) as file: |
||||
return json.load(file) |
||||
|
||||
def create_algorithm(self): |
||||
from supervised_algorithm import supervised_algorithm |
||||
return supervised_algorithm() |
||||
|
||||
def __save_model(self): |
||||
logger.info("Save model '%s'" % self.anomaly_name) |
||||
model_filename = os.path.join(models_folder, self.anomaly_name + ".m") |
||||
self.model.save(model_filename) |
||||
|
||||
def __load_model(self): |
||||
logger.info("Load model '%s'" % self.anomaly_name) |
||||
model_filename = os.path.join(models_folder, self.anomaly_name + ".m") |
||||
if os.path.exists(model_filename): |
||||
self.model = self.create_algorithm() |
||||
self.model.load(model_filename) |
@ -0,0 +1,255 @@
|
||||
import os.path |
||||
import pandas as pd |
||||
import numpy as np |
||||
import math |
||||
import time |
||||
|
||||
from tsfresh.transformers.feature_augmenter import FeatureAugmenter |
||||
from tsfresh.feature_extraction.settings import from_columns |
||||
from pytz import timezone |
||||
|
||||
|
||||
class data_preprocessor: |
||||
# augmented = None |
||||
frame_size = 16 |
||||
calc_features = [ |
||||
# "value__agg_linear_trend__f_agg_\"max\"__chunk_len_5__attr_\"intercept\"", |
||||
# "value__cwt_coefficients__widths_(2, 5, 10, 20)__coeff_12__w_20", |
||||
# "value__cwt_coefficients__widths_(2, 5, 10, 20)__coeff_13__w_5", |
||||
# "value__cwt_coefficients__widths_(2, 5, 10, 20)__coeff_2__w_10", |
||||
# "value__cwt_coefficients__widths_(2, 5, 10, 20)__coeff_2__w_20", |
||||
# "value__cwt_coefficients__widths_(2, 5, 10, 20)__coeff_8__w_20", |
||||
# "value__fft_coefficient__coeff_3__attr_\"abs\"", |
||||
"time_of_day_column_x", |
||||
"time_of_day_column_y", |
||||
"value__abs_energy", |
||||
"value__absolute_sum_of_changes", |
||||
"value__sum_of_reoccurring_data_points", |
||||
] |
||||
time_features = [ |
||||
'time_of_day_column_x', |
||||
'time_of_day_column_y' |
||||
] |
||||
chunk_size = 50000 |
||||
|
||||
def __init__(self, data_provider, augmented_path): |
||||
self.data_provider = data_provider |
||||
self.augmented_path = augmented_path |
||||
self.last_chunk_index = 0 |
||||
self.total_size = 0 |
||||
self.__init_chunks() |
||||
self.synchronize() |
||||
|
||||
def set_data_provider(self, data_provider): |
||||
self.data_provider = data_provider |
||||
|
||||
def synchronize(self): |
||||
start_frame = self.total_size |
||||
stop_frame = self.data_provider.size() |
||||
|
||||
max_chunk_size = 30000 |
||||
for frame in range(start_frame, stop_frame, max_chunk_size): |
||||
data = self.__get_source_frames(frame, min(stop_frame, frame + max_chunk_size)) |
||||
|
||||
if len(data) == 0: |
||||
return |
||||
|
||||
append_augmented = self.__extract_features(data, self.calc_features) |
||||
self.__append_data(append_augmented) |
||||
|
||||
def expand_indexes(self, start_index, stop_index): |
||||
return start_index, stop_index |
||||
|
||||
def get_augmented_data(self, start_index, stop_index, anomalies=[]): |
||||
start_frame = start_index |
||||
stop_frame = stop_index |
||||
augmented = self.__get_data(start_frame, stop_frame) |
||||
|
||||
if len(anomalies) > 0: |
||||
anomalies_indexes = self.transform_anomalies(anomalies) |
||||
augmented = augmented.drop(anomalies_indexes) |
||||
|
||||
return augmented |
||||
|
||||
def transform_anomalies(self, anomalies): |
||||
anomaly_index = None |
||||
dataframe = self.data_provider.get_dataframe(None) |
||||
for anomaly in anomalies: |
||||
start_time = pd.to_datetime(anomaly['start'], unit='ms') |
||||
finish_time = pd.to_datetime(anomaly['finish'], unit='ms') |
||||
current_index = (dataframe['timestamp'] >= start_time) & (dataframe['timestamp'] <= finish_time) |
||||
if anomaly_index is not None: |
||||
anomaly_index = (anomaly_index | current_index) |
||||
else: |
||||
anomaly_index = current_index |
||||
|
||||
rows = dataframe[anomaly_index] |
||||
# indexes = np.floor_divide(rows.index, self.frame_size) |
||||
indexes = np.unique(rows.index) |
||||
return indexes |
||||
|
||||
def inverse_transform_anomalies(self, prediction): |
||||
anomalies = [] |
||||
cur_anomaly = None |
||||
source_dataframe = self.data_provider.get_dataframe(None) |
||||
for i in prediction.index: |
||||
if prediction[i]: |
||||
start_frame_index = max(0, i - self.frame_size + 1) |
||||
finish_frame_index = i |
||||
start = source_dataframe['timestamp'][start_frame_index] |
||||
finish = source_dataframe['timestamp'][finish_frame_index] |
||||
if cur_anomaly is None: |
||||
if len(anomalies) > 0 and start <= anomalies[len(anomalies) - 1]['finish']: |
||||
cur_anomaly = anomalies[len(anomalies) - 1] |
||||
anomalies.pop() |
||||
else: |
||||
cur_anomaly = {'start': start, 'finish': finish} |
||||
cur_anomaly['finish'] = finish |
||||
elif cur_anomaly is not None: |
||||
anomalies.append(cur_anomaly) |
||||
cur_anomaly = None |
||||
|
||||
if cur_anomaly: |
||||
anomalies.append(cur_anomaly) |
||||
return anomalies |
||||
|
||||
def __get_data(self, start_index, stop_index): |
||||
result = pd.DataFrame() |
||||
start_chunk = start_index // self.chunk_size |
||||
finish_chunk = stop_index // self.chunk_size |
||||
for chunk_num in range(start_chunk, finish_chunk + 1): |
||||
chunk = self.__load_chunk(chunk_num) |
||||
if chunk_num == finish_chunk: |
||||
chunk = chunk[:stop_index % self.chunk_size] |
||||
if chunk_num == start_chunk: |
||||
chunk = chunk[start_index % self.chunk_size:] |
||||
result = pd.concat([result, chunk]) |
||||
return result |
||||
|
||||
def __init_chunks(self): |
||||
chunk_index = 0 |
||||
self.last_chunk_index = 0 |
||||
while True: |
||||
filename = self.augmented_path |
||||
if chunk_index > 0: |
||||
filename += "." + str(chunk_index) |
||||
if os.path.exists(filename): |
||||
self.last_chunk_index = chunk_index |
||||
else: |
||||
break |
||||
chunk_index += 1 |
||||
self.total_size = self.last_chunk_index * self.chunk_size |
||||
last_chunk = self.__load_chunk(self.last_chunk_index) |
||||
self.total_size += len(last_chunk) |
||||
|
||||
def __append_data(self, dataframe): |
||||
while len(dataframe) > 0: |
||||
chunk = self.__load_chunk(self.last_chunk_index) |
||||
rows_count = min(self.chunk_size - len(chunk), len(dataframe)) |
||||
|
||||
rows = dataframe.iloc[0:rows_count] |
||||
self.__save_chunk(self.last_chunk_index, rows) |
||||
self.total_size += rows_count |
||||
|
||||
dataframe = dataframe[rows_count:] |
||||
if len(dataframe) > 0: |
||||
self.last_chunk_index += 1 |
||||
