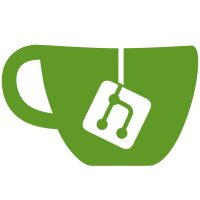
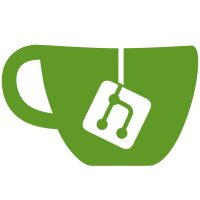
60 changed files with 4 additions and 4509 deletions
@ -0,0 +1,3 @@
|
||||
[submodule "analytics"] |
||||
path = analytics |
||||
url = https://github.com/hastic/analytics |
@ -1,5 +0,0 @@
|
||||
build/ |
||||
dist/ |
||||
*.spec |
||||
__pycache__/ |
||||
test/ |
@ -1,32 +0,0 @@
|
||||
{ |
||||
// Use IntelliSense to learn about possible attributes. |
||||
// Hover to view descriptions of existing attributes. |
||||
// For more information, visit: https://go.microsoft.com/fwlink/?linkid=830387 |
||||
"version": "0.2.0", |
||||
"configurations": [ |
||||
{ |
||||
"name": "Attach (Remote Debug)", |
||||
"type": "python", |
||||
"request": "attach", |
||||
"port": 5679, |
||||
"host": "localhost", |
||||
"pathMappings": [ |
||||
{ |
||||
"localRoot": "${workspaceFolder}", |
||||
"remoteRoot": "/var/www/analytics" |
||||
} |
||||
] |
||||
}, |
||||
{ |
||||
"name": "Python: Current File", |
||||
"type": "python", |
||||
"request": "launch", |
||||
"windows": { |
||||
"program": "${workspaceFolder}\\bin\\server" |
||||
}, |
||||
"linux": { |
||||
"program": "${workspaceFolder}/bin/server" |
||||
} |
||||
} |
||||
] |
||||
} |
@ -1,22 +0,0 @@
|
||||
{ |
||||
"terminal.integrated.shell.windows": "C:\\WINDOWS\\System32\\WindowsPowerShell\\v1.0\\powershell.exe", |
||||
"editor.insertSpaces": true, |
||||
"files.eol": "\n", |
||||
"files.exclude": { |
||||
"**/__pycache__/": true, |
||||
"dist": true, |
||||
"build": true |
||||
}, |
||||
"[python]": { |
||||
"editor.tabSize": 4, |
||||
}, |
||||
"python.envFile": "${workspaceFolder}/.vscode/.env", |
||||
"python.pythonPath": "python", |
||||
"python.linting.enabled": true, |
||||
"python.testing.unittestArgs": [ "-v" ], |
||||
"python.testing.pytestEnabled": false, |
||||
"python.testing.nosetestsEnabled": false, |
||||
"python.testing.unittestEnabled": true, |
||||
"python.linting.pylintEnabled": true, |
||||
"python.jediEnabled": false |
||||
} |
@ -1,27 +0,0 @@
|
||||
# Type hints |
||||
|
||||
Please use: https://www.python.org/dev/peps/pep-0484/ |
||||
|
||||
# Line endings |
||||
|
||||
We use LF everywhere |
||||
|
||||
# Imports |
||||
|
||||
You import local files first, than spesific liba and then standart libs. |
||||
So you import from something very scecific to something very common. |
||||
It allows you to pay attention on most important things from beginning. |
||||
|
||||
``` |
||||
|
||||
from data_provider import DataProvider |
||||
from anomaly_model import AnomalyModel |
||||
from pattern_detection_model import PatternDetectionModel |
||||
|
||||
import numpy as np |
||||
|
||||
from scipy.signal import argrelextrema |
||||
|
||||
import pickle |
||||
|
||||
``` |
@ -1,12 +0,0 @@
|
||||
FROM python:3.6.6 |
||||
|
||||
COPY requirements.txt /requirements.txt |
||||
|
||||
RUN pip install -r /requirements.txt |
||||
|
||||
WORKDIR /var/www/analytics |
||||
|
||||
COPY . /var/www/analytics/ |
||||
|
||||
|
||||
CMD ["python", "-u", "bin/server"] |
@ -1,12 +0,0 @@
|
||||
# Hastic-server-analytics |
||||
|
||||
Python service which gets tasks from [hastic-server-node](https://github.com/hastic/hastic-server/tree/master/server) like |
||||
|
||||
* trains statistical models |
||||
* detect patterns in time series data |
||||
|
||||
## Arhitecture |
||||
|
||||
The service uses [asyncio](https://docs.python.org/3/library/asyncio.html), |
||||
[concurrency](https://docs.python.org/3.6/library/concurrent.futures.html#module-concurrent.futures) and |
||||
[pyzmq](https://pyzmq.readthedocs.io/en/latest/). |
@ -1,39 +0,0 @@
|
||||
""" |
||||
It is the place where we put all classes and types |
||||
common for all analytics code |
||||
|
||||
For example, if you write someting which is used |
||||
in analytic_unit_manager, it should be here. |
||||
|
||||
If you create something spicific which is used only in one place, |
||||
like PatternDetectionCache, then it should not be here. |
||||
""" |
||||
|
||||
import pandas as pd |
||||
from typing import Union, List, Tuple |
||||
|
||||
AnalyticUnitId = str |
||||
|
||||
ModelCache = dict |
||||
|
||||
# TODO: explicit timestamp / value |
||||
TimeSeries = List[Tuple[int, float]] |
||||
|
||||
""" |
||||
Example: |
||||
|
||||
tsis = TimeSeriesIndex(['2017-12-31 16:00:00-08:00', '2017-12-31 17:00:00-08:00', '2017-12-31 18:00:00-08:00']) |
||||
ts = TimeSeries([4, 5, 6], tsis) |
||||
""" |
||||
Timestamp = Union[str, pd.Timestamp] |
||||
|
||||
class TimeSeriesIndex(pd.DatetimeIndex): |
||||
def __new__(cls, *args, **kwargs): |
||||
return pd.DatetimeIndex.__new__(cls, *args, **kwargs) |
||||
|
||||
# TODO: make generic type for values. See List definition for example of generic class |
||||
# TODO: constructor from DataFrame |
||||
# TODO: repleace TimeSeries (above) with this class: rename TimeSeries2 to TimeSeries |
||||
class TimeSeries2(pd.Series): |
||||
def __init__(self, *args, **kwargs): |
||||
super().__init__(*args, **kwargs) |
@ -1,38 +0,0 @@
|
||||
from typing import Optional, List, Dict |
||||
|
||||
from analytic_types.segment import AnomalyDetectorSegment |
||||
from analytic_types.detector import Bound |
||||
|
||||
from utils.meta import JSONClass, SerializableList |
||||
|
||||
@JSONClass |
||||
class AnomalyCache: |
||||
def __init__( |
||||
self, |
||||
alpha: float, |
||||
confidence: float, |
||||
enable_bounds: str, |
||||
seasonality: Optional[int] = None, |
||||
segments: Optional[List[Dict]] = None, |
||||
time_step: Optional[int] = None, |
||||
): |
||||
self.alpha = alpha |
||||
self.confidence = confidence |
||||
self.enable_bounds = enable_bounds |
||||
if seasonality != None and seasonality < 0: |
||||
raise ValueError(f'Can`t create AnomalyCache: got invalid seasonality {seasonality}') |
||||
self.seasonality = seasonality |
||||
self.time_step = time_step |
||||
if segments != None: |
||||
anomaly_segments = map(AnomalyDetectorSegment.from_json, segments) |
||||
self.segments = SerializableList(anomaly_segments) |
||||
else: |
||||
self.segments = [] |
||||
|
||||
def set_segments(self, segments: List[AnomalyDetectorSegment]): |
||||
if len(segments) > 0: |
||||
self.segments = SerializableList(segments) |
||||
|
||||
def get_enabled_bounds(self) -> Bound: |
||||
#TODO: use class with to_json() |
||||
return Bound(self.enable_bounds) |
@ -1,14 +0,0 @@
|
||||
import pandas as pd |
||||
|
||||
|
||||
class DataBucket: |
||||
|
||||
def __init__(self): |
||||
self.data = pd.DataFrame([], columns=['timestamp', 'value']) |
||||
|
||||
def receive_data(self, data: pd.DataFrame): |
||||
self.data = self.data.append(data, ignore_index=True) |
||||
|
||||
def drop_data(self, count: int): |
||||
if count > 0: |
||||
self.data = self.data.iloc[count:] |
@ -1,47 +0,0 @@
|
||||
from analytic_types import ModelCache, TimeSeries |
||||
from analytic_types.segment import Segment |
||||
|
||||
from enum import Enum |
||||
from typing import List, Optional, Tuple |
||||
|
||||
import utils.meta |
||||
|
||||
class Bound(Enum): |
||||
ALL = 'ALL' |
||||
UPPER = 'UPPER' |
||||
LOWER = 'LOWER' |
||||
|
||||
class DetectionResult: |
||||
|
||||
def __init__( |
||||
self, |
||||
cache: Optional[ModelCache] = None, |
||||
segments: Optional[List[Segment]] = None, |
||||
last_detection_time: int = None |
||||
): |
||||
if cache is None: |
||||
cache = {} |
||||
if segments is None: |
||||
segments = [] |
||||
self.cache = cache |
||||
self.segments = segments |
||||
self.last_detection_time = last_detection_time |
||||
|
||||
# TODO: use @utils.meta.JSONClass (now it can't serialize list of objects) |
||||
def to_json(self): |
||||
return { |
||||
'cache': self.cache, |
||||
'segments': list(map(lambda segment: segment.to_json(), self.segments)), |
||||
'lastDetectionTime': self.last_detection_time |
||||
} |
||||
|
||||
@utils.meta.JSONClass |
||||
class ProcessingResult(): |
||||
|
||||
def __init__( |
||||
self, |
||||
lower_bound: Optional[TimeSeries] = None, |
||||
upper_bound: Optional[TimeSeries] = None, |
||||
): |
||||
self.lower_bound = lower_bound |
||||
self.upper_bound = upper_bound |
@ -1,17 +0,0 @@
|
||||
import utils.meta |
||||
|
||||
@utils.meta.JSONClass |
||||
class LearningInfo: |
||||
|
||||
def __init__(self): |
||||
super().__init__() |
||||
self.confidence = [] |
||||
self.patterns_list = [] |
||||
self.pattern_width = [] |
||||
self.pattern_height = [] |
||||
self.pattern_timestamp = [] |
||||
self.segment_center_list = [] |
||||
self.patterns_value = [] |
||||
|
||||
def __str__(self): |
||||
return str(self.to_json()) |
@ -1,57 +0,0 @@
|
||||
from typing import Optional |
||||
|
||||
import utils.meta |
||||
|
||||
@utils.meta.JSONClass |
||||
class Segment: |
||||
''' |
||||
Used for segment manipulation instead of { 'from': ..., 'to': ... } dict |
||||
''' |
||||
|
||||
def __init__( |
||||
self, |
||||
from_timestamp: int, |
||||
to_timestamp: int, |
||||
_id: Optional[str] = None, |
||||
analytic_unit_id: Optional[str] = None, |
||||
labeled: Optional[bool] = None, |
||||
deleted: Optional[bool] = None, |
||||
message: Optional[str] = None |
||||
): |
||||
if to_timestamp < from_timestamp: |
||||
raise ValueError(f'Can`t create segment with to < from: {to_timestamp} < {from_timestamp}') |
||||
self.from_timestamp = from_timestamp |
||||
self.to_timestamp = to_timestamp |
||||
self._id = _id |
||||
self.analytic_unit_id = analytic_unit_id |
||||
self.labeled = labeled |
||||
self.deleted = deleted |
||||
self.message = message |
||||
|
||||
@utils.meta.JSONClass |
||||
class AnomalyDetectorSegment(Segment): |
||||
''' |
||||
Used for segment manipulation instead of { 'from': ..., 'to': ..., 'data': ... } dict |
||||
''' |
||||
|
||||
def __init__( |
||||
self, |
||||
from_timestamp: int, |
||||
to_timestamp: int, |
||||
data = [], |
||||
_id: Optional[str] = None, |
||||
analytic_unit_id: Optional[str] = None, |
||||
labeled: Optional[bool] = None, |
||||
deleted: Optional[bool] = None, |
||||
message: Optional[str] = None |
||||
): |
||||
super().__init__( |
||||
from_timestamp, |
||||
to_timestamp, |
||||
_id, |
||||
analytic_unit_id, |
||||
labeled, |
||||
deleted, |
||||
message |
||||
) |
||||
self.data = data |
@ -1,103 +0,0 @@
|
||||
from typing import Dict |
||||
import logging as log |
||||
import traceback |
||||
from concurrent.futures import Executor, ThreadPoolExecutor |
||||
|
||||
from analytic_unit_worker import AnalyticUnitWorker |
||||
from analytic_types import AnalyticUnitId, ModelCache |
||||
from analytic_types.segment import Segment |
||||
import detectors |
||||
|
||||
|
||||
logger = log.getLogger('AnalyticUnitManager') |
||||
|
||||
|
||||
def get_detector_by_type( |
||||
detector_type: str, analytic_unit_type: str, analytic_unit_id: AnalyticUnitId |
||||
) -> detectors.Detector: |
||||
if detector_type == 'pattern': |
||||
return detectors.PatternDetector(analytic_unit_type, analytic_unit_id) |
||||
elif detector_type == 'threshold': |
||||
return detectors.ThresholdDetector(analytic_unit_id) |
||||
elif detector_type == 'anomaly': |
||||
return detectors.AnomalyDetector(analytic_unit_id) |
||||
|
||||
raise ValueError('Unknown detector type "%s"' % detector_type) |
||||
|
||||
|
||||
class AnalyticUnitManager: |
||||
|
||||
def __init__(self): |
||||
self.analytic_workers: Dict[AnalyticUnitId, AnalyticUnitWorker] = dict() |
||||
self.workers_executor = ThreadPoolExecutor() |
||||
|
||||
def __ensure_worker( |
||||
self, |
||||
analytic_unit_id: AnalyticUnitId, |
||||
detector_type: str, |
||||
analytic_unit_type: str |
||||
) -> AnalyticUnitWorker: |
||||
if analytic_unit_id in self.analytic_workers: |
||||
# TODO: check that type is the same |
||||
return self.analytic_workers[analytic_unit_id] |
||||
detector = get_detector_by_type(detector_type, analytic_unit_type, analytic_unit_id) |
||||
worker = AnalyticUnitWorker(analytic_unit_id, detector, self.workers_executor) |
||||
self.analytic_workers[analytic_unit_id] = worker |
||||
return worker |
||||
|
||||
async def __handle_analytic_task(self, task: object) -> dict: |
||||
""" |
||||
returns payload or None |
||||
""" |
||||
analytic_unit_id: AnalyticUnitId = task['analyticUnitId'] |
||||
log.debug('Analytics get task with type: {} for unit: {}'.format(task['type'], analytic_unit_id)) |
||||
if task['type'] == 'CANCEL': |
||||
if analytic_unit_id in self.analytic_workers: |
||||
self.analytic_workers[analytic_unit_id].cancel() |
||||
return |
||||
|
||||
payload = task['payload'] |
||||
worker = self.__ensure_worker(analytic_unit_id, payload['detector'], payload['analyticUnitType']) |
||||
data = payload.get('data') |
||||
if task['type'] == 'PUSH': |
||||
# TODO: do it a better way |
||||
res = await worker.consume_data(data, payload['cache']) |
||||
if res: |
||||
res.update({ 'analyticUnitId': analytic_unit_id }) |
||||
return res |
||||
elif task['type'] == 'LEARN': |
||||
if 'segments' in payload: |
||||
segments = payload['segments'] |
||||
segments = [Segment.from_json(segment) for segment in segments] |
||||
return await worker.do_train(segments, data, payload['cache']) |
||||
elif 'threshold' in payload: |
||||
return await worker.do_train(payload['threshold'], data, payload['cache']) |
||||
elif 'anomaly' in payload: |
||||
return await worker.do_train(payload['anomaly'], data, payload['cache']) |
||||
else: |
||||
raise ValueError('No segments or threshold in LEARN payload') |
||||
elif task['type'] == 'DETECT': |
||||
return await worker.do_detect(data, payload['cache']) |
||||
elif task['type'] == 'PROCESS': |
||||
return await worker.process_data(data, payload['cache']) |
||||
|
||||
raise ValueError('Unknown task type "%s"' % task['type']) |
||||
|
||||
async def handle_analytic_task(self, task: object): |
||||
try: |
||||
log.debug('Start handle_analytic_task with analytic unit: {}'.format(task['analyticUnitId'])) |
||||
result_payload = await self.__handle_analytic_task(task) |
||||
result_message = { |
||||
'status': 'SUCCESS', |
||||
'payload': result_payload |
||||
} |
||||
log.debug('End correctly handle_analytic_task with anatytic unit: {}'.format(task['analyticUnitId'])) |
||||
return result_message |
||||
except Exception as e: |
||||
error_text = traceback.format_exc() |
||||
logger.error("handle_analytic_task Exception: '%s'" % error_text) |
||||
# TODO: move result to a class which renders to json for messaging to analytics |
||||
return { |
||||
'status': 'FAILED', |
||||
'error': repr(e) |
||||
} |
@ -1,116 +0,0 @@
|
||||
import config |
||||
import detectors |
||||
import logging |
||||
import pandas as pd |
||||
from typing import Optional, Union, Generator, List, Tuple |
||||
import concurrent.futures |
||||
import asyncio |
||||
import utils |
||||
from utils import get_intersected_chunks, get_chunks, prepare_data |
||||
|
||||
from analytic_types import ModelCache, TimeSeries |
||||
from analytic_types.detector import DetectionResult |
||||
|
||||
logger = logging.getLogger('AnalyticUnitWorker') |
||||
|
||||
|
||||
class AnalyticUnitWorker: |
||||
|
||||
CHUNK_WINDOW_SIZE_FACTOR = 100 |
||||
CHUNK_INTERSECTION_FACTOR = 2 |
||||
|
||||
assert CHUNK_WINDOW_SIZE_FACTOR > CHUNK_INTERSECTION_FACTOR, \ |
||||
'CHUNK_INTERSECTION_FACTOR should be less than CHUNK_WINDOW_SIZE_FACTOR' |
||||
|
||||
def __init__(self, analytic_unit_id: str, detector: detectors.Detector, executor: concurrent.futures.Executor): |
||||
self.analytic_unit_id = analytic_unit_id |
||||
self._detector = detector |
||||
self._executor: concurrent.futures.Executor = executor |
||||
self._training_future: asyncio.Future = None |
||||
|
||||
async def do_train( |
||||
self, payload: Union[list, dict], data: TimeSeries, cache: Optional[ModelCache] |
||||
) -> Optional[ModelCache]: |
||||
|
||||
dataframe = prepare_data(data) |
||||
|
||||
cfuture: concurrent.futures.Future = self._executor.submit( |
||||
self._detector.train, dataframe, payload, cache |
||||
) |
||||
self._training_future = asyncio.wrap_future(cfuture) |
||||
try: |
||||
new_cache: ModelCache = await asyncio.wait_for(self._training_future, timeout = config.LEARNING_TIMEOUT) |
||||
return new_cache |
||||
except asyncio.CancelledError: |
||||
return None |
||||
except asyncio.TimeoutError: |
||||
raise Exception('Timeout ({}s) exceeded while learning'.format(config.LEARNING_TIMEOUT)) |
||||
|
||||
async def do_detect(self, data: pd.DataFrame, cache: Optional[ModelCache]) -> DetectionResult: |
||||
|
||||
window_size = self._detector.get_window_size(cache) |
||||
chunk_size = window_size * self.CHUNK_WINDOW_SIZE_FACTOR |
||||
chunk_intersection = window_size * self.CHUNK_INTERSECTION_FACTOR |
||||
|
||||
detections: List[DetectionResult] = [] |
||||
chunks = [] |
||||
# XXX: get_chunks(data, chunk_size) == get_intersected_chunks(data, 0, chunk_size) |
||||
if self._detector.is_detection_intersected(): |
||||
chunks = get_intersected_chunks(data, chunk_intersection, chunk_size) |
||||
else: |
||||
chunks = get_chunks(data, chunk_size) |
||||
|
||||
for chunk in chunks: |
||||
await asyncio.sleep(0) |
||||
chunk_dataframe = prepare_data(chunk) |
||||
detected: DetectionResult = self._detector.detect(chunk_dataframe, cache) |
||||
detections.append(detected) |
||||
|
||||
if len(detections) == 0: |
||||
raise RuntimeError(f'do_detect for {self.analytic_unit_id} got empty detection results') |
||||
|
||||
detection_result = self._detector.concat_detection_results(detections) |
||||
return detection_result.to_json() |
||||
|
||||
def cancel(self): |
||||
if self._training_future is not None: |
||||
self._training_future.cancel() |
||||
|
||||
async def consume_data(self, data: TimeSeries, cache: Optional[ModelCache]) -> Optional[dict]: |
||||
window_size = self._detector.get_window_size(cache) |
||||
|
||||
detections: List[DetectionResult] = [] |
||||
|
||||
for chunk in get_chunks(data, window_size * self.CHUNK_WINDOW_SIZE_FACTOR): |
||||
await asyncio.sleep(0) |
||||
chunk_dataframe = prepare_data(chunk) |
||||
detected = self._detector.consume_data(chunk_dataframe, cache) |
||||
if detected is not None: |
||||
detections.append(detected) |
||||
|
||||
if len(detections) == 0: |
||||
return None |
||||
else: |
||||
detection_result = self._detector.concat_detection_results(detections) |
||||
return detection_result.to_json() |
||||
|
||||
async def process_data(self, data: TimeSeries, cache: ModelCache) -> dict: |
||||
assert isinstance(self._detector, detectors.ProcessingDetector), \ |
||||
f'{self.analytic_unit_id} detector is not ProcessingDetector, can`t process data' |
||||
assert cache is not None, f'{self.analytic_unit_id} got empty cache for processing data' |
||||
|
||||
processed_chunks = [] |
||||
window_size = self._detector.get_window_size(cache) |
||||
for chunk in get_chunks(data, window_size * self.CHUNK_WINDOW_SIZE_FACTOR): |
||||
await asyncio.sleep(0) |
||||
chunk_dataframe = prepare_data(chunk) |
||||
processed = self._detector.process_data(chunk_dataframe, cache) |
||||
if processed is not None: |
||||
processed_chunks.append(processed) |
||||
|
||||
if len(processed_chunks) == 0: |
||||
raise RuntimeError(f'process_data for {self.analytic_unit_id} got empty processing results') |
||||
|
||||
# TODO: maybe we should process all chunks inside of detector? |
||||
result = self._detector.concat_processing_results(processed_chunks) |
||||
return result.to_json() |
@ -1,30 +0,0 @@
|
||||
import os |
||||
import json |
||||
|
||||
|
||||
PARENT_FOLDER = os.path.dirname(os.path.dirname(os.path.dirname(os.path.realpath(__file__)))) |
||||