|
||||
def __load_chunk(self, index): |
||||
filename = self.augmented_path |
||||
if index > 0: |
||||
filename += "." + str(index) |
||||
|
||||
if os.path.exists(filename): |
||||
chunk = pd.read_csv(filename) |
||||
frame_index = np.arange(index * self.chunk_size, index * self.chunk_size + len(chunk)) |
||||
chunk = chunk.set_index(frame_index) |
||||
return chunk |
||||
return pd.DataFrame() |
||||
|
||||
def __save_chunk(self, index, dataframe): |
||||
filename = self.augmented_path |
||||
if index > 0: |
||||
filename += "." + str(index) |
||||
|
||||
if os.path.exists(filename): |
||||
dataframe.to_csv(filename, mode='a', index=False, header=False) |
||||
else: |
||||
dataframe.to_csv(filename, mode='w', index=False, header=True) |
||||
|
||||
def __get_source_frames(self, start_frame, stop_frame): |
||||
start_index = start_frame |
||||
stop_index = stop_frame |
||||
|
||||
# frame = self.source_dataframe[start_index:stop_index] |
||||
# mat = frame.as_matrix() |
||||
|
||||
source_dataframe = self.data_provider.get_data_range(max(start_index - self.frame_size + 1, 0), stop_index) |
||||
|
||||
dataframe = None |
||||
for i in range(start_index, stop_index): |
||||
mini = max(0, i - self.frame_size + 1) |
||||
frame = source_dataframe.loc[mini:i + 1].copy() |
||||
frame['id'] = i |
||||
if dataframe is None: |
||||
dataframe = frame |
||||
else: |
||||
dataframe = dataframe.append(frame, ignore_index=True) |
||||
|
||||
#dataframe = self.source_dataframe[start_index:stop_index].copy() |
||||
#dataframe['id'] = np.floor_divide(dataframe.index, self.frame_size) |
||||
dataframe.reset_index(drop=True, inplace=True) |
||||
return dataframe |
||||
|
||||
def __extract_features(self, data, features=None): |
||||
start_frame = data['id'][0] |
||||
stop_frame = data['id'][len(data)-1] + 1 |
||||
augmented = pd.DataFrame(index=np.arange(start_frame, stop_frame)) |
||||
|
||||
# tsfresh features |
||||
tsfresh_features = None |
||||
if features is not None: |
||||
tsfresh_features = set(features) - set(self.time_features) |
||||
|
||||
augmented = self.__extract_tfresh_features(data, augmented, tsfresh_features) |
||||
|
||||
# time features |
||||
augmented = self.__extract_time_features(data, augmented, features) |
||||
return augmented |
||||
|
||||
def __extract_tfresh_features(self, data, augmented, features): |
||||
relevant_extraction_settings = None |
||||
if features is not None: |
||||
augmented_features = set(features) |
||||
relevant_extraction_settings = from_columns(augmented_features) |
||||
|
||||
#impute_function = partial(impute_dataframe_range, col_to_max=self.col_to_max, |
||||
# col_to_min=self.col_to_min, col_to_median=self.col_to_median) |
||||
|
||||
feature_extractor = FeatureAugmenter( |
||||
kind_to_fc_parameters=relevant_extraction_settings, |
||||
column_id='id', |
||||
column_sort='timestamp') |
||||
feature_extractor.set_timeseries_container(data) |
||||
|
||||
return feature_extractor.transform(augmented) |
||||
|
||||
def __extract_time_features(self, data, augmented, features): |
||||
if features is None: |
||||
features = self.time_features |
||||
|
||||
seconds = np.zeros(len(augmented)) |
||||
first_id = data['id'][0] |
||||
|
||||
for i in range(len(data)): |
||||
id = data['id'][i] - first_id |
||||
timeobj = data['timestamp'][i].time() |
||||
seconds[id] = timeobj.second + 60 * (timeobj.minute + 60 * timeobj.hour) |
||||
|
||||
norm_seconds = 2 * math.pi * seconds / (24 * 3600) |
||||
|
||||
if 'time_of_day_column_x' in features: |
||||
augmented['time_of_day_column_x'] = np.cos(norm_seconds) |
||||
if 'time_of_day_column_y' in features: |
||||
augmented['time_of_day_column_y'] = np.sin(norm_seconds) |
||||
return augmented |
@ -0,0 +1,220 @@
|
||||
from influxdb import InfluxDBClient |
||||
import pandas as pd |
||||
import os.path |
||||
import numpy as np |
||||
|
||||
|
||||
class DataProvider: |
||||
chunk_size = 50000 |
||||
|
||||
def __init__(self, dbconfig, target, data_filename): |
||||
self.dbconfig = dbconfig |
||||
self.target = target |
||||
self.data_filename = data_filename |
||||
self.last_time = None |
||||
self.total_size = 0 |
||||
self.last_chunk_index = 0 |
||||
self.chunk_last_times = {} |
||||
self.__init_chunks() |
||||
self.synchronize() |
||||
|
||||
def get_dataframe(self, after_time=None): |
||||
result = pd.DataFrame() |
||||
for chunk_index, last_chunk_time in self.chunk_last_times.items(): |
||||
if after_time is None or after_time <= last_chunk_time: |
||||
chunk = self.__load_chunk(chunk_index) |
||||
if after_time is not None: |
||||
chunk = chunk[chunk['timestamp'] > after_time] |
||||
result = pd.concat([result, chunk]) |
||||
return result |
||||
|
||||
def get_upper_bound(self, after_time): |
||||
for chunk_index, last_chunk_time in self.chunk_last_times.items(): |
||||
if after_time < last_chunk_time: |
||||
chunk = self.__load_chunk(chunk_index) |
||||
chunk = chunk[chunk['timestamp'] > after_time] |
||||
return chunk.index[0] |
||||
return self.size() |
||||
|
||||
def size(self): |
||||
return self.total_size |
||||
|
||||
def get_data_range(self, start_index, stop_index=None): |
||||
return self.__get_data(start_index, stop_index) |
||||
|
||||
def transform_anomalies(self, anomalies): |
||||
result = [] |
||||
if len(anomalies) == 0: |
||||
return result |
||||
dataframe = self.get_dataframe(None) |
||||
for anomaly in anomalies: |
||||
start_time = pd.to_datetime(anomaly['start']-1, unit='ms') |
||||
finish_time = pd.to_datetime(anomaly['finish']+1, unit='ms') |
||||
current_index = (dataframe['timestamp'] >= start_time) & (dataframe['timestamp'] <= finish_time) |
||||
anomaly_frame = dataframe[current_index] |
||||
cur_anomaly = { |
||||
'start': anomaly_frame.index[0], |
||||
'finish': anomaly_frame.index[len(anomaly_frame) - 1], |
||||
'labeled': anomaly['labeled'] |
||||
} |
||||
result.append(cur_anomaly) |
||||
|
||||
return result |
||||
|
||||
def inverse_transform_indexes(self, indexes): |
||||
if len(indexes) == 0: |
||||
return [] |
||||
dataframe = self.get_data_range(indexes[0][0], indexes[-1][1] + 1) |
||||
|
||||
return [(dataframe['timestamp'][i1], dataframe['timestamp'][i2]) for (i1, i2) in indexes] |
||||
|
||||
def synchronize(self): |
||||
# last_time = None |
||||
# if len(self.dataframe/) > 0: |
||||
# last_time = self.dataframe['time'][len(self.dataframe)-1] |
||||
append_dataframe = self.load_from_db(self.last_time) |
||||
self.__append_data(append_dataframe) |
||||
# append_dataframe |
||||
# append_dataframe.to_csv(self.data_filename, mode='a', index=False, header=False) |
||||
# self.dataframe = pd.concat([self.dataframe, append_dataframe], ignore_index=True) |
||||
|
||||
# def load(self): |
||||
# if os.path.exists(self.data_filename): |
||||
# self.dataframe = pd.read_csv(self.data_filename, parse_dates=[0]) |
||||
# self.synchronize() |
||||
# else: |
||||
# append_dataframe = self.load_from_db() |
||||
# self.__append_data(append_dataframe) |
||||
# #self.dataframe.to_csv(self.data_filename, index=False, header=True) |
||||
|
||||
def custom_query(self, after_time): |
||||
query = self.target["query"] |
||||
timeFilter = "TRUE" |
||||
if after_time is not None: |
||||
timeFilter = "time > '%s'" % (str(after_time)) |
||||
query = query.replace("$timeFilter", timeFilter) |
||||
return query |
||||
|
||||
def load_from_db(self, after_time=None): |
||||
"""Instantiate a connection to the InfluxDB.""" |
||||
host = self.dbconfig['host'] |
||||
port = self.dbconfig['port'] |
||||
user = self.dbconfig['user'] |
||||
password = self.dbconfig['password'] |
||||
dbname = self.dbconfig['dbname'] |
||||
|
||||
client = InfluxDBClient(host, port, user, password, dbname) |
||||
# query = 'select k0, k1, k2 from vals;' |
||||
|
||||
measurement = self.target['measurement'] |
||||
select = self.target['select'] |
||||
tags = self.target['tags'] |
||||
|
||||
if "query" in self.target: |
||||
query = self.custom_query(after_time) |
||||
else: |
||||
select_values = select[0][0]['params'] |
||||
escaped_select_values = ["\"" + value + "\"" for value in select_values] |
||||
|
||||
conditions_entries = [] |
||||
if len(tags) > 0: |
||||
for tag in tags: |
||||
conditions_entries.append("(\"" + tag['key'] + "\"" + tag['operator'] + "'" + tag['value'] + "')") |
||||
if after_time: |
||||
conditions_entries.append("time > '%s'" % (str(after_time))) |
||||
|
||||
condition = "" |
||||
if len(conditions_entries) > 0: |
||||
condition = " where " + " AND ".join(conditions_entries) |
||||
|
||||
query = "select %s from \"%s\"%s;" % (",".join(escaped_select_values), measurement, condition) |
||||
|
||||
result = client.query(query, chunked=True, chunk_size=10000) |
||||
dataframe = pd.DataFrame(result.get_points()) |
||||
if len(dataframe) > 0: |
||||
cols = dataframe.columns.tolist() |
||||
cols.remove('time') |
||||
cols = ['time'] + cols |
||||
dataframe = dataframe[cols] |
||||
|
||||
dataframe['time'] = pd.to_datetime(dataframe['time']) |
||||
dataframe = dataframe.dropna(axis=0, how='any') |
||||
|
||||
return dataframe |
||||
|
||||
def __init_chunks(self): |
||||
chunk_index = 0 |
||||
self.last_chunk_index = 0 |
||||
while True: |
||||
filename = self.data_filename |
||||
if chunk_index > 0: |
||||
filename += "." + str(chunk_index) |
||||
if os.path.exists(filename): |
||||
self.last_chunk_index = chunk_index |
||||
chunk = self.__load_chunk(chunk_index) |
||||
chunk_last_time = chunk.iloc[len(chunk) - 1]['timestamp'] |
||||
self.chunk_last_times[chunk_index] = chunk_last_time |
||||
self.last_time = chunk_last_time |
||||
else: |
||||
break |
||||
chunk_index += 1 |
||||
self.total_size = self.last_chunk_index * self.chunk_size |
||||
last_chunk = self.__load_chunk(self.last_chunk_index) |
||||
self.total_size += len(last_chunk) |
||||
|
||||
def __load_chunk(self, index): |
||||
filename = self.data_filename |
||||
if index > 0: |
||||
filename += "." + str(index) |
||||
|
||||
if os.path.exists(filename): |
||||
chunk = pd.read_csv(filename, parse_dates=[0]) |
||||
frame_index = np.arange(index * self.chunk_size, index * self.chunk_size + len(chunk)) |
||||
chunk = chunk.set_index(frame_index) |
||||
return chunk.rename(columns={chunk.columns[0]: "timestamp", chunk.columns[1]: "value"}) |
||||
return pd.DataFrame() |
||||
|
||||
def __save_chunk(self, index, dataframe): |
||||
filename = self.data_filename |
||||
if index > 0: |
||||
filename += "." + str(index) |
||||
|
||||
chunk_last_time = dataframe.iloc[len(dataframe) - 1]['time'] |
||||
self.chunk_last_times[index] = chunk_last_time |
||||
|
||||
if os.path.exists(filename): |
||||
dataframe.to_csv(filename, mode='a', index=False, header=False) |
||||
else: |
||||
dataframe.to_csv(filename, mode='w', index=False, header=True) |
||||
|
||||
def __append_data(self, dataframe): |
||||
while len(dataframe) > 0: |
||||
chunk = self.__load_chunk(self.last_chunk_index) |
||||
rows_count = min(self.chunk_size - len(chunk), len(dataframe)) |
||||
|
||||
rows = dataframe.iloc[0:rows_count] |
||||
|
||||
if len(rows) > 0: |
||||
self.__save_chunk(self.last_chunk_index, rows) |
||||
self.total_size += rows_count |
||||
|
||||
self.last_time = rows.iloc[-1]['time'] |
||||
dataframe = dataframe[rows_count:] |
||||
|
||||
if len(dataframe) > 0: |
||||
self.last_chunk_index += 1 |
||||
|
||||
def __get_data(self, start_index, stop_index): |
||||
result = pd.DataFrame() |
||||
start_chunk = start_index // self.chunk_size |
||||
finish_chunk = self.last_chunk_index |
||||
if stop_index is not None: |
||||
finish_chunk = stop_index // self.chunk_size |
||||
for chunk_num in range(start_chunk, finish_chunk + 1): |
||||
chunk = self.__load_chunk(chunk_num) |
||||
if stop_index is not None and chunk_num == finish_chunk: |
||||
chunk = chunk[:stop_index % self.chunk_size] |
||||
if chunk_num == start_chunk: |
||||
chunk = chunk[start_index % self.chunk_size:] |
||||
result = pd.concat([result, chunk]) |
||||
return result |
@ -0,0 +1,52 @@
|
||||
#!/usr/bin/env python |
||||
import csv |
||||
import os |
||||
from worker import worker |
||||
|
||||
|
||||
def enqueue_task(): |
||||
tasks_file = "tasks.csv" |
||||
tasks = [] |
||||
with open(tasks_file) as csvfile: |
||||
rdr = csv.reader(csvfile, delimiter=',') |
||||
tasks = list(rdr) |
||||
if len(tasks) == 0: |
||||
return None |
||||
res = tasks[0][0] |
||||
tasks = tasks[1:] |
||||
with open(tasks_file, "w+") as csvfile: |
||||
writer = csv.writer(csvfile) |
||||
writer.writerows(tasks) |
||||
return res |
||||
|
||||
|
||||
def set_lock(value): |
||||
lock_file = "learn.lock" |
||||
exists = os.path.exists(lock_file) |
||||
if exists == value: |
||||
return False |
||||
|
||||
if value: |
||||
open(lock_file, "w+") |
||||
else: |
||||
os.remove(lock_file) |
||||
return True |
||||
|
||||
|
||||
if __name__ == "__main__": |
||||
if not set_lock(True): |
||||
print("learn locked") |
||||
exit(0) |
||||
|
||||
w = worker() |
||||
while True: |