CONFIG_FILE = os.path.join(PARENT_FOLDER, 'config.json') |
||||
|
||||
|
||||
config_exists = os.path.isfile(CONFIG_FILE) |
||||
if config_exists: |
||||
with open(CONFIG_FILE) as f: |
||||
config = json.load(f) |
||||
else: |
||||
print('Config file %s doesn`t exist, using defaults' % CONFIG_FILE) |
||||
|
||||
|
||||
def get_config_field(field: str, default_val = None): |
||||
if field in os.environ: |
||||
return os.environ[field] |
||||
|
||||
if config_exists and field in config and config[field] != '': |
||||
return config[field] |
||||
|
||||
if default_val is not None: |
||||
return default_val |
||||
|
||||
raise Exception('Please configure {}'.format(field)) |
||||
|
||||
HASTIC_SERVER_URL = get_config_field('HASTIC_SERVER_URL', 'ws://localhost:8002') |
||||
LEARNING_TIMEOUT = get_config_field('LEARNING_TIMEOUT', 120) |
@ -1,4 +0,0 @@
|
||||
from detectors.detector import Detector, ProcessingDetector |
||||
from detectors.pattern_detector import PatternDetector |
||||
from detectors.threshold_detector import ThresholdDetector |
||||
from detectors.anomaly_detector import AnomalyDetector |
@ -1,277 +0,0 @@
|
||||
from enum import Enum |
||||
import logging |
||||
import numpy as np |
||||
import pandas as pd |
||||
import math |
||||
from typing import Optional, Union, List, Tuple, Generator |
||||
import operator |
||||
|
||||
from analytic_types import AnalyticUnitId, ModelCache |
||||
from analytic_types.detector import DetectionResult, ProcessingResult, Bound |
||||
from analytic_types.data_bucket import DataBucket |
||||
from analytic_types.segment import Segment, AnomalyDetectorSegment |
||||
from analytic_types.cache import AnomalyCache |
||||
from detectors import Detector, ProcessingDetector |
||||
import utils |
||||
|
||||
MAX_DEPENDENCY_LEVEL = 100 |
||||
MIN_DEPENDENCY_FACTOR = 0.1 |
||||
BASIC_ALPHA = 0.5 |
||||
logger = logging.getLogger('ANOMALY_DETECTOR') |
||||
|
||||
|
||||
class AnomalyDetector(ProcessingDetector): |
||||
|
||||
def __init__(self, analytic_unit_id: AnalyticUnitId): |
||||
super().__init__(analytic_unit_id) |
||||
self.bucket = DataBucket() |
||||
|
||||
def train(self, dataframe: pd.DataFrame, payload: Union[list, dict], cache: Optional[ModelCache]) -> ModelCache: |
||||
cache = AnomalyCache.from_json(payload) |
||||
cache.time_step = utils.find_interval(dataframe) |
||||
segments = cache.segments |
||||
|
||||
if len(segments) > 0: |
||||
seasonality = cache.seasonality |
||||
prepared_segments = [] |
||||
|
||||
for segment in segments: |
||||
segment_len = (int(segment.to_timestamp) - int(segment.from_timestamp)) |
||||
assert segment_len <= seasonality, \ |
||||
f'seasonality {seasonality} must be greater than segment length {segment_len}' |
||||
|
||||
from_index = utils.timestamp_to_index(dataframe, pd.to_datetime(segment.from_timestamp, unit='ms')) |
||||
to_index = utils.timestamp_to_index(dataframe, pd.to_datetime(segment.to_timestamp, unit='ms')) |
||||
segment_data = dataframe[from_index : to_index] |
||||
prepared_segments.append( |
||||
AnomalyDetectorSegment( |
||||
segment.from_timestamp, |
||||
segment.to_timestamp, |
||||
segment_data.value.tolist() |
||||
) |
||||
) |
||||
cache.set_segments(prepared_segments) |
||||
|
||||
return { |
||||
'cache': cache.to_json() |
||||
} |
||||
|
||||
# TODO: ModelCache -> DetectorState |
||||
def detect(self, dataframe: pd.DataFrame, cache: Optional[ModelCache]) -> DetectionResult: |
||||
if cache == None: |
||||
raise f'Analytic unit {self.analytic_unit_id} got empty cache' |
||||
data = dataframe['value'] |
||||
|
||||
cache = AnomalyCache.from_json(cache) |
||||
segments = cache.segments |
||||
enabled_bounds = cache.get_enabled_bounds() |
||||
|
||||
smoothed_data = utils.exponential_smoothing(data, cache.alpha) |
||||
|
||||
lower_bound = smoothed_data - cache.confidence |
||||
upper_bound = smoothed_data + cache.confidence |
||||
|
||||
if len(segments) > 0: |
||||
data_start_time = utils.convert_pd_timestamp_to_ms(dataframe['timestamp'][0]) |
||||
|
||||
for segment in segments: |
||||
seasonality_index = cache.seasonality // cache.time_step |
||||
seasonality_offset = self.get_seasonality_offset( |
||||
segment.from_timestamp, |
||||
cache.seasonality, |
||||
data_start_time, |
||||
cache.time_step |
||||
) |
||||
segment_data = pd.Series(segment.data) |
||||
|
||||
lower_bound = self.add_season_to_data(lower_bound, segment_data, seasonality_offset, seasonality_index, Bound.LOWER) |
||||
upper_bound = self.add_season_to_data(upper_bound, segment_data, seasonality_offset, seasonality_index, Bound.UPPER) |
||||
|
||||
detected_segments = list(self.detections_generator(dataframe, upper_bound, lower_bound, enabled_bounds)) |
||||
|
||||
last_dataframe_time = dataframe.iloc[-1]['timestamp'] |
||||
last_detection_time = utils.convert_pd_timestamp_to_ms(last_dataframe_time) |
||||
|
||||
return DetectionResult(cache.to_json(), detected_segments, last_detection_time) |
||||
|
||||
def consume_data(self, data: pd.DataFrame, cache: Optional[ModelCache]) -> Optional[DetectionResult]: |
||||
if cache is None: |
||||
msg = f'consume_data got invalid cache {cache} for task {self.analytic_unit_id}' |
||||
logging.debug(msg) |
||||
raise ValueError(msg) |
||||
|
||||
data_without_nan = data.dropna() |
||||
|
||||
if len(data_without_nan) == 0: |
||||
return None |
||||
|
||||
self.bucket.receive_data(data_without_nan) |
||||
|
||||
if len(self.bucket.data) >= self.get_window_size(cache): |
||||
return self.detect(self.bucket.data, cache) |
||||
|
||||
return None |
||||
|
||||
def is_detection_intersected(self) -> bool: |
||||
return False |
||||
|
||||
def get_window_size(self, cache: Optional[ModelCache]) -> int: |
||||
''' |
||||
get the number of values that will affect the next value |
||||
''' |
||||
|
||||
if cache is None: |
||||
raise ValueError('anomaly detector got None cache') |
||||
cache = AnomalyCache.from_json(cache) |
||||
|
||||
for level in range(1, MAX_DEPENDENCY_LEVEL): |
||||
if (1 - cache.alpha) ** level < MIN_DEPENDENCY_FACTOR: |
||||
break |
||||
|
||||
seasonality = 0 |
||||
if len(cache.segments) > 0: |
||||
seasonality = cache.seasonality // cache.time_step |
||||
return max(level, seasonality) |
||||
|
||||
def concat_detection_results(self, detections: List[DetectionResult]) -> DetectionResult: |
||||
result = DetectionResult() |
||||
time_step = detections[0].cache['timeStep'] |
||||
for detection in detections: |
||||
result.segments.extend(detection.segments) |
||||
result.last_detection_time = detection.last_detection_time |
||||
result.cache = detection.cache |
||||
result.segments = utils.merge_intersecting_segments(result.segments, time_step) |
||||
return result |
||||
|
||||
# TODO: remove duplication with detect() |
||||
def process_data(self, dataframe: pd.DataFrame, cache: ModelCache) -> ProcessingResult: |
||||
cache = AnomalyCache.from_json(cache) |
||||
segments = cache.segments |
||||
enabled_bounds = cache.get_enabled_bounds() |
||||
|
||||
# TODO: exponential_smoothing should return dataframe with related timestamps |
||||
smoothed_data = utils.exponential_smoothing(dataframe['value'], cache.alpha) |
||||
|
||||
lower_bound = smoothed_data - cache.confidence |
||||
upper_bound = smoothed_data + cache.confidence |
||||
|
||||
if len(segments) > 0: |
||||
data_start_time = utils.convert_pd_timestamp_to_ms(dataframe['timestamp'][0]) |
||||
|
||||
for segment in segments: |
||||
seasonality_index = cache.seasonality // cache.time_step |
||||
# TODO: move it to utils and add tests |
||||
seasonality_offset = self.get_seasonality_offset( |
||||
segment.from_timestamp, |
||||
cache.seasonality, |
||||
data_start_time, |
||||
cache.time_step |
||||
) |
||||
segment_data = pd.Series(segment.data) |
||||
|
||||
lower_bound = self.add_season_to_data(lower_bound, segment_data, seasonality_offset, seasonality_index, Bound.LOWER) |
||||
upper_bound = self.add_season_to_data(upper_bound, segment_data, seasonality_offset, seasonality_index, Bound.UPPER) |
||||
|
||||
# TODO: support multiple segments |
||||
|
||||
timestamps = utils.convert_series_to_timestamp_list(dataframe.timestamp) |
||||
lower_bound_timeseries = list(zip(timestamps, lower_bound.values.tolist())) |
||||
upper_bound_timeseries = list(zip(timestamps, upper_bound.values.tolist())) |
||||
|
||||
if enabled_bounds == Bound.ALL: |
||||
return ProcessingResult(lower_bound_timeseries, upper_bound_timeseries) |
||||
elif enabled_bounds == Bound.UPPER: |
||||
return ProcessingResult(upper_bound = upper_bound_timeseries) |
||||
elif enabled_bounds == Bound.LOWER: |
||||
return ProcessingResult(lower_bound = lower_bound_timeseries) |
||||
|
||||
def add_season_to_data(self, data: pd.Series, segment: pd.Series, offset: int, seasonality: int, bound_type: Bound) -> pd.Series: |
||||
#data - smoothed data to which seasonality will be added |
||||
#if addition == True -> segment is added |
||||
#if addition == False -> segment is subtracted |
||||
len_smoothed_data = len(data) |
||||
for idx, _ in enumerate(data): |
||||
if idx - offset < 0: |
||||
#TODO: add seasonality for non empty parts |
||||
continue |
||||
if (idx - offset) % seasonality == 0: |
||||
if bound_type == Bound.UPPER: |
||||
upper_segment_bound = self.get_segment_bound(segment, Bound.UPPER) |
||||
data = data.add(pd.Series(upper_segment_bound.values, index = segment.index + idx), fill_value = 0) |
||||
elif bound_type == Bound.LOWER: |
||||
lower_segment_bound = self.get_segment_bound(segment, Bound.LOWER) |
||||
data = data.add(pd.Series(lower_segment_bound.values * -1, index = segment.index + idx), fill_value = 0) |
||||
else: |
||||
raise ValueError(f'unknown bound type: {bound_type.value}') |
||||
|
||||
return data[:len_smoothed_data] |
||||
|
||||
def get_segment_bound(self, segment: pd.Series, bound: Bound) -> pd.Series: |
||||
''' |
||||
segment is divided by the median to determine its top or bottom part |
||||
the part is smoothed and raised above the segment or put down below the segment |
||||
''' |
||||
if len(segment) < 2: |
||||
return segment |
||||
comparison_operator = operator.gt if bound == Bound.UPPER else operator.le |
||||
segment = segment - segment.min() |
||||
segment_median = segment.median() |
||||
part = [val if comparison_operator(val, segment_median) else segment_median for val in segment.values] |
||||
part = pd.Series(part, index = segment.index) |
||||
smoothed_part = utils.exponential_smoothing(part, BASIC_ALPHA) |
||||
difference = [abs(x - y) for x, y in zip(part, smoothed_part)] |
||||
max_diff = max(difference) |
||||
bound = [val + max_diff for val in smoothed_part.values] |
||||
bound = pd.Series(bound, index = segment.index) |
||||
return bound |
||||
|
||||
def get_seasonality_offset(self, from_timestamp: int, seasonality: int, data_start_time: int, time_step: int) -> int: |
||||
season_count = math.ceil(abs(from_timestamp - data_start_time) / seasonality) |
||||
start_seasonal_segment = from_timestamp + seasonality * season_count |
||||
seasonality_time_offset = abs(start_seasonal_segment - data_start_time) % seasonality |
||||
seasonality_offset = math.ceil(seasonality_time_offset / time_step) |
||||
return seasonality_offset |
||||
|
||||
def detections_generator( |
||||
self, |
||||
dataframe: pd.DataFrame, |
||||
upper_bound: pd.DataFrame, |
||||
lower_bound: pd.DataFrame, |
||||
enabled_bounds: Bound |
||||
) -> Generator[Segment, None, Segment]: |
||||
in_segment = False |
||||
segment_start = 0 |
||||
bound: Bound = None |
||||
for idx, val in enumerate(dataframe['value'].values): |
||||
if val > upper_bound.values[idx]: |
||||
if enabled_bounds == Bound.UPPER or enabled_bounds == Bound.ALL: |
||||
if not in_segment: |
||||
in_segment = True |
||||
segment_start = dataframe['timestamp'][idx] |
||||
bound = Bound.UPPER |
||||
continue |
||||
|
||||
if val < lower_bound.values[idx]: |
||||
if enabled_bounds == Bound.LOWER or enabled_bounds == Bound.ALL: |
||||
if not in_segment: |
||||
in_segment = True |
||||
segment_start = dataframe['timestamp'][idx] |
||||
bound = Bound.LOWER |
||||
continue |
||||
|
||||
if in_segment: |
||||
segment_end = dataframe['timestamp'][idx - 1] |
||||
yield Segment( |
||||
utils.convert_pd_timestamp_to_ms(segment_start), |
||||
utils.convert_pd_timestamp_to_ms(segment_end), |
||||
message=f'{val} out of {str(bound.value)} bound' |
||||
) |
||||
in_segment = False |
||||
else: |
||||
if in_segment: |
||||
segment_end = dataframe['timestamp'][idx] |
||||
return Segment( |
||||
utils.convert_pd_timestamp_to_ms(segment_start), |
||||
utils.convert_pd_timestamp_to_ms(segment_end), |
||||
message=f'{val} out of {str(bound.value)} bound' |
||||
) |
@ -1,80 +0,0 @@
|
||||
from abc import ABC, abstractmethod |
||||
from pandas import DataFrame |
||||
from typing import Optional, Union, List |
||||
|
||||
from analytic_types import ModelCache, TimeSeries, AnalyticUnitId |
||||
from analytic_types.detector import DetectionResult, ProcessingResult |
||||
from analytic_types.segment import Segment |
||||
|
||||
|
||||
class Detector(ABC): |
||||
|
||||
def __init__(self, analytic_unit_id: AnalyticUnitId): |
||||
self.analytic_unit_id = analytic_unit_id |
||||
|
||||
@abstractmethod |
||||
def train(self, dataframe: DataFrame, payload: Union[list, dict], cache: Optional[ModelCache]) -> ModelCache: |
||||
""" |
||||
Should be thread-safe to other detectors' train method |
||||
""" |
||||
pass |
||||
|
||||
@abstractmethod |
||||
def detect(self, dataframe: DataFrame, cache: Optional[ModelCache]) -> DetectionResult: |
||||
pass |
||||
|
||||
@abstractmethod |
||||
def consume_data(self, data: DataFrame, cache: Optional[ModelCache]) -> Optional[DetectionResult]: |
||||
pass |
||||
|
||||
@abstractmethod |
||||
def get_window_size(self, cache: Optional[ModelCache]) -> int: |
||||
pass |
||||
|
||||
def is_detection_intersected(self) -> bool: |
||||
return True |
||||
|
||||
def concat_detection_results(self, detections: List[DetectionResult]) -> DetectionResult: |
||||
result = DetectionResult() |
||||
for detection in detections: |
||||
result.segments.extend(detection.segments) |
||||
result.last_detection_time = detection.last_detection_time |
||||
result.cache = detection.cache |
||||
return result |
||||
|
||||
def get_value_from_cache(self, cache: ModelCache, key: str, required = False): |
||||
value = cache.get(key) |
||||
if value == None and required: |
||||
raise ValueError(f'Missing required "{key}" field in cache for analytic unit {self.analytic_unit_id}') |
||||
return value |
||||
|
||||
|
||||
class ProcessingDetector(Detector): |
||||
|
||||
@abstractmethod |
||||
def process_data(self, data: TimeSeries, cache: Optional[ModelCache]) -> ProcessingResult: |
||||
''' |
||||
Data processing to receive additional time series that represents detector's settings |
||||
''' |
||||
pass |
||||
|
||||
def concat_processing_results(self, processing_results: List[ProcessingResult]) -> Optional[ProcessingResult]: |
||||
''' |
||||
Concatenate sequential ProcessingResults that received via |
||||
splitting dataset to chunks in analytic worker |
||||
''' |
||||
|
||||
if len(processing_results) == 0: |
||||
return None |
||||
|
||||
united_result = ProcessingResult() |
||||
for result in processing_results: |
||||
if result.lower_bound is not None: |
||||
if united_result.lower_bound is None: united_result.lower_bound = [] |
||||
united_result.lower_bound.extend(result.lower_bound) |
||||
|
||||
if result.upper_bound is not None: |
||||
if united_result.upper_bound is None: united_result.upper_bound = [] |
||||
united_result.upper_bound.extend(result.upper_bound) |
||||
|
||||
return united_result |
@ -1,147 +0,0 @@
|
||||
import models |
||||
|
||||
import asyncio |
||||
import logging |
||||
import config |
||||
|
||||
import pandas as pd |
||||
from typing import Optional, Generator, List |
||||
|
||||
from detectors import Detector |
||||
from analytic_types.data_bucket import DataBucket |
||||
from utils import convert_pd_timestamp_to_ms |
||||
from analytic_types import AnalyticUnitId, ModelCache |
||||
from analytic_types.detector import DetectionResult |
||||
from analytic_types.segment import Segment |
||||
import utils |
||||
|
||||
logger = logging.getLogger('PATTERN_DETECTOR') |
||||
|
||||
|
||||
def resolve_model_by_pattern(pattern: str) -> models.Model: |
||||
if pattern == 'GENERAL': |
||||
return models.GeneralModel() |
||||
if pattern == 'PEAK': |
||||
return models.PeakModel() |
||||
if pattern == 'TROUGH': |
||||
return models.TroughModel() |
||||
if pattern == 'DROP': |
||||
return models.DropModel() |
||||
if pattern == 'JUMP': |
||||
return models.JumpModel() |
||||
if pattern == 'CUSTOM': |
||||
return models.CustomModel() |
||||
raise ValueError('Unknown pattern "%s"' % pattern) |
||||
|
||||
|
||||
class PatternDetector(Detector): |
||||
|
||||
MIN_BUCKET_SIZE = 150 |
||||
BUCKET_WINDOW_SIZE_FACTOR = 5 |
||||
DEFAULT_WINDOW_SIZE = 1 |
||||
|
||||
def __init__(self, pattern_type: str, analytic_unit_id: AnalyticUnitId): |
||||
super().__init__(analytic_unit_id) |
||||
self.pattern_type = pattern_type |
||||
self.model = resolve_model_by_pattern(self.pattern_type) |
||||
self.bucket = DataBucket() |
||||
|
||||
def train(self, dataframe: pd.DataFrame, segments: List[Segment], cache: Optional[ModelCache]) -> ModelCache: |
||||
# TODO: pass only part of dataframe that has segments |
||||
|
||||
if self.contains_labeled_segments(segments) == False: |
||||
msg = f'{self.analytic_unit_id} has no positive labeled segments. Pattern detector needs at least 1 positive labeled segment' |
||||
logger.error(msg) |
||||
raise ValueError(msg) |
||||
|
||||
self.model.state: models.ModelState = self.model.get_state(cache) |
||||
new_cache: models.ModelState = self.model.fit(dataframe, segments, self.analytic_unit_id) |
||||
|
||||
# time step is optional |
||||
if len(dataframe) > 1: |
||||
new_cache.time_step = utils.find_interval(dataframe) |
||||
|
||||
new_cache = new_cache.to_json() |
||||
if len(new_cache) == 0: |
||||
logging.warning('new_cache is empty with data: {}, segments: {}, cache: {}, analytic unit: {}'.format(dataframe, segments, cache, self.analytic_unit_id)) |
||||
return { |
||||
'cache': new_cache |
||||
} |
||||
|
||||
def detect(self, dataframe: pd.DataFrame, cache: Optional[ModelCache]) -> DetectionResult: |
||||
logger.debug('Unit {} got {} data points for detection'.format(self.analytic_unit_id, len(dataframe))) |
||||
# TODO: split and sleep (https://github.com/hastic/hastic-server/pull/124#discussion_r214085643) |
||||
|
||||
if cache is None: |
||||
msg = f'{self.analytic_unit_id} detection got invalid cache, skip detection' |
||||
logger.error(msg) |
||||
raise ValueError(msg) |
||||
|
||||
self.model.state = self.model.get_state(cache) |
||||
window_size = self.model.state.window_size |
||||
|
||||
if window_size is None: |
||||
message = '{} got cache without window_size for detection'.format(self.analytic_unit_id) |
||||
logger.error(message) |
||||
raise ValueError(message) |
||||
|
||||
if len(dataframe) < window_size * 2: |
||||
message = f'{self.analytic_unit_id} skip detection: dataset length {len(dataframe)} points less than minimal length {window_size * 2} points' |
||||
logger.error(message) |
||||
raise ValueError(message) |
||||
|
||||
detected = self.model.detect(dataframe, self.analytic_unit_id) |
||||
|
||||
segments = [Segment(segment[0], segment[1]) for segment in detected['segments']] |
||||
new_cache = detected['cache'].to_json() |
||||
last_dataframe_time = dataframe.iloc[-1]['timestamp'] |
||||
last_detection_time = convert_pd_timestamp_to_ms(last_dataframe_time) |
||||
return DetectionResult(new_cache, segments, last_detection_time) |
||||
|
||||
def consume_data(self, data: pd.DataFrame, cache: Optional[ModelCache]) -> Optional[DetectionResult]: |
||||
logging.debug('Start consume_data for analytic unit {}'.format(self.analytic_unit_id)) |
||||
|
||||
if cache is None: |
||||
logging.debug(f'consume_data get invalid cache {cache} for task {self.analytic_unit_id}, skip') |
||||
return None |
||||
|
||||
data_without_nan = data.dropna() |
||||
|
||||
if len(data_without_nan) == 0: |
||||
return None |
||||
|
||||
self.bucket.receive_data(data_without_nan) |
||||
|
||||
# TODO: use ModelState |
||||
window_size = cache['windowSize'] |
||||
|
||||
bucket_len = len(self.bucket.data) |
||||
if bucket_len < window_size * 2: |
||||
msg = f'{self.analytic_unit_id} bucket data {bucket_len} less than two window size {window_size * 2}, skip run detection from consume_data' |
||||
logger.debug(msg) |
||||
return None |
||||
|
||||
res = self.detect(self.bucket.data, cache) |
||||
|
||||
bucket_size = max(window_size * self.BUCKET_WINDOW_SIZE_FACTOR, self.MIN_BUCKET_SIZE) |
||||
if bucket_len > bucket_size: |
||||
excess_data = bucket_len - bucket_size |
||||
self.bucket.drop_data(excess_data) |
||||
|
||||
logging.debug('End consume_data for analytic unit: {} with res: {}'.format(self.analytic_unit_id, str(res.to_json()))) |
||||
|
||||
if res: |
||||
return res |
||||
else: |
||||
return None |
||||
|
||||
def get_window_size(self, cache: Optional[ModelCache]) -> int: |
||||
if cache is None: return self.DEFAULT_WINDOW_SIZE |
||||
# TODO: windowSize -> window_size |
||||
return cache.get('windowSize', self.DEFAULT_WINDOW_SIZE) |
||||
|
||||
def contains_labeled_segments(self, segments: List[Segment]) -> bool: |
||||
for segment in segments: |
||||
if segment.labeled == True: |
||||
return True |
||||
return False |
@ -1,111 +0,0 @@
|
||||
import logging as log |
||||
|
||||
import operator |
||||
import pandas as pd |
||||
import numpy as np |
||||
from typing import Optional, List |
||||
|
||||
from analytic_types import ModelCache, AnalyticUnitId |
||||
from analytic_types.detector import DetectionResult, ProcessingResult |
||||
from analytic_types.segment import Segment |
||||
from detectors import ProcessingDetector |
||||
from time import time |
||||
import utils |
||||
|
||||
|
||||
logger = log.getLogger('THRESHOLD_DETECTOR') |
||||
|
||||
|
||||
class ThresholdDetector(ProcessingDetector): |
||||
|
||||
WINDOW_SIZE = 3 |
||||
|
||||
def __init__(self, analytic_unit_id: AnalyticUnitId): |
||||
super().__init__(analytic_unit_id) |
||||
|
||||
def train(self, dataframe: pd.DataFrame, threshold: dict, cache: Optional[ModelCache]) -> ModelCache: |
||||
time_step = utils.find_interval(dataframe) |
||||
return { |
||||
'cache': { |
||||
'value': threshold['value'], |
||||
'condition': threshold['condition'], |
||||
'timeStep': time_step |
||||
} |
||||
} |
||||
|
||||
def detect(self, dataframe: pd.DataFrame, cache: ModelCache) -> DetectionResult: |
||||
if cache is None or cache == {}: |
||||
raise ValueError('Threshold detector error: cannot detect before learning') |
||||
if len(dataframe) == 0: |
||||
return None |
||||
|
||||
value = cache['value'] |
||||
condition = cache['condition'] |
||||
|
||||
segments = [] |
||||
for index, row in dataframe.iterrows(): |
||||
current_value = row['value'] |
||||
current_timestamp = utils.convert_pd_timestamp_to_ms(row['timestamp']) |
||||
segment = Segment(current_timestamp, current_timestamp) |
||||
# TODO: merge segments |
||||
if pd.isnull(current_value): |
||||
if condition == 'NO_DATA': |
||||
segment.message = 'NO_DATA detected' |
||||
segments.append(segment) |
||||
continue |
||||
|
||||
comparators = { |
||||
'>': operator.gt, |
||||
'<': operator.lt, |
||||
'=': operator.eq, |
||||
'>=': operator.ge, |
||||
'<=': operator.le |
||||
} |
||||
|
||||
assert condition in comparators.keys(), f'condition {condition} not allowed' |
||||
|
||||
if comparators[condition](current_value, value): |
||||
segment.message = f"{current_value} {condition} threshold's value {value}" |
||||
segments.append(segment) |
||||
|
||||
last_entry = dataframe.iloc[-1] |
||||
last_detection_time = utils.convert_pd_timestamp_to_ms(last_entry['timestamp']) |
||||
return DetectionResult(cache, segments, last_detection_time) |
||||
|
||||
|
||||
def consume_data(self, data: pd.DataFrame, cache: Optional[ModelCache]) -> Optional[DetectionResult]: |
||||
result = self.detect(data, cache) |
||||
return result if result else None |
||||
|
||||
def get_window_size(self, cache: Optional[ModelCache]) -> int: |
||||
return self.WINDOW_SIZE |
||||
|
||||
def concat_detection_results(self, detections: List[DetectionResult]) -> DetectionResult: |
||||
result = DetectionResult() |
||||
time_step = detections[0].cache['timeStep'] |
||||
for detection in detections: |
||||
result.segments.extend(detection.segments) |
||||
result.last_detection_time = detection.last_detection_time |
||||
result.cache = detection.cache |
||||
result.segments = utils.merge_intersecting_segments(result.segments, time_step) |
||||
return result |
||||
|
||||
def process_data(self, dataframe: pd.DataFrame, cache: ModelCache) -> ProcessingResult: |
||||
data = dataframe['value'] |
||||
value = self.get_value_from_cache(cache, 'value', required = True) |
||||
condition = self.get_value_from_cache(cache, 'condition', required = True) |
||||
|
||||
if condition == 'NO_DATA': |
||||
return ProcessingResult() |
||||
|
||||
data.values[:] = value |
||||
timestamps = utils.convert_series_to_timestamp_list(dataframe.timestamp) |
||||
result_series = list(zip(timestamps, data.values.tolist())) |
||||
|
||||
if condition in ['>', '>=', '=']: |
||||
return ProcessingResult(upper_bound = result_series) |
||||
|
||||
if condition in ['<', '<=']: |
||||
return ProcessingResult(lower_bound = result_series) |
||||
|
||||
raise ValueError(f'{condition} condition not supported') |
@ -1,9 +0,0 @@
|
||||
from models.model import Model, ModelState, AnalyticSegment, ModelType, ExtremumType |
||||
from models.triangle_model import TriangleModel, TriangleModelState |
||||
from models.stair_model import StairModel, StairModelState |
||||
from models.drop_model import DropModel |
||||
from models.peak_model import PeakModel |
||||
from models.jump_model import JumpModel |
||||
from models.custom_model import CustomModel |
||||
from models.trough_model import TroughModel |
||||
from models.general_model import GeneralModel, GeneralModelState |
@ -1,30 +0,0 @@
|
||||
from models import Model, AnalyticSegment, ModelState, ModelType |
||||
from analytic_types import AnalyticUnitId, ModelCache |
||||
from analytic_types.learning_info import LearningInfo |
||||
import utils |
||||
|
||||
import pandas as pd |
||||
from typing import List, Optional |
||||
|
||||
|
||||
class CustomModel(Model): |
||||
def do_fit( |
||||
self, |
||||
dataframe: pd.DataFrame, |
||||
labeled_segments: List[AnalyticSegment], |
||||
deleted_segments: List[AnalyticSegment], |
||||
learning_info: LearningInfo |
||||
) -> None: |
||||
pass |
||||
|
||||
def do_detect(self, dataframe: pd.DataFrame) -> list: |
||||
return [] |
||||
|
||||
def find_segment_center(self, dataframe: pd.DataFrame, start: int, end: int) -> int: |
||||
pass |
||||
|
||||
def get_model_type(self) -> ModelType: |
||||
pass |
||||
|
||||
def get_state(self, cache: Optional[ModelCache] = None) -> ModelState: |
||||
pass |
@ -1,9 +0,0 @@
|
||||
from models import StairModel, ModelType, ExtremumType |
||||
|
||||
class DropModel(StairModel): |
||||
|
||||
def get_model_type(self) -> ModelType: |
||||
return ModelType.DROP |
||||
|
||||
def get_extremum_type(self) -> ExtremumType: |
||||
return ExtremumType.MIN |
@ -1,104 +0,0 @@
|
||||
from analytic_types import AnalyticUnitId |
||||
from models import Model, ModelState, AnalyticSegment, ModelType |
||||
from typing import Union, List, Generator |
||||
import utils |
||||
import utils.meta |
||||
import numpy as np |
||||
import pandas as pd |
||||
import scipy.signal |
||||
from scipy.fftpack import fft |
||||
from scipy.signal import argrelextrema |
||||
from scipy.stats.stats import pearsonr |
||||
|
||||
from scipy.stats import gaussian_kde |
||||
from scipy.stats import norm |
||||
import logging |
||||
|
||||
from typing import Optional, List, Tuple |
||||
import math |
||||
from analytic_types import AnalyticUnitId, TimeSeries |
||||
from analytic_types.learning_info import LearningInfo |
||||
|
||||
PEARSON_FACTOR = 0.7 |
||||
|
||||
|
||||
@utils.meta.JSONClass |
||||
class GeneralModelState(ModelState): |
||||
def __init__(self, **kwargs): |
||||
super().__init__(**kwargs) |
||||
|
||||
|
||||
class GeneralModel(Model): |
||||
|
||||
def get_model_type(self) -> ModelType: |
||||
return ModelType.GENERAL |
||||
|
||||
def find_segment_center(self, dataframe: pd.DataFrame, start: int, end: int) -> int: |
||||
data = dataframe['value'] |
||||
segment = data[start: end] |
||||
center_ind = start + math.ceil((end - start) / 2) |
||||
return center_ind |
||||
|
||||
def get_state(self, cache: Optional[dict] = None) -> GeneralModelState: |
||||
return GeneralModelState.from_json(cache) |
||||
|
||||
def do_fit( |
||||
self, |
||||
dataframe: pd.DataFrame, |
||||
labeled_segments: List[AnalyticSegment], |
||||
deleted_segments: List[AnalyticSegment], |
||||
learning_info: LearningInfo |
||||
) -> None: |
||||
data = utils.cut_dataframe(dataframe) |
||||
data = data['value'] |
||||
last_pattern_center = self.state.pattern_center |
||||
self.state.pattern_center = utils.remove_duplicates_and_sort(last_pattern_center + learning_info.segment_center_list) |
||||
self.state.pattern_model = utils.get_av_model(learning_info.patterns_list) |
||||
convolve_list = utils.get_convolve(self.state.pattern_center, self.state.pattern_model, data, self.state.window_size) |
||||
correlation_list = utils.get_correlation(self.state.pattern_center, self.state.pattern_model, data, self.state.window_size) |
||||
|
||||
del_conv_list = [] |
||||
delete_pattern_timestamp = [] |
||||
for segment in deleted_segments: |
||||
del_mid_index = segment.center_index |
||||
delete_pattern_timestamp.append(segment.pattern_timestamp) |
||||
deleted_pat = utils.get_interval(data, del_mid_index, self.state.window_size) |
||||
deleted_pat = utils.subtract_min_without_nan(deleted_pat) |
||||
del_conv_pat = scipy.signal.fftconvolve(deleted_pat, self.state.pattern_model) |
||||
if len(del_conv_pat): del_conv_list.append(max(del_conv_pat)) |
||||
|
||||
self.state.convolve_min, self.state.convolve_max = utils.get_min_max(convolve_list, self.state.window_size / 3) |
||||
self.state.conv_del_min, self.state.conv_del_max = utils.get_min_max(del_conv_list, self.state.window_size) |
||||
|
||||
def do_detect(self, dataframe: pd.DataFrame) -> TimeSeries: |
||||
data = utils.cut_dataframe(dataframe) |
||||
data = data['value'] |
||||
pat_data = self.state.pattern_model |
||||
if pat_data.count(0) == len(pat_data): |
||||
raise ValueError('Labeled patterns must not be empty') |
||||
|
||||
window_size = self.state.window_size |
||||
all_corr = utils.get_correlation_gen(data, window_size, pat_data) |
||||
all_corr_peaks = utils.find_peaks(all_corr, window_size * 2) |
||||
filtered = self.__filter_detection(all_corr_peaks, data) |
||||
filtered = list(filtered) |
||||
return [(item, item + window_size * 2) for item in filtered] |
||||
|
||||
def __filter_detection(self, segments: Generator[int, None, None], data: pd.Series) -> Generator[int, None, None]: |
||||
if not self.state.pattern_center: |
||||
return [] |
||||
window_size = self.state.window_size |
||||
pattern_model = self.state.pattern_model |
||||
for ind, val in segments: |
||||
watch_data = data[ind - window_size: ind + window_size + 1] |
||||
watch_data = utils.subtract_min_without_nan(watch_data) |
||||
convolve_segment = scipy.signal.fftconvolve(watch_data, pattern_model) |
||||
if len(convolve_segment) > 0: |
||||
watch_conv = max(convolve_segment) |
||||
else: |
||||
continue |
||||
if watch_conv < self.state.convolve_min * 0.8 or val < PEARSON_FACTOR: |
||||
continue |
||||
if watch_conv < self.state.conv_del_max * 1.02 and watch_conv > self.state.conv_del_min * 0.98: |
||||
continue |
||||
yield ind |
@ -1,9 +0,0 @@
|
||||
from models import StairModel, ModelType, ExtremumType |
||||
|
||||
class JumpModel(StairModel): |
||||
|
||||
def get_model_type(self) -> ModelType: |
||||
return ModelType.JUMP |
||||
|
||||
def get_extremum_type(self) -> ExtremumType: |
||||
return ExtremumType.MAX |
@ -1,230 +0,0 @@
|
||||
from analytic_types import AnalyticUnitId, ModelCache, TimeSeries |
||||
from analytic_types.segment import Segment |
||||
from analytic_types.learning_info import LearningInfo |
||||
|
||||
import utils |
||||
import utils.meta |
||||
|
||||
from abc import ABC, abstractmethod |
||||
from attrdict import AttrDict |
||||
from typing import Optional, List, Tuple |
||||
import pandas as pd |
||||
import math |
||||
import logging |
||||
from enum import Enum |
||||
|
||||
class ModelType(Enum): |
||||
JUMP = 'jump' |
||||
DROP = 'drop' |
||||
PEAK = 'peak' |
||||
TROUGH = 'trough' |
||||
GENERAL = 'general' |
||||
|
||||
class ExtremumType(Enum): |
||||
MAX = 'max' |
||||
MIN = 'min' |
||||
|
||||
class AnalyticSegment(Segment): |
||||
''' |
||||
Segment with specific analytics fields used by models: |
||||
- `labeled` / `deleted` flags |
||||
- `from` / `to` / `center` indices |
||||
- `length` |
||||
- `data` |
||||
- etc |
||||
''' |
||||
|
||||
def __init__( |
||||
self, |
||||
from_timestamp: int, |
||||
to_timestamp: int, |
||||
_id: str, |
||||
analytic_unit_id: str, |
||||
labeled: bool, |
||||
deleted: bool, |
||||
message: str, |
||||
dataframe: pd.DataFrame, |
||||
center_finder = None |
||||
): |
||||
super().__init__( |
||||
from_timestamp, |
||||
to_timestamp, |
||||
_id, |
||||
analytic_unit_id, |
||||
labeled, |
||||
deleted, |
||||
message |
||||
) |
||||
|
||||
self.from_index = utils.timestamp_to_index(dataframe, pd.to_datetime(self.from_timestamp, unit='ms')) |
||||
self.to_index = utils.timestamp_to_index(dataframe, pd.to_datetime(self.to_timestamp, unit='ms')) |
||||
self.length = abs(self.to_index - self.from_index) |
||||
self.__percent_of_nans = 0 |
||||
|
||||
if callable(center_finder): |
||||
self.center_index = center_finder(dataframe, self.from_index, self.to_index) |
||||
self.pattern_timestamp = dataframe['timestamp'][self.center_index] |
||||
else: |
||||
self.center_index = self.from_index + math.ceil(self.length / 2) |
||||
self.pattern_timestamp = dataframe['timestamp'][self.center_index] |
||||
|
||||
assert len(dataframe['value']) >= self.to_index + 1, \ |
||||
'segment {}-{} out of dataframe length={}'.format(self.from_index, self.to_index + 1, len(dataframe['value'])) |
||||
|
||||
self.data = dataframe['value'][self.from_index: self.to_index + 1] |
||||
|
||||
@property |
||||
def percent_of_nans(self): |
||||
if not self.__percent_of_nans: |
||||
self.__percent_of_nans = self.data.isnull().sum() / len(self.data) |
||||
return self.__percent_of_nans |
||||
|
||||
def convert_nan_to_zero(self): |
||||
nan_list = utils.find_nan_indexes(self.data) |
||||
self.data = utils.nan_to_zero(self.data, nan_list) |
||||
|
||||
|
||||
@utils.meta.JSONClass |
||||
class ModelState(): |
||||
|
||||
def __init__( |
||||
self, |
||||
time_step: int = 0, |
||||
pattern_center: List[int] = None, |
||||
pattern_model: List[float] = None, |
||||
convolve_max: float = 0, |
||||
convolve_min: float = 0, |
||||
window_size: int = 0, |
||||
conv_del_min: float = 0, |
||||
conv_del_max: float = 0 |
||||
): |
||||
self.time_step = time_step |
||||
self.pattern_center = pattern_center if pattern_center is not None else [] |
||||
self.pattern_model = pattern_model if pattern_model is not None else [] |
||||
self.convolve_max = convolve_max |
||||
self.convolve_min = convolve_min |
||||
self.window_size = window_size |
||||
self.conv_del_min = conv_del_min |
||||
self.conv_del_max = conv_del_max |
||||
|
||||
|
||||
class Model(ABC): |
||||
|
||||
HEIGHT_ERROR = 0.1 |
||||
CONV_ERROR = 0.2 |
||||
DEL_CONV_ERROR = 0.02 |
||||
|
||||
@abstractmethod |
||||
def do_fit( |
||||
self, |
||||
dataframe: pd.DataFrame, |
||||
labeled_segments: List[AnalyticSegment], |
||||
deleted_segments: List[AnalyticSegment], |
||||
learning_info: LearningInfo |
||||
) -> None: |
||||
pass |
||||
|
||||
@abstractmethod |
||||
def do_detect(self, dataframe: pd.DataFrame) -> TimeSeries: |
||||
pass |
||||
|
||||
@abstractmethod |
||||
def find_segment_center(self, dataframe: pd.DataFrame, start: int, end: int) -> int: |
||||
pass |
||||
|
||||
@abstractmethod |
||||
def get_model_type(self) -> ModelType: |
||||
pass |
||||
|
||||
@abstractmethod |
||||
def get_state(self, cache: Optional[ModelCache] = None) -> ModelState: |
||||
pass |
||||
|
||||
def fit(self, dataframe: pd.DataFrame, segments: List[Segment], id: AnalyticUnitId) -> ModelState: |
||||
logging.debug('Start method fit for analytic unit {}'.format(id)) |
||||
data = dataframe['value'] |
||||
max_length = 0 |
||||
labeled = [] |
||||
deleted = [] |
||||
for segment_map in segments: |
||||
if segment_map.labeled or segment_map.deleted: |
||||
segment = AnalyticSegment( |
||||
segment_map.from_timestamp, |
||||
segment_map.to_timestamp, |
||||
segment_map._id, |
||||
segment_map.analytic_unit_id, |
||||
segment_map.labeled, |
||||
segment_map.deleted, |
||||
segment_map.message, |
||||
dataframe, |
||||
self.find_segment_center |
||||
) |
||||
if segment.percent_of_nans > 0.1 or len(segment.data) == 0: |
||||
logging.debug(f'segment {segment.from_index}-{segment.to_index} skip because of invalid data') |
||||
continue |
||||
if segment.percent_of_nans > 0: |
||||
segment.convert_nan_to_zero() |
||||
max_length = max(segment.length, max_length) |
||||
if segment.labeled: labeled.append(segment) |
||||
if segment.deleted: deleted.append(segment) |
||||
|
||||
assert len(labeled) > 0, f'labeled list empty, skip fitting for {id}' |
||||
|
||||
if self.state.window_size == 0: |
||||
self.state.window_size = math.ceil(max_length / 2) if max_length else 0 |
||||
learning_info = self.get_parameters_from_segments(dataframe, labeled, deleted, self.get_model_type()) |
||||
self.do_fit(dataframe, labeled, deleted, learning_info) |
||||
logging.debug('fit complete successful with self.state: {} for analytic unit: {}'.format(self.state, id)) |
||||
return self.state |
||||
|
||||
def detect(self, dataframe: pd.DataFrame, id: AnalyticUnitId) -> dict: |
||||
logging.debug('Start method detect for analytic unit {}'.format(id)) |
||||
result = self.do_detect(dataframe) |
||||
segments = [( |
||||
utils.convert_pd_timestamp_to_ms(dataframe['timestamp'][x[0]]), |
||||
utils.convert_pd_timestamp_to_ms(dataframe['timestamp'][x[1]]), |
||||
) for x in result] |
||||
if not self.state: |
||||
logging.warning('Return empty self.state after detect') |
||||
logging.debug('Method detect complete successful for analytic unit {}'.format(id)) |
||||
return { |
||||
'segments': segments, |
||||
'cache': self.state, |
||||
} |
||||
|
||||
def _update_fitting_result(self, state: ModelState, confidences: list, convolve_list: list, del_conv_list: list, height_list: Optional[list] = None) -> None: |
||||
state.confidence = float(min(confidences, default = 1.5)) |