||||
task = enqueue_task() |
||||
if task is None: |
||||
break |
||||
|
||||
w.start() |
||||
w.add_task({"type": "learn", "anomaly_name": task}) |
||||
w.add_task({"type": "predict", "anomaly_name": task}) |
||||
w.stop() |
||||
|
||||
set_lock(False) |
@ -0,0 +1,127 @@
|
||||
from data_provider import DataProvider |
||||
import logging |
||||
import os.path |
||||
import json |
||||
import pandas as pd |
||||
|
||||
datasource_folder = "datasources/" |
||||
dataset_folder = "datasets/" |
||||
anomalies_folder = "anomalies/" |
||||
models_folder = "models/" |
||||
metrics_folder = "metrics/" |
||||
logger = logging.getLogger('analytic_toolset') |
||||
|
||||
|
||||
def segments_box(segments): |
||||
max_time = 0 |
||||
min_time = float("inf") |
||||
for segment in segments: |
||||
min_time = min(min_time, segment['start']) |
||||
max_time = max(max_time, segment['finish']) |
||||
min_time = pd.to_datetime(min_time, unit='ms') |
||||
max_time = pd.to_datetime(max_time, unit='ms') |
||||
return min_time, max_time |
||||
|
||||
|
||||
class PatternDetectionModel: |
||||
|
||||
def __init__(self, pattern_name, preset=None): |
||||
self.pattern_name = pattern_name |
||||
self.preset = preset |
||||
|
||||
self.__load_anomaly_config() |
||||
datasource = self.anomaly_config['metric']['datasource'] |
||||
metric_name = self.anomaly_config['metric']['targets'][0] |
||||
|
||||
dbconfig_filename = os.path.join(datasource_folder, datasource + ".json") |
||||
target_filename = os.path.join(metrics_folder, metric_name + ".json") |
||||
|
||||
dataset_filename = os.path.join(dataset_folder, metric_name + ".csv") |
||||
|
||||
with open(dbconfig_filename, 'r') as config_file: |
||||
dbconfig = json.load(config_file) |
||||
|
||||
with open(target_filename, 'r') as file: |
||||
target = json.load(file) |
||||
|
||||
self.data_prov = DataProvider(dbconfig, target, dataset_filename) |
||||
|
||||
self.model = None |
||||
self.__load_model(preset) |
||||
|
||||
def learn(self, segments): |
||||
self.model = self.__create_model(self.preset) |
||||
window_size = 200 |
||||
|
||||
dataframe = self.data_prov.get_dataframe() |
||||
start_index, stop_index = 0, len(dataframe) |
||||
if len(segments) > 0: |
||||
min_time, max_time = segments_box(segments) |
||||
start_index = dataframe[dataframe['timestamp'] >= min_time].index[0] |
||||
stop_index = dataframe[dataframe['timestamp'] > max_time].index[0] |
||||
start_index = max(start_index - window_size, 0) |
||||
stop_index = min(stop_index + window_size, len(dataframe)) |
||||
|
||||
dataframe = dataframe[start_index:stop_index] |
||||
|
||||
segments = self.data_prov.transform_anomalies(segments) |
||||
self.model.fit(dataframe, segments) |
||||
self.__save_model() |
||||
return 0 |
||||
# return last_prediction_time |
||||
|
||||
def predict(self, last_prediction_time): |
||||
if self.model is None: |
||||
return [], last_prediction_time |
||||
|
||||
window_size = 100 |
||||
last_prediction_time = pd.to_datetime(last_prediction_time, unit='ms') |
||||
|
||||
start_index = self.data_prov.get_upper_bound(last_prediction_time) |
||||
start_index = max(0, start_index - window_size) |
||||
dataframe = self.data_prov.get_data_range(start_index) |
||||
|
||||
predicted_indexes = self.model.predict(dataframe) |
||||
predicted_indexes = [(x, y) for (x, y) in predicted_indexes if x >= start_index and y >= start_index] |
||||
|
||||
predicted_times = self.data_prov.inverse_transform_indexes(predicted_indexes) |
||||
segments = [] |
||||
for time_value in predicted_times: |
||||
ts1 = int(time_value[0].timestamp() * 1000) |
||||
ts2 = int(time_value[1].timestamp() * 1000) |
||||
segments.append({ |
||||
'start': ts1, |
||||
'finish': ts2 |
||||
}) |
||||
|
||||
last_dataframe_time = dataframe.iloc[- 1]['timestamp'] |
||||
last_prediction_time = int(last_dataframe_time.timestamp() * 1000) |
||||
return segments, last_prediction_time |
||||
# return predicted_anomalies, last_prediction_time |
||||
|
||||
def synchronize_data(self): |
||||
self.data_prov.synchronize() |
||||
|
||||
def __create_model(self, preset): |
||||
if preset == "peaks": |
||||
from peaks_detector import PeaksDetector |
||||
return PeaksDetector() |
||||
if preset == "steps" or preset == "cliffs": |
||||
from step_detector import StepDetector |
||||
return StepDetector(preset) |
||||
|
||||
def __load_anomaly_config(self): |
||||
with open(os.path.join(anomalies_folder, self.pattern_name + ".json"), 'r') as config_file: |
||||
self.anomaly_config = json.load(config_file) |
||||
|
||||
def __save_model(self): |
||||
logger.info("Save model '%s'" % self.pattern_name) |
||||
model_filename = os.path.join(models_folder, self.pattern_name + ".m") |
||||
self.model.save(model_filename) |
||||
|
||||
def __load_model(self, preset): |
||||
logger.info("Load model '%s'" % self.pattern_name) |
||||
model_filename = os.path.join(models_folder, self.pattern_name + ".m") |
||||
if os.path.exists(model_filename): |
||||
self.model = self.__create_model(preset) |
||||
self.model.load(model_filename) |
@ -0,0 +1,71 @@
|
||||
from scipy import signal |
||||
import numpy as np |
||||
import step_detect |
||||
|
||||
|
||||
class PeaksDetector: |
||||
def __init__(self): |
||||
pass |
||||
|
||||
def fit(self, dataset, contamination=0.005): |
||||
pass |
||||
|
||||
def predict(self, dataframe): |
||||
array = dataframe['value'].as_matrix() |
||||
window_size = 20 |
||||
# window = np.ones(101) |
||||
# mean_filtered = signal.fftconvolve( |
||||
# np.concatenate([np.zeros(window_size), array, np.zeros(window_size)]), |
||||
# window, |
||||
# mode='valid' |
||||
# ) |
||||
# filtered = np.divide(array, mean_filtered / 101) |
||||
|
||||
window = signal.general_gaussian(2 * window_size + 1, p=0.5, sig=5) |
||||
#print(window) |
||||
filtered = signal.fftconvolve(array, window, mode='valid') |
||||
|
||||
# filtered = np.concatenate([ |
||||
# np.zeros(window_size), |
||||
# filtered, |
||||
# np.zeros(window_size) |
||||
# ]) |
||||
filtered = filtered / np.sum(window) |
||||
array = array[window_size:-window_size] |
||||
filtered = np.subtract(array, filtered) |
||||
|
||||
import matplotlib.pyplot as plt |
||||
|
||||
# filtered = np.convolve(array, step, mode='valid') |
||||
# print(len(array)) |
||||
# print(len(filtered)) |
||||
|
||||
# step = np.hstack((np.ones(window_size), 0, -1*np.ones(window_size))) |
||||
# |
||||
# conv = np.convolve(array, step, mode='valid') |
||||
# |
||||