||||
state.convolve_min, state.convolve_max = utils.get_min_max(convolve_list, state.window_size) |
||||
state.conv_del_min, state.conv_del_max = utils.get_min_max(del_conv_list, 0) |
||||
if height_list is not None: |
||||
state.height_min, state.height_max = utils.get_min_max(height_list, 0) |
||||
|
||||
def get_parameters_from_segments(self, dataframe: pd.DataFrame, labeled: List[dict], deleted: List[dict], model: ModelType) -> dict: |
||||
logging.debug('Start parsing segments') |
||||
learning_info = LearningInfo() |
||||
data = dataframe['value'] |
||||
for segment in labeled: |
||||
confidence = utils.find_confidence(segment.data)[0] |
||||
learning_info.confidence.append(confidence) |
||||
segment_center = segment.center_index |
||||
learning_info.segment_center_list.append(segment_center) |
||||
learning_info.pattern_timestamp.append(segment.pattern_timestamp) |
||||
aligned_segment = utils.get_interval(data, segment_center, self.state.window_size) |
||||
aligned_segment = utils.subtract_min_without_nan(aligned_segment) |
||||
if len(aligned_segment) == 0: |
||||
logging.warning('cant add segment to learning because segment is empty where segments center is: {}, window_size: {}, and len_data: {}'.format( |
||||
segment_center, self.state.window_size, len(data))) |
||||
continue |
||||
learning_info.patterns_list.append(aligned_segment) |
||||
# TODO: use Triangle/Stair types |
||||
if model == ModelType.PEAK or model == ModelType.TROUGH: |
||||
learning_info.pattern_height.append(utils.find_confidence(aligned_segment)[1]) |
||||
learning_info.patterns_value.append(aligned_segment.values.max()) |
||||
if model == ModelType.JUMP or model == ModelType.DROP: |
||||
pattern_height, pattern_length = utils.find_parameters(segment.data, segment.from_index, model.value) |
||||
learning_info.pattern_height.append(pattern_height) |
||||
learning_info.pattern_width.append(pattern_length) |
||||
learning_info.patterns_value.append(aligned_segment.values[self.state.window_size]) |
||||
logging.debug('Parsing segments ended correctly with learning_info: {}'.format(learning_info)) |
||||
return learning_info |
||||
|
@ -1,44 +0,0 @@
|
||||
from analytic_types import TimeSeries |
||||
from models import TriangleModel, ModelType |
||||
import utils |
||||
|
||||
import scipy.signal |
||||
from scipy.signal import argrelextrema |
||||
from typing import Optional, List, Tuple |
||||
import numpy as np |
||||
import pandas as pd |
||||
|
||||
class PeakModel(TriangleModel): |
||||
|
||||
def get_model_type(self) -> ModelType: |
||||
return ModelType.PEAK |
||||
|
||||
def find_segment_center(self, dataframe: pd.DataFrame, start: int, end: int) -> int: |
||||
data = dataframe['value'] |
||||
segment = data[start: end] |
||||
return segment.idxmax() |
||||
|
||||
def get_best_pattern(self, close_patterns: TimeSeries, data: pd.Series) -> List[int]: |
||||
pattern_list = [] |
||||
for val in close_patterns: |
||||
max_val = data[val[0]] |
||||
ind = val[0] |
||||
for i in val: |
||||
if data[i] > max_val: |
||||
max_val = data[i] |
||||
ind = i |
||||
pattern_list.append(ind) |
||||
return pattern_list |
||||
|
||||
def get_extremum_indexes(self, data: pd.Series) -> np.ndarray: |
||||
return argrelextrema(data.values, np.greater)[0] |
||||
|
||||
def get_smoothed_data(self, data: pd.Series, confidence: float, alpha: float) -> pd.Series: |
||||
return utils.exponential_smoothing(data + self.state.confidence, alpha) |
||||
|
||||
def get_possible_segments(self, data: pd.Series, smoothed_data: pd.Series, peak_indexes: List[int]) -> List[int]: |
||||
segments = [] |
||||
for idx in peak_indexes: |
||||
if data[idx] > smoothed_data[idx]: |
||||
segments.append(idx) |
||||
return segments |
@ -1,147 +0,0 @@
|
||||
from models import Model, ModelState, AnalyticSegment, ModelType |
||||
|
||||
from analytic_types import TimeSeries |
||||
from analytic_types.learning_info import LearningInfo |
||||
|
||||
from scipy.fftpack import fft |
||||
from typing import Optional, List |
||||
from enum import Enum |
||||
import scipy.signal |
||||
import utils |
||||
import utils.meta |
||||
import pandas as pd |
||||
import numpy as np |
||||
import operator |
||||
|
||||
POSITIVE_SEGMENT_MEASUREMENT_ERROR = 0.2 |
||||
NEGATIVE_SEGMENT_MEASUREMENT_ERROR = 0.02 |
||||
|
||||
@utils.meta.JSONClass |
||||
class StairModelState(ModelState): |
||||
|
||||
def __init__( |
||||
self, |
||||
confidence: float = 0, |
||||
stair_height: float = 0, |
||||
stair_length: float = 0, |
||||
**kwargs |
||||
): |
||||
super().__init__(**kwargs) |
||||
self.confidence = confidence |
||||
self.stair_height = stair_height |
||||
self.stair_length = stair_length |
||||
|
||||
|
||||
class StairModel(Model): |
||||
|
||||
def get_state(self, cache: Optional[dict] = None) -> StairModelState: |
||||
return StairModelState.from_json(cache) |
||||
|
||||
def get_stair_indexes(self, data: pd.Series, height: float, length: int) -> List[int]: |
||||
"""Get list of start stair segment indexes. |
||||
|
||||
Keyword arguments: |
||||
data -- data, that contains stair (jump or drop) segments |
||||
length -- maximum count of values in the stair |
||||
height -- the difference between stair max_line and min_line(see utils.find_parameters) |
||||
""" |
||||
indexes = [] |
||||
for i in range(len(data) - length - 1): |
||||
is_stair = self.is_stair_in_segment(data.values[i:i + length + 1], height) |
||||
if is_stair == True: |
||||
indexes.append(i) |
||||
return indexes |
||||
|
||||
def is_stair_in_segment(self, segment: np.ndarray, height: float) -> bool: |
||||
if len(segment) < 2: |
||||
return False |
||||
comparison_operator = operator.ge |
||||
if self.get_model_type() == ModelType.DROP: |
||||
comparison_operator = operator.le |
||||
height = -height |
||||
return comparison_operator(max(segment[1:]), segment[0] + height) |
||||
|
||||
def find_segment_center(self, dataframe: pd.DataFrame, start: int, end: int) -> int: |
||||
data = dataframe['value'] |
||||
segment = data[start: end] |
||||
segment_center_index = utils.find_pattern_center(segment, start, self.get_model_type().value) |
||||
return segment_center_index |
||||
|
||||
def do_fit( |
||||
self, |
||||
dataframe: pd.DataFrame, |
||||
labeled_segments: List[AnalyticSegment], |
||||
deleted_segments: List[AnalyticSegment], |
||||
learning_info: LearningInfo |
||||
) -> None: |
||||
data = utils.cut_dataframe(dataframe) |
||||
data = data['value'] |
||||
window_size = self.state.window_size |
||||
last_pattern_center = self.state.pattern_center |
||||
self.state.pattern_center = utils.remove_duplicates_and_sort(last_pattern_center + learning_info.segment_center_list) |
||||
self.state.pattern_model = utils.get_av_model(learning_info.patterns_list) |
||||
convolve_list = utils.get_convolve(self.state.pattern_center, self.state.pattern_model, data, window_size) |
||||
correlation_list = utils.get_correlation(self.state.pattern_center, self.state.pattern_model, data, window_size) |
||||
height_list = learning_info.patterns_value |
||||
|
||||
del_conv_list = [] |
||||
delete_pattern_timestamp = [] |
||||
for segment in deleted_segments: |
||||
segment_cent_index = segment.center_index |
||||
delete_pattern_timestamp.append(segment.pattern_timestamp) |
||||
deleted_stair = utils.get_interval(data, segment_cent_index, window_size) |
||||
deleted_stair = utils.subtract_min_without_nan(deleted_stair) |
||||
del_conv_stair = scipy.signal.fftconvolve(deleted_stair, self.state.pattern_model) |
||||
if len(del_conv_stair) > 0: |
||||
del_conv_list.append(max(del_conv_stair)) |
||||
|
||||
self._update_fitting_result(self.state, learning_info.confidence, convolve_list, del_conv_list) |
||||
self.state.stair_height = int(min(learning_info.pattern_height, default = 1)) |
||||
self.state.stair_length = int(max(learning_info.pattern_width, default = 1)) |
||||
|
||||
def do_detect(self, dataframe: pd.DataFrame) -> TimeSeries: |
||||
data = utils.cut_dataframe(dataframe) |
||||
data = data['value'] |
||||
possible_stairs = self.get_stair_indexes(data, self.state.stair_height, self.state.stair_length + 1) |
||||
result = self.__filter_detection(possible_stairs, data) |
||||
return [(val - 1, val + 1) for val in result] |
||||
|
||||
def __filter_detection(self, segments_indexes: List[int], data: list): |
||||
delete_list = [] |
||||
variance_error = self.state.window_size |
||||
close_segments = utils.close_filtering(segments_indexes, variance_error) |
||||
segments_indexes = utils.best_pattern(close_segments, data, self.get_extremum_type().value) |
||||
if len(segments_indexes) == 0 or len(self.state.pattern_center) == 0: |
||||
return [] |
||||
pattern_data = self.state.pattern_model |
||||
for segment_index in segments_indexes: |
||||
if segment_index <= self.state.window_size or segment_index >= (len(data) - self.state.window_size): |
||||
delete_list.append(segment_index) |
||||
continue |
||||
convol_data = utils.get_interval(data, segment_index, self.state.window_size) |
||||
percent_of_nans = convol_data.isnull().sum() / len(convol_data) |
||||
if len(convol_data) == 0 or percent_of_nans > 0.5: |
||||
delete_list.append(segment_index) |
||||
continue |
||||
elif 0 < percent_of_nans <= 0.5: |
||||
nan_list = utils.find_nan_indexes(convol_data) |
||||
convol_data = utils.nan_to_zero(convol_data, nan_list) |
||||
pattern_data = utils.nan_to_zero(pattern_data, nan_list) |
||||
conv = scipy.signal.fftconvolve(convol_data, pattern_data) |
||||
if len(conv) == 0: |
||||
delete_list.append(segment_index) |
||||
continue |
||||
upper_bound = self.state.convolve_max * (1 + POSITIVE_SEGMENT_MEASUREMENT_ERROR) |
||||
lower_bound = self.state.convolve_min * (1 - POSITIVE_SEGMENT_MEASUREMENT_ERROR) |
||||
delete_up_bound = self.state.conv_del_max * (1 + NEGATIVE_SEGMENT_MEASUREMENT_ERROR) |
||||
delete_low_bound = self.state.conv_del_min * (1 - NEGATIVE_SEGMENT_MEASUREMENT_ERROR) |
||||
max_conv = max(conv) |
||||
if max_conv > upper_bound or max_conv < lower_bound: |
||||
delete_list.append(segment_index) |
||||
elif max_conv < delete_up_bound and max_conv > delete_low_bound: |
||||
delete_list.append(segment_index) |
||||
|
||||
for item in delete_list: |
||||
segments_indexes.remove(item) |
||||
segments_indexes = utils.remove_duplicates_and_sort(segments_indexes) |
||||
return segments_indexes |
@ -1,119 +0,0 @@
|
||||
from analytic_types import AnalyticUnitId, TimeSeries |
||||
from analytic_types.learning_info import LearningInfo |
||||
from models import Model, ModelState, AnalyticSegment |
||||
import utils |
||||
import utils.meta |
||||
|
||||
import scipy.signal |
||||
from scipy.fftpack import fft |
||||
from typing import Optional, List, Tuple |
||||
import numpy as np |
||||
import pandas as pd |
||||
|
||||
|
||||
EXP_SMOOTHING_FACTOR = 0.01 |
||||
|
||||
|
||||
@utils.meta.JSONClass |
||||
class TriangleModelState(ModelState): |
||||
|
||||
def __init__( |
||||
self, |
||||
confidence: float = 0, |
||||
height_max: float = 0, |
||||
height_min: float = 0, |
||||
**kwargs |
||||
): |
||||
super().__init__(**kwargs) |
||||
self.confidence = confidence |
||||
self.height_max = height_max |
||||
self.height_min = height_min |
||||
|
||||
class TriangleModel(Model): |
||||
|
||||
def get_state(self, cache: Optional[dict] = None) -> TriangleModelState: |
||||
return TriangleModelState.from_json(cache) |
||||
|
||||
def do_fit( |
||||
self, |
||||
dataframe: pd.DataFrame, |
||||
labeled_segments: List[AnalyticSegment], |
||||
deleted_segments: List[AnalyticSegment], |
||||
learning_info: LearningInfo |
||||
) -> None: |
||||
data = utils.cut_dataframe(dataframe) |
||||
data = data['value'] |
||||
self.state.pattern_center = utils.remove_duplicates_and_sort(self.state.pattern_center + learning_info.segment_center_list) |
||||
self.state.pattern_model = utils.get_av_model(learning_info.patterns_list) |
||||
convolve_list = utils.get_convolve(self.state.pattern_center, self.state.pattern_model, data, self.state.window_size) |
||||
correlation_list = utils.get_correlation(self.state.pattern_center, self.state.pattern_model, data, self.state.window_size) |
||||
height_list = learning_info.patterns_value |
||||
|
||||
del_conv_list = [] |
||||
delete_pattern_width = [] |
||||
delete_pattern_height = [] |
||||
delete_pattern_timestamp = [] |
||||
for segment in deleted_segments: |
||||
delete_pattern_timestamp.append(segment.pattern_timestamp) |
||||
deleted = utils.get_interval(data, segment.center_index, self.state.window_size) |
||||
deleted = utils.subtract_min_without_nan(deleted) |
||||
del_conv = scipy.signal.fftconvolve(deleted, self.state.pattern_model) |
||||
if len(del_conv): |
||||
del_conv_list.append(max(del_conv)) |
||||
delete_pattern_height.append(utils.find_confidence(deleted)[1]) |
||||
|
||||
self._update_fitting_result(self.state, learning_info.confidence, convolve_list, del_conv_list, height_list) |
||||
|
||||
def do_detect(self, dataframe: pd.DataFrame) -> TimeSeries: |
||||
data = utils.cut_dataframe(dataframe) |
||||
data = data['value'] |
||||
|
||||
all_extremum_indexes = self.get_extremum_indexes(data) |
||||
smoothed_data = self.get_smoothed_data(data, self.state.confidence, EXP_SMOOTHING_FACTOR) |
||||
segments = self.get_possible_segments(data, smoothed_data, all_extremum_indexes) |
||||
result = self.__filter_detection(segments, data) |
||||
result = utils.get_borders_of_peaks(result, data, self.state.window_size, self.state.confidence) |
||||
return result |
||||
|
||||
def __filter_detection(self, segments: List[int], data: pd.Series) -> list: |
||||
delete_list = [] |
||||
variance_error = self.state.window_size |
||||
close_patterns = utils.close_filtering(segments, variance_error) |
||||
segments = self.get_best_pattern(close_patterns, data) |
||||
|
||||
if len(segments) == 0 or len(self.state.pattern_model) == 0: |
||||
return [] |
||||
pattern_data = self.state.pattern_model |
||||
up_height = self.state.height_max * (1 + self.HEIGHT_ERROR) |
||||
low_height = self.state.height_min * (1 - self.HEIGHT_ERROR) |
||||
up_conv = self.state.convolve_max * (1 + 1.5 * self.CONV_ERROR) |
||||
low_conv = self.state.convolve_min * (1 - self.CONV_ERROR) |
||||
up_del_conv = self.state.conv_del_max * (1 + self.DEL_CONV_ERROR) |
||||
low_del_conv = self.state.conv_del_min * (1 - self.DEL_CONV_ERROR) |
||||
for segment in segments: |
||||
if segment > self.state.window_size: |
||||
convol_data = utils.get_interval(data, segment, self.state.window_size) |
||||
convol_data = utils.subtract_min_without_nan(convol_data) |
||||
percent_of_nans = convol_data.isnull().sum() / len(convol_data) |
||||
if percent_of_nans > 0.5: |
||||
delete_list.append(segment) |
||||
continue |
||||
elif 0 < percent_of_nans <= 0.5: |
||||
nan_list = utils.find_nan_indexes(convol_data) |
||||
convol_data = utils.nan_to_zero(convol_data, nan_list) |
||||
pattern_data = utils.nan_to_zero(pattern_data, nan_list) |
||||
conv = scipy.signal.fftconvolve(convol_data, pattern_data) |
||||
pattern_height = convol_data.values.max() |
||||
if pattern_height > up_height or pattern_height < low_height: |
||||
delete_list.append(segment) |
||||
continue |
||||
if max(conv) > up_conv or max(conv) < low_conv: |
||||
delete_list.append(segment) |
||||
continue |
||||
if max(conv) < up_del_conv and max(conv) > low_del_conv: |
||||
delete_list.append(segment) |
||||
else: |
||||
delete_list.append(segment) |
||||
for item in delete_list: |
||||
segments.remove(item) |
||||
return set(segments) |
@ -1,44 +0,0 @@
|
||||
from analytic_types import TimeSeries |
||||
from models import TriangleModel, ModelType |
||||
import utils |
||||
|
||||
import scipy.signal |
||||
from scipy.signal import argrelextrema |
||||
from typing import Optional, List, Tuple |
||||
import numpy as np |
||||
import pandas as pd |
||||
|
||||
class TroughModel(TriangleModel): |
||||
|
||||
def get_model_type(self) -> ModelType: |
||||
return ModelType.TROUGH |
||||
|
||||
def find_segment_center(self, dataframe: pd.DataFrame, start: int, end: int) -> int: |
||||
data = dataframe['value'] |
||||
segment = data[start: end] |
||||
return segment.idxmin() |
||||
|
||||
def get_best_pattern(self, close_patterns: TimeSeries, data: pd.Series) -> List[int]: |
||||
pattern_list = [] |
||||
for val in close_patterns: |
||||
min_val = data[val[0]] |
||||
ind = val[0] |
||||
for i in val: |
||||
if data[i] < min_val: |
||||
min_val = data[i] |
||||
ind = i |
||||
pattern_list.append(ind) |
||||
return pattern_list |
||||
|
||||
def get_extremum_indexes(self, data: pd.Series) -> np.ndarray: |
||||
return argrelextrema(data.values, np.less)[0] |
||||
|
||||
def get_smoothed_data(self, data: pd.Series, confidence: float, alpha: float) -> pd.Series: |
||||
return utils.exponential_smoothing(data - self.state.confidence, alpha) |
||||
|
||||
def get_possible_segments(self, data: pd.Series, smoothed_data: pd.Series, trough_indexes: List[int]) -> List[int]: |
||||
segments = [] |
||||
for idx in trough_indexes: |
||||
if data[idx] < smoothed_data[idx]: |
||||
segments.append(idx) |
||||
return segments |
@ -1,94 +0,0 @@
|
||||
#!/usr/bin/env python3 |
||||
|
||||
import sys |
||||
import os |
||||
|
||||
|
||||
import config |
||||
import json |
||||
import logging |
||||
import asyncio |
||||
import traceback |
||||
|
||||
import services |
||||
from analytic_unit_manager import AnalyticUnitManager |
||||
|
||||
|
||||
server_service: services.ServerService = None |
||||
data_service: services.DataService = None |
||||
analytic_unit_manager: AnalyticUnitManager = None |
||||
|
||||
logger = logging.getLogger('SERVER') |
||||
|
||||
|
||||
async def handle_task(task: object): |
||||
try: |
||||
task_type = task['type'] |
||||
logger.info("Got {} task with id {}, analyticUnitId {}".format(task_type, task['_id'], task['analyticUnitId'])) |
||||
|
||||
task_result_payload = { |
||||
'_id': task['_id'], |
||||
'task': task_type, |
||||
'analyticUnitId': task['analyticUnitId'], |
||||
'status': "IN_PROGRESS" |
||||
} |
||||
|
||||
if not task_type == 'PUSH': |
||||
message = services.server_service.ServerMessage('TASK_RESULT', task_result_payload) |
||||
await server_service.send_message_to_server(message) |
||||
|
||||
res = await analytic_unit_manager.handle_analytic_task(task) |
||||
res['_id'] = task['_id'] |
||||
|
||||
if not task_type == 'PUSH': |
||||
message = services.server_service.ServerMessage('TASK_RESULT', res) |
||||
await server_service.send_message_to_server(message) |
||||
|
||||
except Exception as e: |
||||
error_text = traceback.format_exc() |
||||
logger.error("handle_task Exception: '%s'" % error_text) |
||||
|
||||
async def handle_data(task: object): |
||||
res = await analytic_unit_manager.handle_analytic_task(task) |
||||
|
||||
if res['status'] == 'SUCCESS' and res['payload'] is not None: |
||||
res['_id'] = task['_id'] |
||||
message = services.server_service.ServerMessage('PUSH_DETECT', res) |
||||
await server_service.send_message_to_server(message) |
||||
|
||||
async def handle_message(message: services.ServerMessage): |
||||
if message.method == 'TASK': |
||||
await handle_task(message.payload) |
||||
if message.method == 'DATA': |
||||
await handle_data(message.payload) |
||||
|
||||
def init_services(): |
||||
global server_service |
||||
global data_service |
||||
global analytic_unit_manager |
||||
|
||||
logger.info("Starting services...") |
||||
logger.info("Server...") |
||||
server_service = services.ServerService() |
||||
logger.info("Ok") |
||||