# conv = np.concatenate([ |
||||
# np.zeros(window_size), |
||||
# conv, |
||||
# np.zeros(window_size)]) |
||||
|
||||
#data = step_detect.t_scan(array, window=window_size) |
||||
data = filtered |
||||
data /= data.max() |
||||
|
||||
#plt.plot(array[:1000]) |
||||
plt.plot(data[:1000]) |
||||
plt.show() |
||||
|
||||
result = step_detect.find_steps(data, 0.1) |
||||
return [dataframe.index[x + window_size] for x in result] |
||||
|
||||
def save(self, model_filename): |
||||
pass |
||||
# with open(model_filename, 'wb') as file: |
||||
# pickle.dump((self.clf, self.scaler), file) |
||||
|
||||
def load(self, model_filename): |
||||
pass |
||||
# with open(model_filename, 'rb') as file: |
||||
# self.clf, self.scaler = pickle.load(file) |
@ -0,0 +1,83 @@
|
||||
import argparse |
||||
import csv |
||||
import time |
||||
import datetime |
||||
import pandas as pd |
||||
import matplotlib.pyplot as plt |
||||
|
||||
from influxdb import InfluxDBClient |
||||
from sklearn import svm |
||||
import numpy as np |
||||
import math |
||||
import pickle |
||||
|
||||
|
||||
host = "209.205.120.226" |
||||
port = 8086 |
||||
datasetFile = "/tmp/dataset.csv" |
||||
anomaliesFile = "anomalies.csv" |
||||
predictedAnomaliesFile = "predicted_anomalies.csv" |
||||
modelFilename = 'finalized_model.sav' |
||||
|
||||
|
||||
def readAnomalies(): |
||||
anomalies = [] |
||||
|
||||
with open(anomaliesFile) as csvfile: |
||||
rdr = csv.reader(csvfile, delimiter=',') |
||||
for row in rdr: |
||||
anomaly = (int(row[0]), int(row[1])) |
||||
anomalies.append(anomaly) |
||||
|
||||
return anomalies |
||||
|
||||
|
||||
"""Instantiate a connection to the InfluxDB.""" |
||||
user = '' |
||||
password = '' |
||||
dbname = 'accelerometer' |
||||
query = 'select k0, k1, k2 from vals limit 10000;' |
||||
|
||||
|
||||
client = InfluxDBClient(host, port, user, password, dbname) |
||||
|
||||
def predict(host=host, port=port): |
||||
|
||||
result = client.query(query) |
||||
df = pd.DataFrame(result['vals'], columns=['time', 'k0', 'k1', 'k2']) |
||||
|
||||
basedAnomalies = readAnomalies() |
||||
|
||||
df2 = df.rolling(200, win_type='triang').sum() |
||||
df2['time'] = pd.to_datetime(df2['time']) |
||||
df2 = df2[np.isfinite(df2['k0'])] |
||||
|
||||
print(len(df2)) |
||||
|
||||
|
||||
anomalies = [] |
||||
last_anomaly = (-1, -1) |
||||
with open(modelFilename, 'rb') as fid: |
||||
clf = pickle.load(fid) |
||||
prediction = clf.predict(df2[['k0', 'k1', 'k2']]) |
||||
print(len(prediction)) |
||||
#print(prediction) |
||||
for i in range(len(prediction)): |
||||
if prediction[i] > 0.: |
||||
t = df2['time'][i + 199].timestamp() |
||||
t = ((t + 0 * 3600) * 1000) |
||||
if t < basedAnomalies[len(basedAnomalies) - 1][1]: |
||||
continue |
||||
if t < last_anomaly[1] + 1000: |
||||
last_anomaly = (last_anomaly[0], t) |
||||
else: |
||||
if last_anomaly[1] != -1: |
||||
anomalies.append(last_anomaly) |
||||
last_anomaly = (t, t) |
||||
|
||||
with open(predictedAnomaliesFile, "w") as file: |
||||
for anomaly in anomalies: |
||||
file.write(str(int(anomaly[0])) + "," + str(int(anomaly[1])) + "\n") |
||||
|
||||
predict() |
||||
|
@ -0,0 +1,46 @@
|
||||
from fbprophet import Prophet |
||||
import pandas as pd |
||||
|
||||
|
||||
class prophet_algorithm(object): |
||||
def __init__(self): |
||||
self.model = None |
||||
self.dataset = None |
||||
|
||||
def fit(self, data, anomalies): |
||||
pass |
||||
|
||||
def predict(self, data): |
||||
data = data.reset_index() |
||||
data = data.rename(columns={'timestamp': 'ds', 'value': 'y'}) |
||||
self.dataset = data |
||||
|
||||
self.model = Prophet(yearly_seasonality=False, weekly_seasonality=False, daily_seasonality=True) |
||||
self.model.fit(self.dataset) |
||||
|
||||
future = self.model.make_future_dataframe(freq='H', periods=0, include_history=True) |
||||
forecast = self.model.predict(future) |
||||
cmp_df = forecast.set_index('ds')[['yhat', 'yhat_lower', 'yhat_upper']].join(self.dataset.set_index('ds')) |
||||
cmp_df['e'] = [ max(row.y - row.yhat_upper, row.yhat_lower - row.y, 0) for index, row in cmp_df.iterrows() ] |
||||
return self.__calc_anomalies(cmp_df) |
||||
|
||||
def __calc_anomalies(self, dataset): |
||||
anomalies = [] |
||||
cur_anomaly = None |
||||
for i in range(len(dataset)): |
||||
if dataset['e'][i] > 17: |
||||
if cur_anomaly is None: |
||||
cur_anomaly = {'start': dataset.index[i], 'finish': dataset.index[i], 'weight': 0} |
||||
cur_anomaly['finish'] = dataset.index[i] |
||||
cur_anomaly['weight'] += dataset['e'][i] |
||||
elif cur_anomaly is not None: |
||||
anomalies.append(cur_anomaly) |
||||
cur_anomaly = None |
||||
return anomalies |
||||
|
||||
|
||||
if __name__ == "__main__": |
||||
dataset = pd.read_csv('art_daily_flatmiddle.csv', index_col=['timestamp'], parse_dates=['timestamp']) |
||||
algo = prophet_algorithm(dataset) |
||||
res = algo.fit() |
||||
print(res) |
@ -0,0 +1,231 @@
|
||||
|
||||
""" |
||||
Thomas Kahn |
||||
thomas.b.kahn@gmail.com |
||||
""" |
||||
from __future__ import absolute_import |
||||
from math import sqrt |
||||
import multiprocessing as mp |
||||
import numpy as np |
||||
from six.moves import range |
||||
from six.moves import zip |
||||
|
||||
|
||||
def t_scan(L, window = 1e3, num_workers = -1): |
||||
""" |
||||
Computes t statistic for i to i+window points versus i-window to i |
||||
points for each point i in input array. Uses multiple processes to |
||||
do this calculation asynchronously. Array is decomposed into window |
||||
number of frames, each consisting of points spaced at window |
||||
intervals. This optimizes the calculation, as the drone function |
||||
need only compute the mean and variance for each set once. |
||||
Parameters |
||||
---------- |
||||
L : numpy array |
||||
1 dimensional array that represents time series of datapoints |
||||
window : int / float |
||||
Number of points that comprise the windows of data that are |
||||
compared |
||||
num_workers : int |
||||
Number of worker processes for multithreaded t_stat computation |
||||
Defult value uses num_cpu - 1 workers |
||||
Returns |
||||
------- |
||||
t_stat : numpy array |
||||
Array which holds t statistic values for each point. The first |
||||
and last (window) points are replaced with zero, since the t |
||||
statistic calculation cannot be performed in that case. |
||||
""" |
||||
size = L.size |
||||
window = int(window) |
||||
frames = list(range(window)) |
||||
n_cols = (size // window) - 1 |