logger.info("Data service...") |
||||
data_service = services.DataService(server_service) |
||||
logger.info("Ok") |
||||
logger.info("Analytic unit manager...") |
||||
analytic_unit_manager = AnalyticUnitManager() |
||||
logger.info("Ok") |
||||
|
||||
async def app_loop(): |
||||
async for message in server_service: |
||||
asyncio.ensure_future(handle_message(message)) |
||||
|
||||
|
||||
def run_server(): |
||||
loop = asyncio.get_event_loop() |
||||
#loop.set_debug(True) |
||||
logger.info("Ok") |
||||
init_services() |
||||
print('Analytics process is running') # we need to print to stdout and flush |
||||
sys.stdout.flush() # because node.js expects it |
||||
|
||||
loop.run_until_complete(app_loop()) |
@ -1,2 +0,0 @@
|
||||
from services.server_service import ServerService, ServerMessage |
||||
from services.data_service import DataService |
@ -1,85 +0,0 @@
|
||||
from services.server_service import ServerMessage, ServerService |
||||
|
||||
import json |
||||
import asyncio |
||||
|
||||
""" |
||||
This is how you can save a file: |
||||
|
||||
async def test_file_save(): |
||||
async with data_service.open('filename') as f: |
||||
print('write content') |
||||
await f.write('test string') |
||||
|
||||
async with data_service.open('filename') as f: |
||||
content = await f.load() |
||||
print(content) |
||||
print('test file ok') |
||||
""" |
||||
|
||||
|
||||
LOCK_WAIT_SLEEP_TIMESPAN = 100 # mc |
||||
|
||||
class FileDescriptor: |
||||
def __init__(self, filename: str, data_service): |
||||
self.filename = filename |
||||
self.data_service = data_service |
||||
|
||||
async def write(self, content: str): |
||||
await self.data_service.save_file_content(self, content) |
||||
|
||||
async def load(self) -> str: |
||||
return await self.data_service.load_file_content(self) |
||||
|
||||
async def __aenter__(self): |
||||
await self.data_service.wait_and_lock(self) |
||||
return self |
||||
|
||||
async def __aexit__(self, *exc): |
||||
await self.data_service.unlock(self) |
||||
|
||||
|
||||
class DataService: |
||||
|
||||
def __init__(self, server_service: ServerService): |
||||
"""Creates fs over network via server_service""" |
||||
self.server_service = server_service |
||||
self.locks = set() |
||||
|
||||
def open(self, filename: str) -> FileDescriptor: |
||||
return FileDescriptor(filename, self) |
||||
|
||||
async def wait_and_lock(self, file_descriptor: FileDescriptor): |
||||
filename = file_descriptor.filename |
||||
while True: |
||||
if filename in self.locks: |
||||
asyncio.sleep(LOCK_WAIT_SLEEP_TIMESPAN) |
||||
continue |
||||
else: |
||||
self.locks.add(filename) |
||||
break |
||||
|
||||
async def unlock(self, file_descriptor: FileDescriptor): |
||||
filename = file_descriptor.filename |
||||
self.locks.remove(filename) |
||||
|
||||
async def save_file_content(self, file_descriptor: FileDescriptor, content: str): |
||||
""" Saves json - serializable obj with file_descriptor.filename """ |
||||
self.__check_lock(file_descriptor) |
||||
message_payload = { |
||||
'filename': file_descriptor.filename, |
||||
'content': content |
||||
} |
||||
message = ServerMessage('FILE_SAVE', message_payload) |
||||
await self.server_service.send_request_to_server(message) |
||||
|
||||
async def load_file_content(self, file_descriptor: FileDescriptor) -> str: |
||||
self.__check_lock(file_descriptor) |
||||
message_payload = { 'filename': file_descriptor.filename } |
||||
message = ServerMessage('FILE_LOAD', message_payload) |
||||
return await self.server_service.send_request_to_server(message) |
||||
|
||||
def __check_lock(self, file_descriptor: FileDescriptor): |
||||
filename = file_descriptor.filename |
||||
if filename not in self.locks: |
||||
raise RuntimeError('No lock for file %s' % filename) |
@ -1,132 +0,0 @@
|
||||
import config |
||||
|
||||
import websockets |
||||
|
||||
import logging |
||||
import json |
||||
import asyncio |
||||
import traceback |
||||
|
||||
import utils.concurrent |
||||
import utils.meta |
||||
|
||||
from typing import Optional |
||||
|
||||
logger = logging.getLogger('SERVER_SERVICE') |
||||
|
||||
|
||||
PARSE_MESSAGE_OR_SAVE_LOOP_INTERRUPTED = False |
||||
SERVER_SOCKET_RECV_LOOP_INTERRUPTED = False |
||||
|
||||
|
||||
@utils.meta.JSONClass |
||||
class ServerMessage: |
||||
def __init__(self, method: str, payload: object = None, request_id: int = None): |
||||
# TODO: add error type / case |
||||
self.method = method |
||||
self.payload = payload |
||||
self.request_id = request_id |
||||
|
||||
|
||||
class ServerService(utils.concurrent.AsyncZmqActor): |
||||
|
||||
def __init__(self): |
||||
super(ServerService, self).__init__() |
||||
self.__aiter_inited = False |
||||
# this typing doesn't help vscode, maybe there is a mistake |
||||
self.__server_socket: Optional[websockets.Connect] = None |
||||
self.__request_next_id = 1 |
||||
self.__responses = dict() |
||||
self.start() |
||||
|
||||
async def send_message_to_server(self, message: ServerMessage): |
||||
# Following message will be sent to actor's self._on_message() |
||||
# We do it cuz we created self.__server_socket in self._run() method, |
||||
# which runs in the actor's thread, not the thread we created ServerService |
||||
|
||||
# in theory, we can try to use zmq.proxy: |
||||
# zmq.proxy(self.__actor_socket, self.__server_socket) |
||||
# and do here something like: |
||||
# self.__actor_socket.send_string(json.dumps(message.to_json())) |
||||
await self._put_message_to_thread(json.dumps(message.to_json())) |
||||
|
||||
async def send_request_to_server(self, message: ServerMessage) -> object: |
||||
if message.request_id is not None: |
||||
raise ValueError('Message can`t have request_id before it is scheduled') |
||||
request_id = message.request_id = self.__request_next_id |
||||
self.request_next_id = self.__request_next_id + 1 |
||||
asyncio.ensure_future(self.send_message_to_server(message)) |
||||
# you should await self.__responses[request_id] which should be a task, |
||||
# which you resolve somewhere else |
||||
while request_id not in self.__responses: |
||||
await asyncio.sleep(1) |
||||
response = self.__responses[request_id] |
||||
del self.__responses[request_id] |
||||
return response |
||||
|
||||
def __aiter__(self): |
||||
if self.__aiter_inited: |
||||
raise RuntimeError('Can`t iterate twice') |
||||
__aiter_inited = True |
||||
return self |
||||
|
||||
async def __anext__(self) -> ServerMessage: |
||||
while not PARSE_MESSAGE_OR_SAVE_LOOP_INTERRUPTED: |
||||
thread_message = await self._recv_message_from_thread() |
||||
server_message = self.__parse_message_or_save(thread_message) |
||||
if server_message is None: |
||||
continue |
||||
else: |
||||
return server_message |
||||
|
||||
async def _run_thread(self): |
||||
logger.info("Binding to %s ..." % config.HASTIC_SERVER_URL) |
||||
# TODO: consider to use async context for socket |
||||
await self.__server_socket_recv_loop() |
||||
|
||||
async def _on_message_to_thread(self, message: str): |
||||
await self.__server_socket.send(message) |
||||
|
||||
async def __server_socket_recv_loop(self): |
||||
while not SERVER_SOCKET_RECV_LOOP_INTERRUPTED: |
||||
received_string = await self.__reconnect_recv() |
||||
if received_string == 'PING': |
||||
asyncio.ensure_future(self.__handle_ping()) |
||||
else: |
||||
asyncio.ensure_future(self._send_message_from_thread(received_string)) |
||||
|
||||
async def __reconnect_recv(self) -> str: |
||||
while not SERVER_SOCKET_RECV_LOOP_INTERRUPTED: |
||||
try: |
||||
if self.__server_socket is None: |
||||
self.__server_socket = await websockets.connect(config.HASTIC_SERVER_URL) |
||||
first_message = await self.__server_socket.recv() |
||||
if first_message == 'EALREADYEXISTING': |
||||
raise ConnectionError('Can`t connect as a second analytics') |
||||
return await self.__server_socket.recv() |
||||
except (ConnectionRefusedError, websockets.ConnectionClosedError): |
||||
if not self.__server_socket is None: |
||||
self.__server_socket.close() |
||||
# TODO: this logic increases the number of ThreadPoolExecutor |
||||
self.__server_socket = None |
||||
# TODO: move to config |
||||
reconnect_delay = 3 |
||||
print('connection is refused or lost, trying to reconnect in %s seconds' % reconnect_delay) |
||||
await asyncio.sleep(reconnect_delay) |
||||
raise InterruptedError() |
||||
|
||||
async def __handle_ping(self): |
||||
# TODO: self.__server_socket can be None |
||||
await self.__server_socket.send('PONG') |
||||
|
||||
def __parse_message_or_save(self, text: str) -> Optional[ServerMessage]: |
||||
try: |
||||
message_object = json.loads(text) |
||||
message = ServerMessage.from_json(message_object) |
||||
if message.request_id is not None: |
||||
self.__responses[message_object['requestId']] = message.payload |
||||
return None |
||||
return message |
||||
except Exception: |
||||
error_text = traceback.format_exc() |
||||
logger.error("__handle_message Exception: '%s'" % error_text) |
@ -1,4 +0,0 @@
|
||||
from utils.common import * |
||||
from utils.time import * |
||||
from utils.dataframe import * |
||||
from utils.meta import * |
@ -1,443 +0,0 @@
|
||||
import numpy as np |
||||
import pandas as pd |
||||
import scipy.signal |
||||
from scipy.fftpack import fft |
||||
from scipy.signal import argrelextrema |
||||
from scipy.stats import gaussian_kde |
||||
from scipy.stats.stats import pearsonr |
||||
import math |
||||
from typing import Optional, Union, List, Generator, Tuple |
||||
import utils |
||||
import logging |
||||
from itertools import islice |
||||
from collections import deque |
||||
from analytic_types import TimeSeries |
||||
from analytic_types.segment import Segment |
||||
|
||||
SHIFT_FACTOR = 0.05 |
||||
CONFIDENCE_FACTOR = 0.5 |
||||
SMOOTHING_FACTOR = 5 |
||||
MEASUREMENT_ERROR = 0.05 |
||||
|
||||
|
||||
def exponential_smoothing(series: pd.Series, alpha: float, last_smoothed_value: Optional[float] = None) -> pd.Series: |
||||
if alpha < 0 or alpha > 1: |
||||
raise ValueError('Alpha must be within the boundaries: 0 <= alpha <= 1') |
||||
if len(series) < 2: |
||||
return series |
||||
if last_smoothed_value is None: |
||||
result = [series.values[0]] |
||||
else: |
||||
result = [float(last_smoothed_value)] |
||||
if np.isnan(result): |
||||
result = [0] |
||||
for n in range(1, len(series)): |
||||
if np.isnan(series[n]): |
||||
result.append((1 - alpha) * result[n - 1]) |
||||
series.values[n] = result[n] |
||||
else: |
||||
result.append(alpha * series[n] + (1 - alpha) * result[n - 1]) |
||||
|
||||
assert len(result) == len(series), \ |
||||
f'len of smoothed data {len(result)} != len of original dataset {len(series)}' |
||||
return pd.Series(result, index = series.index) |
||||
|
||||
def find_pattern(data: pd.Series, height: float, length: int, pattern_type: str) -> list: |
||||
pattern_list = [] |
||||
right_bound = len(data) - length - 1 |
||||
for i in range(right_bound): |
||||
for x in range(1, length): |
||||
if pattern_type == 'jump': |
||||
if(data[i + x] > data[i] + height): |
||||
pattern_list.append(i) |
||||
elif pattern_type == 'drop': |
||||
if(data[i + x] < data[i] - height): |
||||
pattern_list.append(i) |
||||
return pattern_list |
||||
|
||||
def timestamp_to_index(dataframe: pd.DataFrame, timestamp: int): |
||||
data = dataframe['timestamp'] |
||||
idx, = np.where(data >= timestamp) |
||||
if len(idx) > 0: |
||||
time_ind = int(idx[0]) |
||||
else: |
||||
raise ValueError('Dataframe doesn`t contain timestamp: {}'.format(timestamp)) |
||||
return time_ind |
||||
|
||||
def find_peaks(data: Generator[float, None, None], size: int) -> Generator[float, None, None]: |
||||
window = deque(islice(data, size * 2 + 1)) |
||||
for i, v in enumerate(data, size): |
||||
current = window[size] |
||||
#TODO: remove max() from loop |
||||
if current == max(window) and current != window[size + 1]: |
||||
yield i, current |
||||
window.append(v) |
||||
window.popleft() |
||||
|
||||
def ar_mean(numbers: List[float]): |
||||
return float(sum(numbers)) / max(len(numbers), 1) |
||||
|
||||
def get_av_model(patterns_list: list): |
||||
if not patterns_list: return [] |
||||
patterns_list = get_same_length(patterns_list) |
||||
value_list = list(map(list, zip(*patterns_list))) |
||||
return list(map(ar_mean, value_list)) |
||||
|
||||
def get_same_length(patterns_list: list): |
||||
for index in range(len(patterns_list)): |
||||
if type(patterns_list[index]) == pd.Series: |
||||
patterns_list[index] = patterns_list[index].tolist() |
||||
patterns_list = list(filter(None, patterns_list)) |
||||
max_length = max(map(len, patterns_list)) |
||||
for pat in patterns_list: |
||||
if len(pat) < max_length: |
||||
length_difference = max_length - len(pat) |
||||
added_values = list(0 for _ in range(length_difference)) |
||||
pat.extend(added_values) |
||||
return patterns_list |
||||
|
||||
def close_filtering(pattern_list: List[int], win_size: int) -> TimeSeries: |
||||
if len(pattern_list) == 0: |
||||
return [] |
||||
s = [[pattern_list[0]]] |
||||
k = 0 |
||||
for i in range(1, len(pattern_list)): |
||||
if pattern_list[i] - win_size <= s[k][-1]: |
||||
s[k].append(pattern_list[i]) |
||||
else: |
||||
k += 1 |
||||
s.append([pattern_list[i]]) |
||||
return s |
||||
|
||||
def merge_intersecting_segments(segments: List[Segment], time_step: int) -> List[Segment]: |
||||
''' |
||||
Find intersecting segments in segments list and merge it. |
||||
''' |
||||
if len(segments) < 2: |
||||
return segments |
||||
segments = sorted(segments, key = lambda segment: segment.from_timestamp) |
||||
previous_segment = segments[0] |
||||
for i in range(1, len(segments)): |
||||
if segments[i].from_timestamp <= previous_segment.to_timestamp + time_step: |
||||
segments[i].message = segments[-1].message |
||||
segments[i].from_timestamp = min(previous_segment.from_timestamp, segments[i].from_timestamp) |
||||
segments[i].to_timestamp = max(previous_segment.to_timestamp, segments[i].to_timestamp) |
||||
segments[i - 1] = None |
||||
previous_segment = segments[i] |
||||
segments = [x for x in segments if x is not None] |
||||
return segments |
||||
|
||||
def find_interval(dataframe: pd.DataFrame) -> int: |
||||
if len(dataframe) < 2: |
||||
raise ValueError('Can`t find interval: length of data must be at least 2') |
||||
delta = utils.convert_pd_timestamp_to_ms(dataframe.timestamp[1]) - utils.convert_pd_timestamp_to_ms(dataframe.timestamp[0]) |
||||
return delta |
||||
|
||||
def get_start_and_end_of_segments(segments: List[List[int]]) -> TimeSeries: |
||||
''' |
||||
find start and end of segment: [1, 2, 3, 4] -> [1, 4] |
||||
if segment is 1 index - it will be doubled: [7] -> [7, 7] |
||||
''' |
||||
result = [] |
||||
for segment in segments: |
||||
if len(segment) == 0: |
||||
continue |
||||
elif len(segment) > 1: |
||||
segment = [segment[0], segment[-1]] |
||||
else: |
||||
segment = [segment[0], segment[0]] |
||||
result.append(segment) |
||||
return result |
||||
|
||||
def best_pattern(pattern_list: list, data: pd.Series, dir: str) -> list: |
||||
new_pattern_list = [] |
||||
for val in pattern_list: |
||||
max_val = data[val[0]] |
||||
min_val = data[val[0]] |
||||
ind = val[0] |
||||
for i in val: |
||||
if dir == 'max': |
||||
if data[i] > max_val: |
||||
max_val = data[i] |
||||
ind = i |
||||
else: |
||||
if data[i] < min_val: |
||||
min_val = data[i] |
||||
ind = i |
||||
new_pattern_list.append(ind) |
||||
return new_pattern_list |
||||
|
||||
def find_nan_indexes(segment: pd.Series) -> list: |
||||
nan_list = pd.isnull(segment) |
||||
nan_list = np.array(nan_list) |
||||
nan_indexes = np.where(nan_list == True)[0] |
||||
return list(nan_indexes) |
||||
|
||||
def check_nan_values(segment: Union[pd.Series, list]) -> Union[pd.Series, list]: |
||||
nan_list = utils.find_nan_indexes(segment) |
||||
if len(nan_list) > 0: |
||||
segment = utils.nan_to_zero(segment, nan_list) |
||||
return segment |
||||
|
||||
def nan_to_zero(segment: Union[pd.Series, list], nan_list: list) -> Union[pd.Series, list]: |
||||
if type(segment) == pd.Series: |
||||
for val in nan_list: |
||||
segment.values[val] = 0 |
||||
else: |
||||
for val in nan_list: |
||||
segment[val] = 0 |
||||
return segment |
||||
|
||||
def find_confidence(segment: pd.Series) -> (float, float): |
||||
segment = utils.check_nan_values(segment) |
||||
segment_min = min(segment) |
||||
segment_max = max(segment) |
||||
height = segment_max - segment_min |
||||
if height: |
||||
return (CONFIDENCE_FACTOR * height, height) |
||||
else: |
||||
return (0, 0) |
||||
|
||||
def find_width(pattern: pd.Series, selector: bool) -> int: |
||||
pattern = pattern.values |
||||
center = utils.find_extremum_index(pattern, selector) |
||||
pattern_left = pattern[:center] |
||||
pattern_right = pattern[center:] |
||||
left_extremum_index = utils.find_last_extremum(pattern_left, selector) |
||||
right_extremum_index = utils.find_extremum_index(pattern_right, not selector) |
||||
left_width = center - left_extremum_index |
||||
right_width = right_extremum_index + 1 |
||||
return right_width + left_width |
||||
|
||||
def find_last_extremum(segment: np.ndarray, selector: bool) -> int: |
||||
segment = segment[::-1] |
||||
first_extremum_ind = find_extremum_index(segment, not selector) |
||||
last_extremum_ind = len(segment) - first_extremum_ind - 1 |
||||
return last_extremum_ind |
||||
|
||||
def find_extremum_index(segment: np.ndarray, selector: bool) -> int: |
||||
if selector: |
||||
return segment.argmax() |
||||
else: |
||||
return segment.argmin() |
||||
|
||||
def get_interval(data: pd.Series, center: int, window_size: int, normalization = False) -> pd.Series: |
||||
""" |
||||
Get an interval with 2*window_size length |
||||
window_size to the left, window_size to the right of center |
||||
If normalization == True - subtract minimum from the interval |
||||
""" |
||||
if center >= len(data): |
||||
logging.warning('Pattern center {} is out of data with len {}'.format(center, len(data))) |
||||
return [] |
||||
left_bound = center - window_size |
||||
right_bound = center + window_size + 1 |
||||
if left_bound < 0: |
||||
left_bound = 0 |
||||
if right_bound > len(data): |
||||
right_bound = len(data) |
||||
result_interval = data[left_bound: right_bound] |
||||
if normalization: |
||||
result_interval = subtract_min_without_nan(result_interval) |
||||
return result_interval |
||||
|
||||
def get_borders_of_peaks(pattern_centers: List[int], data: pd.Series, window_size: int, confidence: float, max_border_factor = 1.0, inverse = False) -> TimeSeries: |
||||
""" |
||||
Find start and end of patterns for peak |
||||
max_border_factor - final border of pattern |
||||
if reverse == True - segments will be inversed (trough -> peak / peak -> trough) |
||||
""" |
||||
if len(pattern_centers) == 0: |
||||
return [] |
||||
border_list = [] |
||||
window_size = math.ceil(max_border_factor * window_size) |
||||
for center in pattern_centers: |
||||
current_pattern = get_interval(data, center, window_size, True) |
||||
if inverse: |
||||
current_pattern = inverse_segment(current_pattern) |
||||
current_pattern = current_pattern - confidence |
||||
left_segment = current_pattern[:window_size] # a.iloc[a.index < center] |
||||
right_segment = current_pattern[window_size:] # a.iloc[a.index >= center] |
||||
left_border = get_end_of_segment(left_segment, descending = False) |
||||
right_border = get_end_of_segment(right_segment) |
||||
border_list.append((left_border, right_border)) |
||||
return border_list |
||||
|
||||
def get_end_of_segment(segment: pd.Series, skip_positive_values = True, descending = True) -> int: |
||||
""" |
||||
Find end of descending or ascending part of pattern |
||||
Allowable error is 1 index |
||||
""" |
||||
if not descending: |
||||
segment = segment.iloc[::-1] |
||||
if len(segment) == 0: |
||||
return 1 |
||||
for idx in range(1, len(segment) - 1): |
||||
if skip_positive_values and segment.values[idx] > 0: |
||||
continue |
||||
if segment.values[idx] >= segment.values[idx - 1]: |
||||
return segment.index[idx - 1] |
||||
return segment.index[-1] |
||||
|
||||
def inverse_segment(segment: pd.Series) -> pd.Series: |
||||
""" |
||||
Сonvert trough to peak and virce versa |
||||
""" |
||||
if len(segment) > 0: |
||||
rev_val = max(segment.values) |
||||
for idx in range(len(segment)): |
||||
segment.values[idx] = math.fabs(segment.values[idx] - rev_val) |
||||
return segment |
||||
|
||||
def subtract_min_without_nan(segment: pd.Series) -> pd.Series: |
||||
if len(segment) == 0: |
||||
return [] |
||||
nan_list = utils.find_nan_indexes(segment) |
||||
if len(nan_list) > 0: |
||||
return segment |
||||
else: |
||||
segment = segment - min(segment) |
||||
return segment |
||||
|
||||
def get_convolve(segments: list, av_model: list, data: pd.Series, window_size: int) -> list: |
||||
labeled_segment = [] |
||||
convolve_list = [] |
||||
for segment in segments: |
||||
labeled_segment = utils.get_interval(data, segment, window_size) |
||||
labeled_segment = utils.subtract_min_without_nan(labeled_segment) |
||||
labeled_segment = utils.check_nan_values(labeled_segment) |
||||
auto_convolve = scipy.signal.fftconvolve(labeled_segment, labeled_segment) |
||||
convolve_segment = scipy.signal.fftconvolve(labeled_segment, av_model) |
||||
if len(auto_convolve) > 0: |
||||
convolve_list.append(max(auto_convolve)) |
||||
if len(convolve_segment) > 0: |
||||
convolve_list.append(max(convolve_segment)) |
||||
return convolve_list |
||||
|
||||
def get_correlation_gen(data: pd.Series, window_size: int, pattern_model: List[float]) -> Generator[float, None, None]: |
||||
#Get a new dataset by correlating between a sliding window in data and pattern_model |
||||
for i in range(window_size, len(data) - window_size): |
||||
watch_data = data[i - window_size: i + window_size + 1] |
||||
correlation = pearsonr(watch_data, pattern_model) |
||||
if len(correlation) > 0: |
||||
yield(correlation[0]) |
||||
|
||||
def get_correlation(segments: list, av_model: list, data: pd.Series, window_size: int) -> list: |
||||
labeled_segment = [] |
||||
correlation_list = [] |
||||
p_value_list = [] |
||||
for segment in segments: |
||||
labeled_segment = utils.get_interval(data, segment, window_size) |
||||
labeled_segment = utils.subtract_min_without_nan(labeled_segment) |
||||
labeled_segment = utils.check_nan_values(labeled_segment) |
||||
if len(labeled_segment) == 0 or len(labeled_segment) != len(av_model): |
||||
continue |
||||
correlation = pearsonr(labeled_segment, av_model) |
||||
if len(correlation) > 1: |
||||
correlation_list.append(correlation[0]) |
||||
p_value_list.append(correlation[1]) |
||||
return correlation_list |
||||
|
||||
def get_distribution_density(segment: pd.Series) -> float: |
||||
segment.dropna(inplace = True) |
||||
if len(segment) < 2 or len(segment.nonzero()[0]) == 0: |
||||
return (0, 0, 0) |
||||
min_jump = min(segment) |
||||
max_jump = max(segment) |
||||
pdf = gaussian_kde(segment) |
||||
x = np.linspace(segment.min() - 1, segment.max() + 1, len(segment)) |
||||
y = pdf(x) |
||||
ax_list = list(zip(x, y)) |
||||
ax_list = np.array(ax_list, np.float32) |
||||
antipeaks_kde = argrelextrema(np.array(ax_list), np.less)[0] |
||||
peaks_kde = argrelextrema(np.array(ax_list), np.greater)[0] |
||||
try: |
||||
min_peak_index = peaks_kde[0] |
||||
segment_min_line = ax_list[min_peak_index, 0] |
||||
max_peak_index = peaks_kde[1] |
||||
segment_max_line = ax_list[max_peak_index, 0] |
||||
segment_median = ax_list[antipeaks_kde[0], 0] |
||||
except IndexError: |
||||
segment_max_line = max_jump * (1 - SHIFT_FACTOR) |
||||
segment_min_line = min_jump * (1 - SHIFT_FACTOR) |
||||
segment_median = (max_jump - min_jump) / 2 + min_jump |
||||
return segment_median, segment_max_line, segment_min_line |
||||
|
||||
def find_parameters(segment_data: pd.Series, segment_from_index: int, pat_type: str) -> [int, float, int]: |
||||
segment = segment_data |
||||
if len(segment_data) > SMOOTHING_FACTOR * 3: |
||||
flat_segment = segment_data.rolling(window = SMOOTHING_FACTOR).mean() |
||||
segment = flat_segment.dropna() |
||||
segment_median, segment_max_line, segment_min_line = utils.get_distribution_density(segment) |
||||
height = 0.95 * (segment_max_line - segment_min_line) |
||||
length = utils.get_pattern_length(segment_data, segment_min_line, segment_max_line, pat_type) |
||||
return height, length |
||||
|
||||
def find_pattern_center(segment_data: pd.Series, segment_from_index: int, pattern_type: str): |
||||
segment_median = utils.get_distribution_density(segment_data)[0] |
||||
cen_ind = utils.pattern_intersection(segment_data.tolist(), segment_median, pattern_type) |
||||
if len(cen_ind) > 0: |
||||
pat_center = cen_ind[0] |
||||
segment_cent_index = pat_center + segment_from_index |
||||
else: |
||||
segment_cent_index = math.ceil((len(segment_data)) / 2) |
||||
return segment_cent_index |
||||
|
||||
def get_pattern_length(segment_data: pd.Series, segment_min_line: float, segment_max_line: float, pat_type: str) -> int: |
||||
# TODO: move function to jump & drop merged model |
||||
segment_max = max(segment_data) |
||||
segment_min = min(segment_data) |
||||
# TODO: use better way |
||||
if segment_min_line <= segment_min: |
||||
segment_min_line = segment_min * (1 + MEASUREMENT_ERROR) |
||||
if segment_max_line >= segment_max: |
||||
segment_max_line = segment_max * (1 - MEASUREMENT_ERROR) |
||||
min_line = [] |
||||
max_line = [] |
||||
for i in range(len(segment_data)): |
||||
min_line.append(segment_min_line) |
||||
max_line.append(segment_max_line) |
||||
min_line = np.array(min_line) |
||||
max_line = np.array(max_line) |
||||
segment_array = np.array(segment_data.tolist()) |
||||
idmin = np.argwhere(np.diff(np.sign(min_line - segment_array)) != 0).reshape(-1) |
||||
idmax = np.argwhere(np.diff(np.sign(max_line - segment_array)) != 0).reshape(-1) |
||||
if len(idmin) > 0 and len(idmax) > 0: |
||||
if pat_type == 'jump': |
||||
result_length = idmax[0] - idmin[-1] + 1 |
||||
elif pat_type == 'drop': |
||||
result_length = idmin[0] - idmax[-1] + 1 |
||||
return result_length if result_length > 0 else 0 |
||||
else: |
||||
return 0 |
||||
|
||||
def pattern_intersection(segment_data: list, median: float, pattern_type: str) -> list: |
||||
center_index = [] |
||||
if pattern_type == 'jump': |
||||
for i in range(1, len(segment_data) - 1): |
||||
if segment_data[i - 1] < median and segment_data[i + 1] > median: |
||||
center_index.append(i) |
||||
elif pattern_type == 'drop': |
||||
for i in range(1, len(segment_data) - 1): |
||||
if segment_data[i - 1] > median and segment_data[i + 1] < median: |
||||
center_index.append(i) |
||||
delete_index = [] |
||||
for i in range(1, len(center_index)): |
||||
if center_index[i] == center_index[i - 1] + 1: |
||||
delete_index.append(i - 1) |
||||
|
||||
return [x for (idx, x) in enumerate(center_index) if idx not in delete_index] |
||||
|
||||
def cut_dataframe(data: pd.DataFrame) -> pd.DataFrame: |
||||
data_min = data['value'].min() |
||||
if not np.isnan(data_min) and data_min > 0: |
||||
data['value'] = data['value'] - data_min |
||||
return data |
||||
|
||||
def get_min_max(array: list, default): |
||||
return float(min(array, default=default)), float(max(array, default=default)) |
||||
|
||||
def remove_duplicates_and_sort(array: list) -> list: |
||||
array = list(frozenset(array)) |
||||
array.sort() |
||||
return array |
@ -1,130 +0,0 @@
|
||||
import asyncio |
||||
import threading |
||||
import zmq |
||||
import zmq.asyncio |
||||
from abc import ABC, abstractmethod |
||||
|
||||
|
||||
# This const defines Thread <-> Actor zmq one-to-one connection |
||||
# We create a seperate zmq context, so zqm address 'inproc://xxx' doesn't matter |
||||
# It is default address and you may want to use AsyncZmqThread another way |
||||
ZMQ_THREAD_ACTOR_ADDR = 'inproc://xxx' |
||||
|
||||
|
||||
# Inherience order (threading.Thread, ABC) is essential. Otherwise it's a MRO error. |
||||
class AsyncZmqThread(threading.Thread, ABC): |
||||
"""Class for wrapping zmq socket into a thread with it's own asyncio event loop |
||||
|
||||
""" |
||||
|
||||
def __init__(self, |
||||
zmq_context: zmq.asyncio.Context, |
||||
zmq_socket_addr: str, |
||||
zmq_socket_type = zmq.PAIR |
||||
): |
||||
super(AsyncZmqThread, self).__init__() |
||||
self._zmq_context = zmq_context # you can use it in child classes |
||||
self.__zmq_socket_addr = zmq_socket_addr |
||||
self.__zmq_socket_type = zmq_socket_type |
||||
self.__asyncio_loop = None |
||||
self.__zmq_socket = None |
||||
|
||||
async def __message_recv_loop(self): |
||||
while True: |
||||
text = await self.__zmq_socket.recv_string() |
||||
asyncio.ensure_future(self._on_message_to_thread(text)) |
||||
|
||||
async def _send_message_from_thread(self, message: str): |
||||
await self.__zmq_socket.send_string(message) |
||||
|
||||
@abstractmethod |
||||
async def _on_message_to_thread(self, message: str): |
||||
"""Override this method to receive messages""" |
||||
|
||||
@abstractmethod |
||||
async def _run_thread(self): |
||||
"""Override this method to do some async work. |
||||
This method uses a separate thread. |
||||
|
||||
You can block yourself here if you don't do any await. |
||||
|
||||
Example: |
||||
|
||||
``` |
||||
async def _run_thread(self): |
||||
i = 0 |
||||
while True: |
||||
await asyncio.sleep(1) |
||||
i += 1 |
||||
await self._send_message_from_thread(f'{self.name}: ping {i}') |
||||
``` |
||||
""" |
||||
|
||||
def run(self): |
||||
self.__asyncio_loop = asyncio.new_event_loop() |
||||
asyncio.set_event_loop(self.__asyncio_loop) |
||||
self.__zmq_socket = self._zmq_context.socket(self.__zmq_socket_type) |
||||
self.__zmq_socket.connect(self.__zmq_socket_addr) |
||||
asyncio.ensure_future(self.__message_recv_loop()) |
||||
self.__asyncio_loop.run_until_complete(self._run_thread()) |
||||
|
||||
# TODO: implement stop signal handling |
||||
|
||||
|
||||
class AsyncZmqActor(AsyncZmqThread): |
||||
"""Threaded and Async Actor model based on ZMQ inproc communication |
||||
|
||||
override following: |
||||
``` |
||||
async def _run_thread(self) |
||||
async def _on_message_to_thread(self, message: str) |
||||
``` |
||||
|
||||
both methods run in actor's thread |
||||
|
||||
you can call `self._send_message_from_thread('txt')` |
||||
|
||||
to receive it later in `self._recv_message_from_thread()`. |
||||
|
||||
Example: |
||||
|
||||
``` |
||||
class MyActor(AsyncZmqActor): |
||||
async def _run_thread(self): |
||||
self.counter = 0 |
||||
# runs in a different thread |
||||
await self._send_message_from_thread('some_txt_message_to_actor') |
||||
|
||||
def async _on_message_to_thread(self, message): |
||||
# runs in Thread-actor |
||||
self.counter++ |
||||
|
||||
asyncZmqActor = MyActor() |
||||
asyncZmqActor.start() |
||||
``` |
||||
""" |
||||
|
||||
def __init__(self): |
||||
super(AsyncZmqActor, self).__init__(zmq.asyncio.Context(), ZMQ_THREAD_ACTOR_ADDR) |
||||
|
||||
self.__actor_socket = self._zmq_context.socket(zmq.PAIR) |
||||
self.__actor_socket.bind(ZMQ_THREAD_ACTOR_ADDR) |
||||
|
||||
async def _put_message_to_thread(self, message: str): |
||||
"""It "sends" `message` to thread, |
||||
|
||||
but we can't await it's `AsyncZmqThread._on_message_to_thread()` |
||||
|
||||
so it's "put", not "send" |
||||
""" |
||||
await self.__actor_socket.send_string(message) |
||||
|
||||
async def _recv_message_from_thread(self) -> str: |
||||
"""Returns next message ``'txt'`` from thread sent by |
||||
|
||||
``AsyncZmqActor._send_message_from_thread('txt')`` |
||||
|
||||
""" |
||||
return await self.__actor_socket.recv_string() |
||||
|
||||
# TODO: implement graceful stopping |
@ -1,63 +0,0 @@
|
||||
from itertools import chain |
||||
import pandas as pd |
||||
import numpy as np |
||||
from typing import Generator |
||||
|
||||
def prepare_data(data: list) -> pd.DataFrame: |
||||
""" |
||||
Takes list |
||||
- converts it into pd.DataFrame, |
||||
- converts 'timestamp' column to pd.Datetime, |
||||
- subtracts min value from the dataset |
||||
""" |
||||
data = pd.DataFrame(data, columns=['timestamp', 'value']) |
||||
data['timestamp'] = pd.to_datetime(data['timestamp'], unit='ms') |
||||
data.fillna(value = np.nan, inplace = True) |
||||
return data |
||||
|
||||
def get_intersected_chunks(data: list, intersection: int, chunk_size: int) -> Generator[list, None, None]: |
||||
""" |
||||
Returns generator that splits dataframe on intersected segments. |
||||
Intersection makes it able to detect pattern that present in dataframe on the border between chunks. |
||||
intersection - length of intersection. |
||||
chunk_size - length of chunk |
||||
""" |
||||
assert chunk_size > 0, 'chunk size must be great than zero' |
||||
assert intersection > 0, 'intersection length must be great than zero' |
||||
|
||||
data_len = len(data) |
||||
|
||||
if data_len <= chunk_size: |
||||
yield data |
||||
return |
||||
|
||||
nonintersected = chunk_size - intersection |
||||
|
||||
offset = 0 |
||||
while True: |
||||
left_values = data_len - offset |
||||
if left_values == 0: |
||||
break |
||||
if left_values <= chunk_size: |
||||
yield data[offset : data_len] |
||||
break |
||||
else: |
||||
yield data[offset: offset + chunk_size] |
||||
offset += min(nonintersected, left_values) |
||||
|
||||
def get_chunks(data: list, chunk_size: int) -> Generator[list, None, None]: |
||||
""" |
||||
Returns generator that splits dataframe on non-intersected segments. |
||||
chunk_size - length of chunk |
||||
""" |
||||
assert chunk_size > 0, 'chunk size must be great than zero' |
||||
|
||||
chunks_iterables = [iter(data)] * chunk_size |
||||
result_chunks = zip(*chunks_iterables) |
||||
partial_chunk_len = len(data) % chunk_size |
||||
|
||||
if partial_chunk_len != 0: |
||||
result_chunks = chain(result_chunks, [data[-partial_chunk_len:]]) |
||||
|
||||
for chunk in result_chunks: |
||||
yield list(chunk) |
@ -1,81 +0,0 @@
|
||||
from inspect import signature, Parameter |
||||
from functools import wraps |
||||
from typing import Optional, List |
||||
import re |
||||
|
||||
|
||||
CAMEL_REGEX = re.compile(r'([A-Z])') |
||||
UNDERSCORE_REGEX = re.compile(r'_([a-z])') |
||||
|
||||
def camel_to_underscore(name): |
||||
#TODO: need to rename 'from'/'to' to 'from_timestamp'/'to_timestamp' everywhere(in analytics, server, panel) |
||||
if name == 'from' or name == 'to': |
||||
name += '_timestamp' |
||||
return CAMEL_REGEX.sub(lambda x: '_' + x.group(1).lower(), name) |
||||
|
||||
def underscore_to_camel(name): |
||||
if name == 'from_timestamp' or name == 'to_timestamp': |
||||
name = name.replace('_timestamp', '') |
||||
return UNDERSCORE_REGEX.sub(lambda x: x.group(1).upper(), name) |
||||
|
||||
def is_field_private(field_name: str) -> Optional[str]: |
||||
m = re.match(r'_[^(__)]+__', field_name) |
||||
return m is not None |
||||
|
||||
def serialize(obj): |
||||
if hasattr(obj, 'to_json') == True: |
||||
return obj.to_json() |
||||
else: |
||||
return obj |
||||
|
||||
def inited_params(target_init): |
||||
target_params = signature(target_init).parameters.values() |
||||
if len(target_params) < 1: |
||||
raise ValueError('init function mush have at least self parameter') |
||||
if len(target_params) == 1: |
||||
return target_init |
||||
_, *target_params = target_params # we will not use self any more |
||||
|
||||
@wraps(target_init) |
||||
def wrapped_init(wrapped_self, *wrapped_args, **wrapped_kwargs): |
||||
for tp in target_params: |
||||
if tp.default is Parameter.empty: |
||||
continue |
||||
setattr(wrapped_self, tp.name, tp.default) |
||||
|
||||
for tp, v in zip(target_params, wrapped_args): |
||||
setattr(wrapped_self, tp.name, v) |
||||
|
||||
for k, v in wrapped_kwargs.items(): |
||||
setattr(wrapped_self, k, v) |
||||
|
||||
target_init(wrapped_self, *wrapped_args, **wrapped_kwargs) |
||||
|
||||
return wrapped_init |
||||
|
||||
def JSONClass(target_class): |
||||
|
||||
def to_json(self) -> dict: |
||||
""" |
||||
returns a json representation of the class |
||||
where all None - values and private fileds are skipped |
||||
""" |
||||
return { |
||||
underscore_to_camel(k): serialize(v) for k, v in self.__dict__.items() |
||||
if v is not None and not is_field_private(k) |
||||
} |
||||
|
||||
def from_json(json_object: Optional[dict]) -> target_class: |
||||
if json_object is None: |
||||
json_object = {} |
||||
init_object = { camel_to_underscore(k): v for k, v in json_object.items() } |
||||
return target_class(**init_object) |
||||
|
||||
# target_class.__init__ = inited_params(target_class.__init__) |
||||
target_class.to_json = to_json |
||||
target_class.from_json = from_json |
||||
return target_class |
||||
|
||||
class SerializableList(List[dict]): |
||||
def to_json(self): |
||||
return list(map(lambda s: s.to_json(), self)) |
@ -1,13 +0,0 @@
|
||||
import pandas as pd |
||||
from typing import List |
||||
|
||||
def convert_sec_to_ms(sec) -> int: |
||||
return int(sec) * 1000 |
||||
|
||||
def convert_pd_timestamp_to_ms(timestamp: pd.Timestamp) -> int: |
||||
# TODO: convert from nanoseconds to millisecond in a better way: not by dividing by 10^6 |
||||
return int(timestamp.value) // 1000000 |
||||
|
||||
def convert_series_to_timestamp_list(series: pd.Series) -> List[int]: |
||||
timestamps = map(lambda value: convert_pd_timestamp_to_ms(value), series) |
||||
return list(timestamps) |
@ -1,32 +0,0 @@
|
||||
#!/usr/bin/env python3 |
||||
|
||||
import sys |
||||
import os |
||||
|
||||
if sys.version_info[:3] < (3, 6, 5) or sys.version_info[:2] >= (3, 7): |
||||
sys.stderr.write('Required python is >= 3.6.5 and < 3.7.0 \n') |
||||
sys.stderr.write('Your python version is: %d.%d.%d\n' % sys.version_info[:3]) |
||||
sys.exit(1) |
||||
|
||||
# #TODO: make wrapper script that set PYTHONPATH instead |
||||
sys.path.append(os.path.join(os.path.dirname(__file__), '..', 'analytics')) |
||||
|
||||
import logging |
||||
|
||||
root_logger = logging.getLogger() |
||||
root_logger.setLevel(logging.DEBUG) |
||||
|
||||
|
||||
logging_formatter = logging.Formatter("%(asctime)s [Analytics] [%(levelname)-5.5s] %(message)s") |
||||
|
||||
logging_handler = logging.StreamHandler(sys.stdout) |
||||
logging_handler.setLevel(logging.DEBUG) |
||||
logging_handler.setFormatter(logging_formatter) |
||||
|
||||
root_logger.addHandler(logging_handler) |
||||
|
||||
|
||||
from server import run_server |
||||
|
||||
if __name__ == "__main__": |
||||
run_server() |
@ -1 +0,0 @@
|
||||
hiddenimports=['pandas._libs.tslibs.timedeltas'] |
@ -1 +0,0 @@
|
||||
hiddenimports=['scipy._lib.messagestream'] |
@ -1,7 +0,0 @@
|
||||
attrdict==2.0.0 |
||||
aiounittest==1.1.0 |
||||
numpy==1.14.5 |
||||
pandas==0.20.3 |
||||
pyzmq==18.0.1 |
||||
scipy==1.1.0 |
||||
websockets==8.1 |
@ -1,3 +0,0 @@
|
||||
#!/bin/bash |
||||
cd .. |
||||
python3.6 -m PyInstaller --paths=analytics/ --additional-hooks-dir=pyinstaller_hooks bin/server |
@ -1,4 +0,0 @@
|
||||
import sys |
||||
import os |
||||
|
||||