||||
|
||||
t_stat = np.zeros((window, n_cols)) |
||||
|
||||
if num_workers == 1: |
||||
results = [_t_scan_drone(L, n_cols, frame, window) for frame in frames] |
||||
else: |
||||
if num_workers == -1: |
||||
num_workers = mp.cpu_count() - 1 |
||||
pool = mp.Pool(processes = num_workers) |
||||
results = [pool.apply_async(_t_scan_drone, args=(L, n_cols, frame, window)) for frame in frames] |
||||
results = [r.get() for r in results] |
||||
pool.close() |
||||
|
||||
for index, row in results: |
||||
t_stat[index] = row |
||||
|
||||
t_stat = np.concatenate(( |
||||
np.zeros(window), |
||||
t_stat.transpose().ravel(order='C'), |
||||
np.zeros(size % window) |
||||
)) |
||||
|
||||
return t_stat |
||||
|
||||
|
||||
def _t_scan_drone(L, n_cols, frame, window=1e3): |
||||
""" |
||||
Drone function for t_scan. Not Intended to be called manually. |
||||
Computes t_scan for the designated frame, and returns result as |
||||
array along with an integer tag for proper placement in the |
||||
aggregate array |
||||
""" |
||||
size = L.size |
||||
window = int(window) |
||||
root_n = sqrt(window) |
||||
|
||||
output = np.zeros(n_cols) |
||||
b = L[frame:window+frame] |
||||
b_mean = b.mean() |
||||
b_var = b.var() |
||||
for i in range(window+frame, size-window, window): |
||||
a = L[i:i+window] |
||||
a_mean = a.mean() |
||||
a_var = a.var() |
||||
output[i // window - 1] = root_n * (a_mean - b_mean) / sqrt(a_var + b_var) |
||||
b_mean, b_var = a_mean, a_var |
||||
|
||||
return frame, output |
||||
|
||||
|
||||
def mz_fwt(x, n=2): |
||||
""" |
||||
Computes the multiscale product of the Mallat-Zhong discrete forward |
||||
wavelet transform up to and including scale n for the input data x. |
||||
If n is even, the spikes in the signal will be positive. If n is odd |
||||
the spikes will match the polarity of the step (positive for steps |
||||
up, negative for steps down). |
||||
This function is essentially a direct translation of the MATLAB code |
||||
provided by Sadler and Swami in section A.4 of the following: |
||||
http://www.dtic.mil/dtic/tr/fulltext/u2/a351960.pdf |
||||
Parameters |
||||
---------- |
||||
x : numpy array |
||||
1 dimensional array that represents time series of data points |
||||
n : int |
||||
Highest scale to multiply to |
||||
Returns |
||||
------- |
||||
prod : numpy array |
||||
The multiscale product for x |
||||
""" |
||||
N_pnts = x.size |
||||
lambda_j = [1.5, 1.12, 1.03, 1.01][0:n] |
||||
if n > 4: |
||||
lambda_j += [1.0]*(n-4) |
||||
|
||||
H = np.array([0.125, 0.375, 0.375, 0.125]) |
||||
G = np.array([2.0, -2.0]) |
||||
|
||||
Gn = [2] |
||||
Hn = [3] |
||||
for j in range(1,n): |
||||
q = 2**(j-1) |
||||
Gn.append(q+1) |
||||
Hn.append(3*q+1) |
||||
|
||||
S = np.concatenate((x[::-1], x)) |
||||
S = np.concatenate((S, x[::-1])) |
||||
prod = np.ones(N_pnts) |
||||
for j in range(n): |
||||
n_zeros = 2**j - 1 |
||||
Gz = _insert_zeros(G, n_zeros) |
||||
Hz = _insert_zeros(H, n_zeros) |
||||
current = (1.0/lambda_j[j])*np.convolve(S,Gz) |
||||
current = current[N_pnts+Gn[j]:2*N_pnts+Gn[j]] |
||||
prod *= current |
||||
if j == n-1: |
||||
break |
||||
S_new = np.convolve(S, Hz) |
||||
S_new = S_new[N_pnts+Hn[j]:2*N_pnts+Hn[j]] |
||||
S = np.concatenate((S_new[::-1], S_new)) |
||||
S = np.concatenate((S, S_new[::-1])) |
||||
return prod |
||||
|
||||
|
||||
def _insert_zeros(x, n): |
||||
""" |
||||
Helper function for mz_fwt. Splits input array and adds n zeros |
||||
between values. |
||||
""" |
||||
newlen = (n+1)*x.size |
||||
out = np.zeros(newlen) |
||||
indices = list(range(0, newlen-n, n+1)) |
||||
out[indices] = x |
||||
return out |
||||
|
||||
|
||||
def find_steps(array, threshold): |
||||
""" |
||||
Finds local maxima by segmenting array based on positions at which |
||||
the threshold value is crossed. Note that this thresholding is |
||||
applied after the absolute value of the array is taken. Thus, |
||||
the distinction between upward and downward steps is lost. However, |
||||
get_step_sizes can be used to determine directionality after the |
||||
fact. |
||||
Parameters |
||||
---------- |
||||
array : numpy array |
||||
1 dimensional array that represents time series of data points |
||||
threshold : int / float |
||||
Threshold value that defines a step |
||||
Returns |
||||
------- |
||||
steps : list |
||||
List of indices of the detected steps |
||||
""" |
||||
steps = [] |
||||
array = np.abs(array) |
||||
above_points = np.where(array > threshold, 1, 0) |
||||
ap_dif = np.diff(above_points) |
||||
cross_ups = np.where(ap_dif == 1)[0] |
||||
cross_dns = np.where(ap_dif == -1)[0] |
||||
for upi, dni in zip(cross_ups,cross_dns): |
||||
steps.append(np.argmax(array[upi:dni]) + upi) |
||||
return steps |
||||
|
||||
|
||||
def get_step_sizes(array, indices, window=1000): |
||||
""" |
||||
Calculates step size for each index within the supplied list. Step |
||||
size is determined by averaging over a range of points (specified |
||||
by the window parameter) before and after the index of step |
||||
occurrence. The directionality of the step is reflected by the sign |
||||
of the step size (i.e. a positive value indicates an upward step, |
||||
and a negative value indicates a downward step). The combined |
||||
standard deviation of both measurements (as a measure of uncertainty |
||||
in step calculation) is also provided. |
||||
Parameters |
||||
---------- |
||||
array : numpy array |
||||
1 dimensional array that represents time series of data points |
||||
indices : list |
||||
List of indices of the detected steps (as provided by |
||||
find_steps, for example) |
||||
window : int, optional |
||||
Number of points to average over to determine baseline levels |
||||
before and after step. |
||||
Returns |
||||
------- |
||||
step_sizes : list |
||||
List of the calculated sizes of each step |
||||
step_error : list |
||||
""" |
||||
step_sizes = [] |
||||
step_error = [] |
||||
indices = sorted(indices) |
||||
last = len(indices) - 1 |
||||
for i, index in enumerate(indices): |
||||
if i == 0: |
||||
q = min(window, indices[i+1]-index) |
||||
elif i == last: |
||||
q = min(window, index - indices[i-1]) |
||||
else: |
||||
q = min(window, index-indices[i-1], indices[i+1]-index) |
||||
a = array[index:index+q] |
||||
b = array[index-q:index] |
||||
step_sizes.append(a.mean() - b.mean()) |
||||
step_error.append(sqrt(a.var()+b.var())) |
||||
return step_sizes, step_error |
@ -0,0 +1,188 @@
|
||||