sys.path.append(os.path.join(os.path.dirname(__file__), '..', 'analytics')) |
@ -1,16 +0,0 @@
|
||||
from analytic_types import TimeSeriesIndex, TimeSeries2 |
||||
|
||||
import unittest |
||||
|
||||
|
||||
class TestDataset(unittest.TestCase): |
||||
def test_basic_timeseries_index(self): |
||||
tsi = TimeSeriesIndex(['2017-12-31 16:00:00-08:00']) |
||||
self.assertEqual(len(tsi), 1) |
||||
tsi2 = TimeSeriesIndex(['2017-12-31 16:00:00-08:00', '2017-12-31 17:00:00-08:00', '2017-12-31 18:00:00-08:00']) |
||||
self.assertEqual(len(tsi2), 3) |
||||
|
||||
def test_basic_timeseries(self): |
||||
tsis = TimeSeriesIndex(['2017-12-31 16:00:00-08:00', '2017-12-31 17:00:00-08:00', '2017-12-31 18:00:00-08:00']) |
||||
ts = TimeSeries2([4, 5, 6], tsis) |
||||
self.assertEqual(len(ts), 3) |
@ -1,38 +0,0 @@
|
||||
import unittest |
||||
import pandas as pd |
||||
import random |
||||
from typing import List |
||||
|
||||
from analytic_types.data_bucket import DataBucket |
||||
from tests.test_dataset import create_list_of_timestamps |
||||
|
||||
class TestBucket(unittest.TestCase): |
||||
|
||||
def test_receive_data(self): |
||||
bucket = DataBucket() |
||||
data_val = list(range(6)) |
||||
timestamp_list = create_list_of_timestamps(len(data_val)) |
||||
for val in data_val: |
||||
bucket.receive_data(get_pd_dataframe([val], [1523889000000 + val])) |
||||
for idx, row in bucket.data.iterrows(): |
||||
self.assertEqual(data_val[idx], row['value']) |
||||
self.assertEqual(timestamp_list[idx], row['timestamp']) |
||||
|
||||
def test_drop_data(self): |
||||
bucket = DataBucket() |
||||
data_val = list(range(10)) |
||||
timestamp_list = create_list_of_timestamps(len(data_val)) |
||||
bucket.receive_data(get_pd_dataframe(data_val, timestamp_list)) |
||||
bucket.drop_data(5) |
||||
expected_data = data_val[5:] |
||||
expected_timestamp = timestamp_list[5:] |
||||
self.assertEqual(expected_data, bucket.data['value'].tolist()) |
||||
self.assertEqual(expected_timestamp, bucket.data['timestamp'].tolist()) |
||||
|
||||
if __name__ == '__main__': |
||||
unittest.main() |
||||
|
||||
def get_pd_dataframe(value: List[int], timestamp: List[int]) -> pd.DataFrame: |
||||
if len(value) != len(timestamp): |
||||
raise ValueError(f'len(value) should be equal to len(timestamp)') |
||||
return pd.DataFrame({ 'value': value, 'timestamp': timestamp }) |
@ -1,386 +0,0 @@
|
||||
import unittest |
||||
import pandas as pd |
||||
import numpy as np |
||||
from utils import prepare_data |
||||
import models |
||||
import random |
||||
import scipy.signal |
||||
from typing import List |
||||
|
||||
from analytic_types.segment import Segment |
||||
|
||||
class TestDataset(unittest.TestCase): |
||||
|
||||
def test_models_with_corrupted_dataframe(self): |
||||
data = [[1523889000000 + i, float('nan')] for i in range(10)] |
||||
dataframe = pd.DataFrame(data, columns=['timestamp', 'value']) |
||||
segments = [] |
||||
|
||||
model_instances = [ |
||||
models.JumpModel(), |
||||
models.DropModel(), |
||||
models.GeneralModel(), |
||||
models.PeakModel(), |
||||
models.TroughModel() |
||||
] |
||||
|
||||
for model in model_instances: |
||||
model_name = model.__class__.__name__ |
||||
model.state = model.get_state(None) |
||||
with self.assertRaises(AssertionError): |
||||
model.fit(dataframe, segments, 'test') |
||||
|
||||
def test_peak_antisegments(self): |
||||
data_val = [1.0, 1.0, 1.0, 2.0, 3.0, 2.0, 1.0, 1.0, 1.0, 1.0, 5.0, 7.0, 5.0, 1.0, 1.0, 1.0, 1.0, 1.0, 1.0, 1.0] |
||||
dataframe = create_dataframe(data_val) |
||||
segments = [{'_id': 'Esl7uetLhx4lCqHa', 'analyticUnitId': 'opnICRJwOmwBELK8', 'from': 1523889000010, 'to': 1523889000012, 'labeled': True, 'deleted': False}, |
||||
{'_id': 'Esl7uetLhx4lCqHa', 'analyticUnitId': 'opnICRJwOmwBELK8', 'from': 1523889000003, 'to': 1523889000005, 'labeled': False, 'deleted': True}] |
||||
segments = [Segment.from_json(segment) for segment in segments] |
||||
|
||||
try: |
||||
model = models.PeakModel() |
||||
model_name = model.__class__.__name__ |
||||
model.state = model.get_state(None) |
||||
model.fit(dataframe, segments, 'test') |
||||
except ValueError: |
||||
self.fail('Model {} raised unexpectedly'.format(model_name)) |
||||
|
||||
def test_jump_antisegments(self): |
||||
data_val = [1.0, 1.0, 1.0, 1.0, 1.0, 5.0, 5.0, 5.0, 5.0, 1.0, 1.0, 1.0, 1.0, 9.0, 9.0, 9.0, 9.0, 9.0, 1.0, 1.0] |
||||
dataframe = create_dataframe(data_val) |
||||
segments = [{'_id': 'Esl7uetLhx4lCqHa', 'analyticUnitId': 'opnICRJwOmwBELK8', 'from': 1523889000010, 'to': 1523889000016, 'labeled': True, 'deleted': False}, |
||||
{'_id': 'Esl7uetLhx4lCqHa', 'analyticUnitId': 'opnICRJwOmwBELK8', 'from': 1523889000002, 'to': 1523889000008, 'labeled': False, 'deleted': True}] |
||||
segments = [Segment.from_json(segment) for segment in segments] |
||||
|
||||
try: |
||||
model = models.JumpModel() |
||||
model_name = model.__class__.__name__ |
||||
model.state = model.get_state(None) |
||||
model.fit(dataframe, segments, 'test') |
||||
except ValueError: |
||||
self.fail('Model {} raised unexpectedly'.format(model_name)) |
||||
|
||||
def test_trough_antisegments(self): |
||||
data_val = [9.0, 9.0, 9.0, 9.0, 7.0, 4.0, 7.0, 9.0, 9.0, 9.0, 5.0, 1.0, 5.0, 9.0, 9.0, 9.0, 9.0, 9.0, 9.0, 9.0] |
||||
dataframe = create_dataframe(data_val) |
||||
segments = [{'_id': 'Esl7uetLhx4lCqHa', 'analyticUnitId': 'opnICRJwOmwBELK8', 'from': 1523889000010, 'to': 1523889000012, 'labeled': True, 'deleted': False}, |
||||
{'_id': 'Esl7uetLhx4lCqHa', 'analyticUnitId': 'opnICRJwOmwBELK8', 'from': 1523889000003, 'to': 1523889000005, 'labeled': False, 'deleted': True}] |
||||
segments = [Segment.from_json(segment) for segment in segments] |
||||
|
||||
try: |
||||
model = models.TroughModel() |
||||
model_name = model.__class__.__name__ |
||||
model.state = model.get_state(None) |
||||
model.fit(dataframe, segments, 'test') |
||||
except ValueError: |
||||
self.fail('Model {} raised unexpectedly'.format(model_name)) |
||||
|
||||
def test_drop_antisegments(self): |
||||
data_val = [9.0, 9.0, 9.0, 9.0, 9.0, 5.0, 5.0, 5.0, 5.0, 9.0, 9.0, 9.0, 9.0, 1.0, 1.0, 1.0, 1.0, 1.0, 9.0, 9.0] |
||||
dataframe = create_dataframe(data_val) |
||||
segments = [{'_id': 'Esl7uetLhx4lCqHa', 'analyticUnitId': 'opnICRJwOmwBELK8', 'from': 1523889000010, 'to': 1523889000016, 'labeled': True, 'deleted': False}, |
||||
{'_id': 'Esl7uetLhx4lCqHa', 'analyticUnitId': 'opnICRJwOmwBELK8', 'from': 1523889000002, 'to': 1523889000008, 'labeled': False, 'deleted': True}] |
||||
segments = [Segment.from_json(segment) for segment in segments] |
||||
|
||||
try: |
||||
model = models.DropModel() |
||||
model_name = model.__class__.__name__ |
||||
model.state = model.get_state(None) |
||||
model.fit(dataframe, segments, 'test') |
||||
except ValueError: |
||||
self.fail('Model {} raised unexpectedly'.format(model_name)) |
||||
|
||||
def test_general_antisegments(self): |
||||
data_val = [1.0, 2.0, 1.0, 2.0, 5.0, 6.0, 3.0, 2.0, 1.0, 1.0, 8.0, 9.0, 8.0, 1.0, 2.0, 3.0, 2.0, 1.0, 1.0, 2.0] |
||||
dataframe = create_dataframe(data_val) |
||||
segments = [{'_id': 'Esl7uetLhx4lCqHa', 'analyticUnitId': 'opnICRJwOmwBELK8', 'from': 1523889000010, 'to': 1523889000012, 'labeled': True, 'deleted': False}, |
||||
{'_id': 'Esl7uetLhx4lCqHa', 'analyticUnitId': 'opnICRJwOmwBELK8', 'from': 1523889000003, 'to': 1523889000005, 'labeled': False, 'deleted': True}] |
||||
segments = [Segment.from_json(segment) for segment in segments] |
||||
|
||||
try: |
||||
model = models.GeneralModel() |
||||
model_name = model.__class__.__name__ |
||||
model.state = model.get_state(None) |
||||
model.fit(dataframe, segments, 'test') |
||||
except ValueError: |
||||
self.fail('Model {} raised unexpectedly'.format(model_name)) |
||||
|
||||
def test_jump_empty_segment(self): |
||||
data_val = [1.0, 1.0, 1.0, 1.0, 1.0, 5.0, 5.0, 5.0, 5.0, 1.0, 1.0, 1.0, 1.0, 9.0, 9.0, 9.0, 9.0, 0, 0, 0, 0, 0, 0, 0, 0, 0] |
||||
dataframe = create_dataframe(data_val) |
||||
segments = [{'_id': 'Esl7uetLhx4lCqHa', 'analyticUnitId': 'opnICRJwOmwBELK8', 'from': 1523889000019, 'to': 1523889000025, 'labeled': True, 'deleted': False}, |
||||
{'_id': 'Esl7uetLhx4lCqHa', 'analyticUnitId': 'opnICRJwOmwBELK8', 'from': 1523889000002, 'to': 1523889000008, 'labeled': True, 'deleted': False}] |
||||
segments = [Segment.from_json(segment) for segment in segments] |
||||
|
||||
try: |
||||
model = models.JumpModel() |
||||
model_name = model.__class__.__name__ |
||||
model.state = model.get_state(None) |
||||
model.fit(dataframe, segments, 'test') |
||||
except ValueError: |
||||
self.fail('Model {} raised unexpectedly'.format(model_name)) |
||||
|
||||
def test_drop_empty_segment(self): |
||||
data_val = [1.0, 1.0, 1.0, 1.0, 1.0, 5.0, 5.0, 5.0, 5.0, 1.0, 1.0, 1.0, 1.0, 9.0, 9.0, 9.0, 9.0, 0, 0, 0, 0, 0, 0, 0, 0, 0] |
||||
dataframe = create_dataframe(data_val) |
||||
segments = [{'_id': 'Esl7uetLhx4lCqHa', 'analyticUnitId': 'opnICRJwOmwBELK8', 'from': 1523889000019, 'to': 1523889000025, 'labeled': True, 'deleted': False}, |
||||
{'_id': 'Esl7uetLhx4lCqHa', 'analyticUnitId': 'opnICRJwOmwBELK8', 'from': 1523889000002, 'to': 1523889000008, 'labeled': True, 'deleted': False}] |
||||
segments = [Segment.from_json(segment) for segment in segments] |
||||
|
||||
try: |
||||
model = models.DropModel() |
||||
model.state = model.get_state(None) |
||||
model_name = model.__class__.__name__ |
||||
model.fit(dataframe, segments, 'test') |
||||
except ValueError: |
||||
self.fail('Model {} raised unexpectedly'.format(model_name)) |
||||
|
||||
def test_value_error_dataset_input_should_have_multiple_elements(self): |
||||
data_val = [1.0, 1.0, 1.0, 1.0, 1.0, 1.0, 1.0, 1.0, 5.0, 5.0, 4.0, 5.0, 5.0, 6.0, 5.0, 1.0, 2.0, 3.0, 4.0, 5.0,3.0,3.0,2.0,7.0,8.0,9.0,8.0,7.0,6.0] |
||||
dataframe = create_dataframe(data_val) |
||||
segments = [{'_id': 'Esl7uetLhx4lCqHa', 'analyticUnitId': 'opnICRJwOmwBELK8', 'from': 1523889000007, 'to': 1523889000011, 'labeled': True, 'deleted': False}] |
||||
segments = [Segment.from_json(segment) for segment in segments] |
||||
|
||||
try: |
||||
model = models.JumpModel() |
||||
model.state = model.get_state(None) |
||||
model_name = model.__class__.__name__ |
||||
model.fit(dataframe, segments, 'test') |
||||
except ValueError: |
||||
self.fail('Model {} raised unexpectedly'.format(model_name)) |
||||
|
||||
def test_prepare_data_for_nonetype(self): |
||||
data = [[1523889000000, None], [1523889000001, None], [1523889000002, None]] |
||||
try: |
||||
data = prepare_data(data) |
||||
except ValueError: |
||||
self.fail('Model {} raised unexpectedly'.format(model_name)) |
||||
|
||||
def test_prepare_data_for_nan(self): |
||||
data = [[1523889000000, np.nan], [1523889000001, np.nan], [1523889000002, np.nan]] |
||||
try: |
||||
data = prepare_data(data) |
||||
except ValueError: |
||||
self.fail('Model {} raised unexpectedly'.format(model_name)) |
||||
|
||||
def test_prepare_data_output_fon_nan(self): |
||||
data_nan = [[1523889000000, np.nan], [1523889000001, np.nan], [1523889000002, np.nan]] |
||||
data_none = [[1523889000000, None], [1523889000001, None], [1523889000002, None]] |
||||
return_data_nan = prepare_data(data_nan) |
||||
return_data_none = prepare_data(data_none) |
||||
for item in return_data_nan.value: |
||||
self.assertTrue(np.isnan(item)) |
||||
for item in return_data_none.value: |
||||
self.assertTrue(np.isnan(item)) |
||||
|
||||
def test_three_value_segment(self): |
||||
data_val = [1.0, 1.0, 1.0, 1.0, 1.0, 5.0, 2.0, 5.0, 5.0, 1.0, 1.0, 1.0, 1.0, 9.0, 9.0, 9.0, 9.0, 2.0, 3.0, 4.0, 5.0, 4.0, 2.0, 1.0, 3.0, 4.0] |
||||
dataframe = create_dataframe(data_val) |
||||
segments = [{'_id': 'Esl7uetLhx4lCqHa', 'analyticUnitId': 'opnICRJwOmwBELK8', 'from': 1523889000004, 'to': 1523889000006, 'labeled': True, 'deleted': False}] |
||||
segments = [Segment.from_json(segment) for segment in segments] |
||||
|
||||
model_instances = [ |
||||
models.GeneralModel(), |
||||
models.PeakModel(), |
||||
] |
||||
try: |
||||
for model in model_instances: |
||||
model_name = model.__class__.__name__ |
||||
model.state = model.get_state(None) |
||||
model.fit(dataframe, segments, 'test') |
||||
except ValueError: |
||||
self.fail('Model {} raised unexpectedly'.format(model_name)) |
||||
|
||||
def test_general_for_two_labeling(self): |
||||
data_val = [1.0, 2.0, 5.0, 2.0, 1.0, 1.0, 3.0, 6.0, 4.0, 2.0, 1.0, 0, 0] |
||||
dataframe = create_dataframe(data_val) |
||||
segments = [{'_id': 'Esl7uetLhx4lCqHa', 'analyticUnitId': 'opnICRJwOmwBELK8', 'from': 1523889000001, 'to': 1523889000003, 'labeled': True, 'deleted': False}] |
||||
segments = [Segment.from_json(segment) for segment in segments] |
||||
|
||||
model = models.GeneralModel() |
||||
model.state = model.get_state(None) |
||||
model.fit(dataframe, segments,'test') |
||||
result = len(data_val) + 1 |
||||
for _ in range(2): |
||||
model.do_detect(dataframe) |
||||
max_pattern_index = max(model.do_detect(dataframe)) |
||||
self.assertLessEqual(max_pattern_index[0], result) |
||||
|
||||
|
||||
def test_peak_model_for_cache(self): |
||||
cache = { |
||||
'patternCenter': [1, 6], |
||||
'patternModel': [1, 4, 0], |
||||
'confidence': 2, |
||||
'convolveMax': 8, |
||||
'convolveMin': 7, |
||||
'windowSize': 1, |
||||
'convDelMin': 0, |
||||
'convDelMax': 0, |
||||
'heightMax': 4, |
||||
'heightMin': 4, |
||||
} |
||||
data_val = [2.0, 5.0, 1.0, 1.0, 1.0, 2.0, 5.0, 1.0, 1.0, 2.0, 3.0, 7.0, 1.0, 1.0, 1.0] |
||||
dataframe = create_dataframe(data_val) |
||||
segments = [{'_id': 'Esl7uetLhx4lCqHa', 'analyticUnitId': 'opnICRJwOmwBELK8', 'from': 1523889000010, 'to': 1523889000012, 'labeled': True, 'deleted': False}] |
||||
segments = [Segment.from_json(segment) for segment in segments] |
||||
|
||||
model = models.PeakModel() |
||||
model.state = model.get_state(cache) |
||||
result = model.fit(dataframe, segments, 'test') |
||||
self.assertEqual(len(result.pattern_center), 3) |
||||
|
||||
def test_trough_model_for_cache(self): |
||||
cache = { |
||||
'patternCenter': [2, 6], |
||||
'patternModel': [5, 0.5, 4], |
||||
'confidence': 2, |
||||
'convolveMax': 8, |
||||
'convolveMin': 7, |
||||
'window_size': 1, |
||||
'convDelMin': 0, |
||||
'convDelMax': 0, |
||||
} |
||||
data_val = [5.0, 5.0, 1.0, 4.0, 5.0, 5.0, 0.0, 4.0, 5.0, 5.0, 6.0, 1.0, 5.0, 5.0, 5.0] |
||||
dataframe = create_dataframe(data_val) |
||||
segments = [{'_id': 'Esl7uetLhx4lCqHa', 'analyticUnitId': 'opnICRJwOmwBELK8', 'from': 1523889000010, 'to': 1523889000012, 'labeled': True, 'deleted': False}] |
||||
segments = [Segment.from_json(segment) for segment in segments] |
||||
|
||||
model = models.TroughModel() |
||||
model.state = model.get_state(cache) |
||||
result = model.fit(dataframe, segments, 'test') |
||||
self.assertEqual(len(result.pattern_center), 3) |
||||
|
||||
def test_jump_model_for_cache(self): |
||||
cache = { |
||||
'patternCenter': [2, 6], |
||||
'patternModel': [5, 0.5, 4], |
||||
'confidence': 2, |
||||
'convolveMax': 8, |
||||
'convolveMin': 7, |
||||
'window_size': 1, |
||||
'convDelMin': 0, |
||||
'convDelMax': 0, |
||||
} |
||||
data_val = [1.0, 1.0, 1.0, 4.0, 4.0, 0.0, 0.0, 5.0, 5.0, 0.0, 0.0, 4.0, 4.0, 4.0, 4.0] |
||||
dataframe = create_dataframe(data_val) |
||||
segments = [{'_id': 'Esl7uetLhx4lCqHa', 'analyticUnitId': 'opnICRJwOmwBELK8', 'from': 152388900009, 'to': 1523889000013, 'labeled': True, 'deleted': False}] |
||||
segments = [Segment.from_json(segment) for segment in segments] |
||||
|
||||
model = models.JumpModel() |
||||
model.state = model.get_state(cache) |
||||
result = model.fit(dataframe, segments, 'test') |
||||
self.assertEqual(len(result.pattern_center), 3) |
||||
|
||||
def test_models_for_pattern_model_cache(self): |
||||
cache = { |
||||
'patternCenter': [4, 12], |
||||
'patternModel': [], |
||||
'confidence': 2, |
||||
'convolveMax': 8, |
||||
'convolveMin': 7, |
||||
'window_size': 2, |
||||
'convDelMin': 0, |
||||
'convDelMax': 0, |
||||
} |
||||
data_val = [5.0, 5.0, 5.0, 5.0, 1.0, 1.0, 1.0, 1.0, 9.0, 9.0, 9.0, 9.0, 0, 0, 0, 0, 0, 0, 6.0, 6.0, 6.0, 1.0, 1.0, 1.0, 1.0, 1.0] |
||||
dataframe = create_dataframe(data_val) |
||||
segments = [{'_id': 'Esl7uetLhx4lCqHa', 'analyticUnitId': 'opnICRJwOmwBELK8', 'from': 1523889000019, 'to': 1523889000024, 'labeled': True, 'deleted': False}] |
||||
segments = [Segment.from_json(segment) for segment in segments] |
||||
|
||||
try: |
||||
model = models.DropModel() |
||||
model_name = model.__class__.__name__ |
||||
model.state = model.get_state(cache) |
||||
model.fit(dataframe, segments, 'test') |
||||
except ValueError: |
||||
self.fail('Model {} raised unexpectedly'.format(model_name)) |
||||
|
||||
def test_problem_data_for_random_model(self): |
||||
problem_data = [2.0, 3.0, 3.0, 3.0, 2.0, 2.0, 2.0, 2.0, 2.0, 2.0, 2.0, 2.0, 2.0, 2.0, 2.0, 2.0, 2.0, 2.0, 2.0, 2.0, 3.0, 3.0, 3.0, |
||||
3.0, 3.0, 3.0, 5.0, 5.0, 5.0, 5.0, 2.0, 2.0, 2.0, 2.0, 3.0, 3.0, 3.0, 3.0, 3.0, 3.0, 2.0, 3.0, 3.0, 3.0, 3.0, 3.0, 3.0, 3.0, 3.0, 3.0, 3.0, |
||||
3.0, 3.0, 2.0, 2.0, 2.0, 2.0, 3.0, 3.0, 3.0, 3.0, 3.0, 3.0, 3.0, 3.0, 2.0, 2.0, 2.0, 6.0, 7.0, 8.0, 8.0, 4.0, 2.0, 2.0, 3.0, 3.0, 3.0, 4.0, |
||||
4.0, 4.0, 4.0, 3.0, 3.0, 3.0, 3.0, 2.0, 2.0, 2.0, 2.0, 2.0, 2.0, 2.0, 3.0, 3.0, 3.0, 3.0, 3.0, 3.0, 3.0, 2.0, 2.0, 2.0, 2.0, 2.0, 2.0, 3.0, |
||||
4.0, 4.0, 4.0, 4.0, 4.0, 6.0, 5.0, 4.0, 4.0, 3.0, 3.0, 3.0, 4.0, 4.0, 4.0, 4.0, 4.0, 4.0, 4.0, 2.0, 3.0, 3.0, 2.0, 2.0, 2.0, 2.0, 2.0, 2.0, |
||||
2.0, 8.0, 2.0, 2.0, 2.0, 2.0, 2.0, 2.0, 2.0, 2.0, 2.0, 2.0, 2.0, 2.0, 2.0, 2.0, 2.0] |
||||
data = create_dataframe(problem_data) |
||||
cache = { |
||||
'patternCenter': [5, 50], |
||||
'patternModel': [], |
||||
'windowSize': 2, |
||||
'convolveMin': 0, |
||||
'convolveMax': 0, |
||||
'convDelMin': 0, |
||||
'convDelMax': 0, |
||||
} |
||||
max_ws = 20 |
||||
iteration = 1 |
||||
for ws in range(1, max_ws): |
||||
for _ in range(iteration): |
||||
pattern_model = create_random_model(ws) |
||||
convolve = scipy.signal.fftconvolve(pattern_model, pattern_model) |
||||
cache['windowSize'] = ws |
||||
cache['patternModel'] = pattern_model |
||||
cache['convolveMin'] = max(convolve) |
||||
cache['convolveMax'] = max(convolve) |
||||
try: |
||||
model = models.GeneralModel() |
||||
model.state = model.get_state(cache) |
||||
model_name = model.__class__.__name__ |
||||
model.detect(data, 'test') |
||||
except ValueError: |
||||
self.fail('Model {} raised unexpectedly with av_model {} and window size {}'.format(model_name, pattern_model, ws)) |
||||
|
||||
def test_random_dataset_for_random_model(self): |
||||
data = create_random_model(random.randint(1, 100)) |
||||
data = create_dataframe(data) |
||||
model_instances = [ |
||||
models.PeakModel(), |
||||
models.TroughModel() |
||||
] |
||||
cache = { |
||||
'patternCenter': [5, 50], |
||||
'patternModel': [], |
||||
'windowSize': 2, |
||||
'convolveMin': 0, |
||||
'convolveMax': 0, |
||||
'confidence': 0, |
||||
'heightMax': 0, |
||||
'heightMin': 0, |
||||
'convDelMin': 0, |
||||
'convDelMax': 0, |
||||
} |
||||
ws = random.randint(1, int(len(data['value']/2))) |
||||
pattern_model = create_random_model(ws) |
||||
convolve = scipy.signal.fftconvolve(pattern_model, pattern_model) |
||||
confidence = 0.2 * (data['value'].max() - data['value'].min()) |
||||
cache['windowSize'] = ws |
||||
cache['patternModel'] = pattern_model |
||||
cache['convolveMin'] = max(convolve) |
||||
cache['convolveMax'] = max(convolve) |
||||
cache['confidence'] = confidence |
||||
cache['heightMax'] = data['value'].max() |
||||
cache['heightMin'] = confidence |
||||
try: |
||||