import numpy as np |
||||
import pickle |
||||
|
||||
|
||||
def find_segments(array, threshold): |
||||
segments = [] |
||||
above_points = np.where(array > threshold, 1, 0) |
||||
ap_dif = np.diff(above_points) |
||||
cross_ups = np.where(ap_dif == 1)[0] |
||||
cross_dns = np.where(ap_dif == -1)[0] |
||||
for upi, dni in zip(cross_ups,cross_dns): |
||||
segments.append((upi, dni)) |
||||
return segments |
||||
|
||||
|
||||
def is_intersect(target_segment, segments): |
||||
for segment in segments: |
||||
start = max(segment['start'], target_segment[0]) |
||||
finish = min(segment['finish'], target_segment[1]) |
||||
if start <= finish: |
||||
return True |
||||
return False |
||||
|
||||
|
||||
def calc_intersections(segments, finded_segments): |
||||
intersections = 0 |
||||
labeled = 0 |
||||
for segment in segments: |
||||
if not segment['labeled']: |
||||
continue |
||||
|
||||
labeled += 1 |
||||
intersect = False |
||||
for finded_segment in finded_segments: |
||||
start = max(segment['start'], finded_segment[0]) |
||||
finish = min(segment['finish'], finded_segment[1]) |
||||
if start <= finish: |
||||
intersect = True |
||||
break |
||||
if intersect: |
||||
intersections += 1 |
||||
return intersections, labeled |
||||
|
||||
|
||||
def cost_function(segments, finded_segments): |
||||
intersections, labeled = calc_intersections(segments, finded_segments) |
||||
return intersections == labeled |
||||
|
||||
|
||||
def compress_segments(segments): |
||||
result = [] |
||||
for segment in segments: |
||||
if len(result) == 0 or result[len(result) - 1][1] < segment[0]: |
||||
result.append(segment) |
||||
else: |
||||
result[len(result) - 1] = (result[len(result) - 1][0], segment[1]) |
||||
return result |
||||
|
||||
|
||||
class StepDetector: |
||||
def __init__(self, preset): |
||||
self.preset = preset |
||||
self.mean = None |
||||
self.window_size = None |
||||
self.corr_max = None |
||||
self.threshold = None |
||||
self.segments = [] |
||||
|
||||
def fit(self, dataframe, segments, contamination=0.01): |
||||
array = dataframe['value'].as_matrix() |
||||
self.mean = array.mean() |
||||
self.segments = segments |
||||
|
||||
norm_data = (array - self.mean) |
||||
|
||||
self.__optimize(norm_data, segments, contamination) |
||||
|
||||
# print(self.threshold) |
||||
|
||||
# import matplotlib.pyplot as plt |
||||
# fig, ax = plt.subplots(figsize=[18, 16]) |
||||
# ax = fig.add_subplot(2, 1, 1) |
||||
# ax.plot(array) |
||||
# ax = fig.add_subplot(2, 1, 2, sharex=ax) |
||||
# ax.plot(corr_res) |
||||
# plt.show() |
||||
|
||||
# #print(R.size) |
||||
# # Nw = 20 |
||||
# # result = R[Nw,Nw:-1] |
||||
# # result[0] = 0 |
||||
# #ax.plot(result) |
||||
# #print(len(data)) |
||||
# #print(len(R)) |
||||
# |
||||
# print(self.window_size) |
||||
# print(self.threshold) |
||||
|
||||
def predict(self, dataframe): |
||||
array = dataframe['value'].as_matrix() |
||||
|
||||
norm_data = (array - self.mean) |
||||
|
||||
step_size = self.window_size // 2 |
||||
pattern = np.concatenate([[-1] * step_size, [1] * step_size]) |
||||
corr_res = np.correlate(norm_data, pattern, mode='valid') / self.window_size |
||||
corr_res = np.concatenate((np.zeros(step_size), corr_res, np.zeros(step_size))) |
||||
|
||||
corr_res /= self.corr_max |
||||
|
||||
result = self.__predict(corr_res, self.threshold) |
||||
|
||||
# import matplotlib.pyplot as plt |
||||
# fig, ax = plt.subplots(figsize=[18, 16]) |
||||
# ax = fig.add_subplot(2, 1, 1) |
||||
# ax.plot(array[:70000]) |
||||
# ax = fig.add_subplot(2, 1, 2, sharex=ax) |
||||
# ax.plot(corr_res[:70000]) |
||||
# plt.show() |
||||
|
||||
result.sort() |
||||
result = compress_segments(result) |
||||
|
||||
if len(self.segments) > 0: |
||||
result = [segment for segment in result if not is_intersect(segment, self.segments)] |
||||
return result |
||||
|
||||
def __optimize(self, data, segments, contamination): |
||||
window_size = 10 |
||||
mincost = None |
||||
while window_size < 100: |
||||
# print(window_size) |
||||
cost = self.__optimize_threshold(data, window_size, segments, contamination) |
||||
if mincost is None or cost < mincost: |
||||
mincost = cost |
||||
self.window_size = window_size |
||||
window_size = int(window_size * 1.2) |
||||
self.__optimize_threshold(data, self.window_size, segments, contamination) |
||||
|
||||
def __optimize_threshold(self, data, window_size, segments, contamination): |
||||
step_size = window_size // 2 |
||||
pattern = np.concatenate([[-1] * step_size, [1] * step_size]) |
||||
corr_res = np.correlate(data, pattern, mode='same') / window_size |
||||
corr_res = np.concatenate((np.zeros(step_size), corr_res, np.zeros(step_size))) |
||||
self.corr_max = corr_res.max() |
||||
corr_res /= self.corr_max |
||||
N = 20 |
||||
lower = 0. |
||||
upper = 1. |
||||
cost = 0 |
||||
for i in range(0, N): |
||||
self.threshold = 0.5 * (lower + upper) |
||||
result = self.__predict(corr_res, self.threshold) |
||||
|
||||
if len(segments) > 0: |
||||
intersections, labeled = calc_intersections(segments, result) |
||||
good = intersections == labeled |
||||
cost = len(result) |
||||
else: |
||||
total_sum = 0 |
||||
for segment in result: |
||||
total_sum += (segment[1] - segment[0]) |
||||
good = total_sum > len(data) * contamination |
||||
cost = -self.threshold |
||||
|
||||
if good: |
||||
lower = self.threshold |
||||
else: |
||||
upper = self.threshold |
||||
|
||||
return cost |
||||
|
||||
def __predict(self, data, threshold): |
||||
segments = find_segments(data, threshold) |
||||
segments += find_segments(data * -1, threshold) |
||||
#segments -= 1 |
||||
return [(x - 1, y - 1) for (x, y) in segments] |
||||
|
||||
def save(self, model_filename): |
||||
with open(model_filename, 'wb') as file: |
||||
pickle.dump((self.mean, self.window_size, self.corr_max, self.threshold), file) |
||||
|
||||
def load(self, model_filename): |
||||
try: |
||||
with open(model_filename, 'rb') as file: |
||||
self.mean, self.window_size, self.corr_max, self.threshold = pickle.load(file) |
||||
except: |
||||
pass |
@ -0,0 +1,71 @@
|
||||
import pickle |
||||
from tsfresh.transformers.feature_selector import FeatureSelector |
||||
from sklearn.preprocessing import MinMaxScaler |
||||
from sklearn.ensemble import IsolationForest |
||||
import pandas as pd |
||||
|
||||
from sklearn import svm |
||||
|
||||
|
||||
class supervised_algorithm(object): |
||||
frame_size = 16 |
||||
good_features = [ |
||||
#"value__agg_linear_trend__f_agg_\"max\"__chunk_len_5__attr_\"intercept\"", |
||||
# "value__cwt_coefficients__widths_(2, 5, 10, 20)__coeff_12__w_20", |
||||
# "value__cwt_coefficients__widths_(2, 5, 10, 20)__coeff_13__w_5", |
||||
# "value__cwt_coefficients__widths_(2, 5, 10, 20)__coeff_2__w_10", |
||||
# "value__cwt_coefficients__widths_(2, 5, 10, 20)__coeff_2__w_20", |
||||
# "value__cwt_coefficients__widths_(2, 5, 10, 20)__coeff_8__w_20", |
||||
# "value__fft_coefficient__coeff_3__attr_\"abs\"", |
||||
"time_of_day_column_x", |
||||
"time_of_day_column_y", |
||||
"value__abs_energy", |
||||
# "value__absolute_sum_of_changes", |
||||
# "value__sum_of_reoccurring_data_points", |
||||
] |
||||
clf = None |
||||
scaler = None |
||||
|
||||
def __init__(self): |
||||
self.features = [] |
||||
self.col_to_max, self.col_to_min, self.col_to_median = None, None, None |
||||
self.augmented_path = None |
||||
|
||||
def fit(self, dataset, contamination=0.005): |
||||
dataset = dataset[self.good_features] |
||||
dataset = dataset[-100000:] |
||||
|
||||
self.scaler = MinMaxScaler(feature_range=(-1, 1)) |
||||
# self.clf = svm.OneClassSVM(nu=contamination, kernel="rbf", gamma=0.1) |
||||
self.clf = IsolationForest(contamination=contamination) |
||||
|
||||
self.scaler.fit(dataset) |
||||
|
||||
dataset = self.scaler.transform(dataset) |
||||
self.clf.fit(dataset) |
||||
|
||||
def predict(self, dataframe): |
||||
dataset = dataframe[self.good_features] |
||||
dataset = self.scaler.transform(dataset) |
||||
prediction = self.clf.predict(dataset) |
||||
|
||||
# for i in range(len(dataset)): |
||||
# print(str(dataset[i]) + " " + str(prediction[i])) |
||||
|
||||
prediction = [x < 0.0 for x in prediction] |
||||
return pd.Series(prediction, index=dataframe.index) |
||||
|
||||
def save(self, model_filename): |
||||
with open(model_filename, 'wb') as file: |
||||
pickle.dump((self.clf, self.scaler), file) |
||||
|
||||
def load(self, model_filename): |
||||
with open(model_filename, 'rb') as file: |
||||
self.clf, self.scaler = pickle.load(file) |
||||
|
||||
def __select_features(self, x, y): |
||||
# feature_selector = FeatureSelector() |
||||
feature_selector = FeatureSelector() |
||||
|
||||
feature_selector.fit(x, y) |
||||
return feature_selector.relevant_features |
@ -0,0 +1,131 @@
|
||||
from anomaly_model import AnomalyModel |
||||
from pattern_detection_model import PatternDetectionModel |
||||
import queue |
||||
import threading |
||||
import json |
||||
import logging |
||||
import sys |
||||
import traceback |
||||
|
||||
logging.basicConfig(level=logging.DEBUG, |
||||
format='%(asctime)s %(name)-12s %(levelname)-8s %(message)s', |
||||
filename='analytic_toolset.log', |
||||
filemode='a') |
||||
logger = logging.getLogger('analytic_toolset') |
||||
|
||||
|
||||
class worker(object): |
||||
models_cache = {} |
||||
thread = None |
||||
queue = queue.Queue() |
||||
|
||||
def start(self): |
||||
self.thread = threading.Thread(target=self.run) |
||||
self.thread.start() |
||||
|
||||
def stop(self): |
||||
if self.thread: |
||||
self.queue.put(None) |
||||
self.thread.join() |
||||
|
||||
def run(self): |
||||
while True: |
||||
task = self.queue.get() |
||||
if task['type'] == "stop": |
||||
break |
||||
self.do_task(task) |
||||
self.queue.task_done() |
||||
|
||||
def add_task(self, task): |
||||
self.queue.put(task) |
||||
|
||||
def do_task(self, task): |
||||
try: |
||||
type = task['type'] |
||||
anomaly_id = task['anomaly_id'] |
||||
if type == "predict": |
||||
last_prediction_time = task['last_prediction_time'] |
||||
analytics_type = task['analytics_type'] |
||||
preset = None |
||||
if "preset" in task: |
||||
preset = task['preset'] |
||||
result = self.do_predict(anomaly_id, last_prediction_time, analytics_type, preset) |
||||
elif type == "learn": |
||||
segments = task['segments'] |
||||
analytics_type = task['analytics_type'] |
||||
preset = None |
||||
if "preset" in task: |
||||
preset = task['preset'] |
||||
result = self.do_learn(anomaly_id, segments, analytics_type, preset) |
||||
else: |
||||
result = { |
||||
'status': "failed", |
||||
'error': "unknown type " + str(type) |
||||
} |
||||
except Exception as e: |
||||
#traceback.extract_stack() |
||||
error_text = traceback.format_exc() |
||||
logger.error("Exception: '%s'" % error_text) |
||||
result = { |
||||
'task': type, |
||||
'status': "failed", |
||||
'anomaly_id': anomaly_id, |
||||
'error': str(e) |
||||
} |
||||
return result |
||||
|
||||
def do_learn(self, anomaly_id, segments, analytics_type, preset=None): |
||||
model = self.get_model(anomaly_id, analytics_type, preset) |
||||
model.synchronize_data() |
||||
last_prediction_time = model.learn(segments) |
||||
result = self.do_predict(anomaly_id, last_prediction_time, analytics_type, preset) |
||||
result['task'] = 'learn' |
||||
return result |
||||
|
||||
def do_predict(self, anomaly_id, last_prediction_time, analytics_type, preset=None): |
||||
model = self.get_model(anomaly_id, analytics_type, preset) |
||||
model.synchronize_data() |
||||
segments, last_prediction_time = model.predict(last_prediction_time) |
||||
return { |
||||
'task': "predict", |
||||
'status': "success", |
||||
'anomaly_id': anomaly_id, |
||||
'segments': segments, |
||||
'last_prediction_time': last_prediction_time |
||||
} |
||||
|
||||
def get_model(self, anomaly_id, analytics_type, preset=None): |
||||
if anomaly_id not in self.models_cache: |
||||
if analytics_type == "anomalies": |
||||
model = AnomalyModel(anomaly_id) |
||||
elif analytics_type == "patterns": |
||||
model = PatternDetectionModel(anomaly_id, preset) |
||||
self.models_cache[anomaly_id] = model |
||||
return self.models_cache[anomaly_id] |
||||
|
||||
|
||||
if __name__ == "__main__": |
||||
w = worker() |
||||
logger.info("Worker was started") |
||||
while True: |
||||
try: |
||||
text = input("") |
||||
task = json.loads(text) |
||||
logger.info("Received command '%s'" % text) |
||||
if task['type'] == "stop": |
||||
logger.info("Stopping...") |
||||
break |
||||
print(json.dumps({ |
||||
'task': task['type'], |
||||
'anomaly_id': task['anomaly_id'], |
||||
'__task_id': task['__task_id'], |
||||
'status': "in progress" |
||||
})) |
||||
sys.stdout.flush() |
||||
res = w.do_task(task) |
||||
res['__task_id'] = task['__task_id'] |
||||
print(json.dumps(res)) |
||||
sys.stdout.flush() |
||||
except Exception as e: |
||||
logger.error("Exception: '%s'" % str(e)) |
||||
|
Loading…
Reference in new issue