for model in model_instances: |
||||
model_name = model.__class__.__name__ |
||||
model.state = model.get_state(cache) |
||||
model.detect(data, 'test') |
||||
except ValueError: |
||||
self.fail('Model {} raised unexpectedly with dataset {} and cache {}'.format(model_name, data['value'], cache)) |
||||
|
||||
if __name__ == '__main__': |
||||
unittest.main() |
||||
|
||||
def create_dataframe(data_val: list) -> pd.DataFrame: |
||||
data_ind = create_list_of_timestamps(len(data_val)) |
||||
data = {'timestamp': data_ind, 'value': data_val} |
||||
dataframe = pd.DataFrame(data) |
||||
dataframe['timestamp'] = pd.to_datetime(dataframe['timestamp'], unit='ms') |
||||
return dataframe |
||||
|
||||
def create_list_of_timestamps(length: int) -> List[int]: |
||||
return [1523889000000 + i for i in range(length)] |
||||
|
||||
def create_random_model(window_size: int) -> list: |
||||
return [random.randint(0, 100) for _ in range(window_size * 2 + 1)] |
@ -1,265 +0,0 @@
|
||||
import unittest |
||||
import pandas as pd |
||||
|
||||
from detectors import pattern_detector, threshold_detector, anomaly_detector |
||||
from analytic_types.detector import DetectionResult, ProcessingResult, Bound |
||||
from analytic_types.segment import Segment |
||||
from tests.test_dataset import create_dataframe, create_list_of_timestamps |
||||
from utils import convert_pd_timestamp_to_ms |
||||
|
||||
class TestPatternDetector(unittest.TestCase): |
||||
|
||||
def test_small_dataframe(self): |
||||
|
||||
data = [[0,1], [1,2]] |
||||
dataframe = pd.DataFrame(data, columns=['timestamp', 'values']) |
||||
cache = { 'windowSize': 10 } |
||||
|
||||
detector = pattern_detector.PatternDetector('GENERAL', 'test_id') |
||||
with self.assertRaises(ValueError): |
||||
detector.detect(dataframe, cache) |
||||
|
||||
def test_only_negative_segments(self): |
||||
data_val = [0, 1, 2, 1, 2, 10, 1, 2, 1] |
||||
data_ind = [1523889000000 + i for i in range(len(data_val))] |
||||
data = {'timestamp': data_ind, 'value': data_val} |
||||
dataframe = pd.DataFrame(data = data) |
||||
segments = [{'_id': 'Esl7uetLhx4lCqHa', 'analyticUnitId': 'opnICRJwOmwBELK8', 'from': 1523889000019, 'to': 1523889000025, 'labeled': False, 'deleted': False}, |
||||
{'_id': 'Esl7uetLhx4lCqHa', 'analyticUnitId': 'opnICRJwOmwBELK8', 'from': 1523889000002, 'to': 1523889000008, 'labeled': False, 'deleted': False}] |
||||
segments = [Segment.from_json(segment) for segment in segments] |
||||
cache = {} |
||||
detector = pattern_detector.PatternDetector('PEAK', 'test_id') |
||||
excepted_error_message = 'test_id has no positive labeled segments. Pattern detector needs at least 1 positive labeled segment' |
||||
|
||||
try: |
||||
detector.train(dataframe, segments, cache) |
||||
except ValueError as e: |
||||
self.assertEqual(str(e), excepted_error_message) |
||||
|
||||
def test_positive_and_negative_segments(self): |
||||
data_val = [1.0, 1.0, 1.0, 2.0, 3.0, 2.0, 1.0, 1.0, 1.0, 1.0, 5.0, 7.0, 5.0, 1.0, 1.0, 1.0, 1.0, 1.0, 1.0, 1.0] |
||||
dataframe = create_dataframe(data_val) |
||||
segments = [{'_id': 'Esl7uetLhx4lCqHa', 'analyticUnitId': 'opnICRJwOmwBELK8', 'from': 1523889000004, 'to': 1523889000006, 'labeled': True, 'deleted': False}, |
||||
{'_id': 'Esl7uetLhx4lCqHa', 'analyticUnitId': 'opnICRJwOmwBELK8', 'from': 1523889000001, 'to': 1523889000003, 'labeled': False, 'deleted': False}] |
||||
segments = [Segment.from_json(segment) for segment in segments] |
||||
cache = {} |
||||
detector = pattern_detector.PatternDetector('PEAK', 'test_id') |
||||
try: |
||||
detector.train(dataframe, segments, cache) |
||||
except Exception as e: |
||||
self.fail('detector.train fail with error {}'.format(e)) |
||||
|
||||
class TestThresholdDetector(unittest.TestCase): |
||||
|
||||
def test_invalid_cache(self): |
||||
|
||||
detector = threshold_detector.ThresholdDetector('test_id') |
||||
|
||||
with self.assertRaises(ValueError): |
||||
detector.detect([], None) |
||||
|
||||
with self.assertRaises(ValueError): |
||||
detector.detect([], {}) |
||||
|
||||
|
||||
class TestAnomalyDetector(unittest.TestCase): |
||||
|
||||
def test_detect(self): |
||||
data_val = [0, 1, 2, 1, 2, 10, 1, 2, 1] |
||||
data_ind = [1523889000000 + i for i in range(len(data_val))] |
||||
data = {'timestamp': data_ind, 'value': data_val} |
||||
dataframe = pd.DataFrame(data = data) |
||||
dataframe['timestamp'] = pd.to_datetime(dataframe['timestamp'], unit='ms') |
||||
cache = { |
||||
'confidence': 2, |
||||
'alpha': 0.1, |
||||
'enableBounds': 'ALL', |
||||
'timeStep': 1 |
||||
} |
||||
detector = anomaly_detector.AnomalyDetector('test_id') |
||||
|
||||
detect_result: DetectionResult = detector.detect(dataframe, cache) |
||||
detected_segments = list(map(lambda s: {'from': s.from_timestamp, 'to': s.to_timestamp}, detect_result.segments)) |
||||
result = [{ 'from': 1523889000005.0, 'to': 1523889000005.0 }] |
||||
self.assertEqual(result, detected_segments) |
||||
|
||||
cache = { |
||||
'confidence': 2, |
||||
'alpha': 0.1, |
||||
'enableBounds': 'ALL', |
||||
'timeStep': 1, |
||||
'seasonality': 4, |
||||
'segments': [{ 'from': 1523889000001, 'to': 1523889000002, 'data': [10] }] |
||||
} |
||||
detect_result: DetectionResult = detector.detect(dataframe, cache) |
||||
detected_segments = list(map(lambda s: {'from': s.from_timestamp, 'to': s.to_timestamp}, detect_result.segments)) |
||||
result = [] |
||||
self.assertEqual(result, detected_segments) |
||||
|
||||
def test_process_data(self): |
||||
data_val = [0, 1, 2, 1, 2, 10, 1, 2, 1] |
||||
data_ind = [1523889000000 + i for i in range(len(data_val))] |
||||
data = {'timestamp': data_ind, 'value': data_val} |
||||
dataframe = pd.DataFrame(data = data) |
||||
dataframe['timestamp'] = pd.to_datetime(dataframe['timestamp'], unit='ms') |
||||
cache = { |
||||
'confidence': 2, |
||||
'alpha': 0.1, |
||||
'enableBounds': 'ALL', |
||||
'timeStep': 1 |
||||
} |
||||
detector = anomaly_detector.AnomalyDetector('test_id') |
||||
detect_result: ProcessingResult = detector.process_data(dataframe, cache) |
||||
expected_result = { |
||||
'lowerBound': [ |
||||
(1523889000000, -2.0), |
||||
(1523889000001, -1.9), |
||||
(1523889000002, -1.71), |
||||
(1523889000003, -1.6389999999999998), |
||||
(1523889000004, -1.4750999999999999), |
||||
(1523889000005, -0.5275899999999998), |
||||
(1523889000006, -0.5748309999999996), |
||||
(1523889000007, -0.5173478999999996), |
||||
(1523889000008, -0.5656131099999995) |
||||
], |
||||
'upperBound': [ |
||||
(1523889000000, 2.0), |
||||
(1523889000001, 2.1), |
||||
(1523889000002, 2.29), |
||||
(1523889000003, 2.361), |
||||
(1523889000004, 2.5249), |
||||
(1523889000005, 3.47241), |
||||
(1523889000006, 3.4251690000000004), |
||||
(1523889000007, 3.4826521), |
||||
(1523889000008, 3.4343868900000007) |
||||
]} |
||||
self.assertEqual(detect_result.to_json(), expected_result) |
||||
|
||||
cache = { |
||||
'confidence': 2, |
||||
'alpha': 0.1, |
||||
'enableBounds': 'ALL', |
||||
'timeStep': 1, |
||||
'seasonality': 5, |
||||
'segments': [{ 'from': 1523889000001, 'to': 1523889000002,'data': [1] }] |
||||
} |
||||
detect_result: ProcessingResult = detector.process_data(dataframe, cache) |
||||
expected_result = { |
||||
'lowerBound': [ |
||||
(1523889000000, -2.0), |
||||
(1523889000001, -2.9), |
||||
(1523889000002, -1.71), |
||||
(1523889000003, -1.6389999999999998), |
||||
(1523889000004, -1.4750999999999999), |
||||
(1523889000005, -0.5275899999999998), |
||||
(1523889000006, -1.5748309999999996), |
||||
(1523889000007, -0.5173478999999996), |
||||
(1523889000008, -0.5656131099999995) |
||||
], |
||||
'upperBound': [ |
||||
(1523889000000, 2.0), |
||||
(1523889000001, 3.1), |
||||
(1523889000002, 2.29), |
||||
(1523889000003, 2.361), |
||||
(1523889000004, 2.5249), |
||||
(1523889000005, 3.47241), |
||||
(1523889000006, 4.425169), |
||||
(1523889000007, 3.4826521), |
||||
(1523889000008, 3.4343868900000007) |
||||
]} |
||||
self.assertEqual(detect_result.to_json(), expected_result) |
||||
|
||||
def test_get_seasonality_offset(self): |
||||
detector = anomaly_detector.AnomalyDetector('test_id') |
||||
from_timestamp = 1573700973027 |
||||
seasonality = 3600000 |
||||
data_start_time = 1573698780000 |
||||
time_step = 30000 |
||||
detected_offset = detector.get_seasonality_offset(from_timestamp, seasonality, data_start_time, time_step) |
||||
expected_offset = 74 |
||||
self.assertEqual(detected_offset, expected_offset) |
||||
|
||||
def test_segment_generator(self): |
||||
detector = anomaly_detector.AnomalyDetector('test_id') |
||||
data = [1, 1, 5, 1, -4, 5, 5, 5, -3, 1] |
||||
timestamps = create_list_of_timestamps(len(data)) |
||||
dataframe = create_dataframe(data) |
||||
upper_bound = pd.Series([2, 2, 2, 2, 2, 2, 2, 2, 2, 2]) |
||||
lower_bound = pd.Series([0, 0, 0, 0, 0, 0, 0, 0, 0, 0]) |
||||
segments = list(detector.detections_generator(dataframe, upper_bound, lower_bound, enabled_bounds=Bound.ALL)) |
||||
|
||||
segments_borders = list(map(lambda s: [s.from_timestamp, s.to_timestamp], segments)) |
||||
self.assertEqual(segments_borders, [[timestamps[2], timestamps[2]], [timestamps[4], timestamps[8]]]) |
||||
|
||||
def test_consume_data(self): |
||||
cache = { |
||||
'confidence': 2, |
||||
'alpha': 0.1, |
||||
'enableBounds': 'ALL', |
||||
'timeStep': 1 |
||||
} |
||||
detector = anomaly_detector.AnomalyDetector('test_id') |
||||
|
||||
detect_result: DetectionResult = None |
||||
for val in range(22): |
||||
value = 1 if val != 10 else 5 |
||||
dataframe = pd.DataFrame({'value': [value], 'timestamp': [1523889000000 + val]}) |
||||
dataframe['timestamp'] = pd.to_datetime(dataframe['timestamp'], unit='ms') |
||||
detect_result = detector.consume_data(dataframe, cache) |
||||
|
||||
detected_segments = list(map(lambda s: {'from': s.from_timestamp, 'to': s.to_timestamp}, detect_result.segments)) |
||||
result = [{ 'from': 1523889000010, 'to': 1523889000010 }] |
||||
self.assertEqual(result, detected_segments) |
||||
|
||||
def test_get_segment_bound(self): |
||||
detector = anomaly_detector.AnomalyDetector('test_id') |
||||
peak_segment = pd.Series([1,2,3,4,3,2,1]) |
||||
trough_segment = pd.Series([4,3,2,1,2,3,4]) |
||||
expected_peak_segment_results = { |
||||
'max_value': 3, |
||||
'min_value': 1.5 |
||||
} |
||||
expected_trough_segment_results = { |
||||
'max_value': 3.5, |
||||
'min_value': 2.75 |
||||
} |
||||
peak_detector_result_upper = detector.get_segment_bound(peak_segment, Bound.UPPER) |
||||
peak_detector_result_lower = detector.get_segment_bound(peak_segment, Bound.LOWER) |
||||
trough_detector_result_upper = detector.get_segment_bound(trough_segment, Bound.UPPER) |
||||
trough_detector_result_lower = detector.get_segment_bound(trough_segment, Bound.LOWER) |
||||
|
||||
self.assertGreaterEqual( |
||||
max(peak_detector_result_upper), |
||||
expected_peak_segment_results['max_value'] |
||||
) |
||||
self.assertLessEqual( |
||||
max(peak_detector_result_lower), |
||||
expected_peak_segment_results['min_value'] |
||||
) |
||||
self.assertGreaterEqual( |
||||
max(trough_detector_result_upper), |
||||
expected_trough_segment_results['max_value'] |
||||
) |
||||
self.assertLessEqual( |
||||
max(trough_detector_result_lower), |
||||
expected_trough_segment_results['min_value'] |
||||
) |
||||
|
||||
def test_get_segment_bound_corner_cases(self): |
||||
detector = anomaly_detector.AnomalyDetector('test_id') |
||||
empty_segment = pd.Series([]) |
||||
same_values_segment = pd.Series([2,2,2,2,2,2]) |
||||
empty_detector_result_upper = detector.get_segment_bound(empty_segment, Bound.UPPER) |
||||
empty_detector_result_lower = detector.get_segment_bound(empty_segment, Bound.LOWER) |
||||
same_values_detector_result_upper = detector.get_segment_bound(same_values_segment, Bound.UPPER) |
||||
same_values_detector_result_lower = detector.get_segment_bound(same_values_segment, Bound.LOWER) |
||||
|
||||
self.assertEqual(len(empty_detector_result_upper), 0) |
||||
self.assertEqual(len(empty_detector_result_lower), 0) |
||||
self.assertEqual(min(same_values_detector_result_upper), 0) |
||||
self.assertEqual(max(same_values_detector_result_upper), 0) |
||||
self.assertEqual(min(same_values_detector_result_lower), 0) |
||||
self.assertEqual(max(same_values_detector_result_lower), 0) |
||||
|
||||
if __name__ == '__main__': |
||||
unittest.main() |
@ -1,100 +0,0 @@
|
||||
from models import PeakModel, DropModel, TroughModel, JumpModel, GeneralModel |
||||
from models import GeneralModelState |
||||
import utils.meta |
||||
import aiounittest |
||||
from analytic_unit_manager import AnalyticUnitManager |
||||
from collections import namedtuple |
||||
|
||||
TestData = namedtuple('TestData', ['uid', 'type', 'values', 'segments']) |
||||
|
||||
def get_random_id() -> str: |
||||
return str(id(list())) |
||||
|
||||
class TestDataset(aiounittest.AsyncTestCase): |
||||
|
||||
timestep = 50 #ms |
||||
|
||||
def _fill_task(self, uid, data, task_type, analytic_unit_type, segments=None, cache=None): |
||||
task = { |
||||
'analyticUnitId': uid, |
||||
'type': task_type, |
||||
'payload': { |
||||
'data': data, |
||||
'from': data[0][0], |
||||
'to': data[-1][0], |
||||
'analyticUnitType': analytic_unit_type, |
||||
'detector': 'pattern', |
||||
'cache': cache |
||||
}, |
||||
'_id': get_random_id() |
||||
} |
||||
if segments: task['payload']['segments'] = segments |
||||
|
||||
return task |
||||
|
||||
def _convert_values(self, values) -> list: |
||||
from_t = 0 |
||||
to_t = len(values) * self.timestep |
||||
return list(zip(range(from_t, to_t, self.timestep), values)) |
||||
|
||||
def _index_to_test_time(self, idx) -> int: |
||||
return idx * self.timestep |
||||
|
||||
def _get_learn_task(self, test_data): |
||||
uid, analytic_unit_type, values, segments = test_data |
||||
data = self._convert_values(values) |
||||
segments = [{ |
||||
'analyticUnitId': uid, |
||||
'from': self._index_to_test_time(s[0]), |
||||
'to': self._index_to_test_time(s[1]), |
||||
'labeled': True, |
||||
'deleted': False |
||||
} for s in segments] |
||||
return self._fill_task(uid, data, 'LEARN', analytic_unit_type, segments=segments) |
||||
|
||||
def _get_detect_task(self, test_data, cache): |
||||
uid, analytic_unit_type, values, _ = test_data |
||||
data = self._convert_values(values) |
||||
return self._fill_task(uid, data, 'DETECT', analytic_unit_type, cache=cache) |
||||
|
||||
def _get_test_dataset(self, pattern) -> tuple: |
||||
""" |
||||
pattern name: ([dataset values], [list of segments]) |
||||
|
||||
segment - (begin, end) - indexes in dataset values |
||||
returns dataset in format (data: List[int], segments: List[List[int]]) |
||||
""" |
||||
datasets = { |
||||
'PEAK': ([0, 0, 1, 2, 3, 4, 3, 2, 1, 0, 0], [[2, 8]]), |
||||
'JUMP': ([0, 0, 1, 2, 3, 4, 4, 4], [[1, 6]]), |
||||
'DROP': ([4, 4, 4, 3, 2, 1, 0, 0], [[1, 6]]), |
||||
'TROUGH': ([4, 4, 3, 2, 1, 0, 1, 2, 3, 4, 4], [[1, 9]]), |
||||
'GENERAL': ([0, 0, 1, 2, 3, 4, 3, 2, 1, 0, 0], [[2, 8]]) |
||||
} |
||||
return datasets[pattern] |
||||
|
||||
async def _learn(self, task, manager=None) -> dict: |
||||
if not manager: manager = AnalyticUnitManager() |
||||
result = await manager.handle_analytic_task(task) |
||||
return result['payload']['cache'] |
||||
|
||||
async def _detect(self, task, manager=None) -> dict: |
||||
if not manager: manager = AnalyticUnitManager() |
||||
result = await manager.handle_analytic_task(task) |
||||
return result |
||||
|
||||
async def _test_detect(self, test_data, manager=None): |
||||
learn_task = self._get_learn_task(test_data) |
||||
cache = await self._learn(learn_task, manager) |
||||
detect_task = self._get_detect_task(test_data, cache) |
||||
result = await self._detect(detect_task, manager) |
||||
return result |
||||
|
||||
async def test_unit_manager(self): |
||||
test_data = TestData(get_random_id(), 'PEAK', [0,1,2,5,10,5,2,1,1,1,0,0,0,0], [[1,7]]) |
||||
manager = AnalyticUnitManager() |
||||
|
||||
with_manager = await self._test_detect(test_data, manager) |
||||
without_manager = await self._test_detect(test_data) |
||||
self.assertEqual(with_manager, without_manager) |
||||
|
@ -1,43 +0,0 @@
|
||||
import unittest |
||||
import pandas as pd |
||||
import numpy as np |
||||
import models |
||||
|
||||
class TestModel(unittest.TestCase): |
||||
|
||||
def test_stair_model_get_indexes(self): |
||||
drop_model = models.DropModel() |
||||
jump_model = models.JumpModel() |
||||
drop_data = pd.Series([4, 4, 4, 1, 1, 1, 5, 5, 2, 2, 2]) |
||||
jump_data = pd.Series([1, 1, 1, 4, 4, 4, 2, 2, 5, 5, 5]) |
||||
jump_data_one_stair = pd.Series([1, 3, 3]) |
||||
drop_data_one_stair = pd.Series([4, 2, 1]) |
||||
height = 2 |
||||
length = 2 |
||||
expected_result = [2, 7] |
||||
drop_model_result = drop_model.get_stair_indexes(drop_data, height, length) |
||||
jump_model_result = jump_model.get_stair_indexes(jump_data, height, length) |
||||
drop_one_stair_result = drop_model.get_stair_indexes(drop_data_one_stair, height, 1) |
||||
jump_one_stair_result = jump_model.get_stair_indexes(jump_data_one_stair, height, 1) |
||||
for val in expected_result: |
||||
self.assertIn(val, drop_model_result) |
||||
self.assertIn(val, jump_model_result) |
||||
self.assertEqual(0, drop_one_stair_result[0]) |
||||
self.assertEqual(0, jump_one_stair_result[0]) |
||||
|
||||
def test_stair_model_get_indexes_corner_cases(self): |
||||
drop_model = models.DropModel() |
||||
jump_model = models.JumpModel() |
||||
empty_data = pd.Series([]) |
||||
nan_data = pd.Series([np.nan, np.nan, np.nan, np.nan]) |
||||
height, length = 2, 2 |
||||
length_zero, height_zero = 0, 0 |
||||
expected_result = [] |
||||
drop_empty_data_result = drop_model.get_stair_indexes(empty_data, height, length) |
||||
drop_nan_data_result = drop_model.get_stair_indexes(nan_data, height_zero, length_zero) |
||||
jump_empty_data_result = jump_model.get_stair_indexes(empty_data, height, length) |
||||
jump_nan_data_result = jump_model.get_stair_indexes(nan_data, height_zero, length_zero) |
||||
self.assertEqual(drop_empty_data_result, expected_result) |
||||
self.assertEqual(drop_nan_data_result, expected_result) |
||||
self.assertEqual(jump_empty_data_result, expected_result) |
||||
self.assertEqual(jump_nan_data_result, expected_result) |
@ -1,359 +0,0 @@
|
||||
from analytic_types.segment import Segment |
||||
|
||||
import utils |
||||
import unittest |
||||
import numpy as np |
||||
import pandas as pd |
||||
import math |
||||
import random |
||||
|
||||
RELATIVE_TOLERANCE = 1e-1 |
||||
|
||||
class TestUtils(unittest.TestCase): |
||||
|
||||
#example test for test's workflow purposes |
||||
def test_segment_parsion(self): |
||||
self.assertTrue(True) |
||||
|
||||
def test_confidence_all_normal_value(self): |
||||
segment = [1, 2, 0, 6, 8, 5, 3] |
||||
utils_result = utils.find_confidence(segment)[0] |
||||
result = 4.0 |
||||
self.assertTrue(math.isclose(utils_result, result, rel_tol = RELATIVE_TOLERANCE)) |
||||
|
||||
def test_confidence_all_nan_value(self): |
||||
segment = [np.nan, np.nan, np.nan, np.nan] |
||||
self.assertEqual(utils.find_confidence(segment)[0], 0) |
||||
|
||||
def test_confidence_with_nan_value(self): |
||||
data = [np.nan, np.nan, 0, 8] |
||||
utils_result = utils.find_confidence(data)[0] |
||||
result = 4.0 |
||||
self.assertTrue(math.isclose(utils_result, result, rel_tol = RELATIVE_TOLERANCE)) |
||||
|
||||
def test_interval_all_normal_value(self): |
||||
data = [1, 2, 1, 2, 4, 1, 2, 4, 5, 6] |
||||
data = pd.Series(data) |
||||
center = 4 |
||||
window_size = 2 |
||||
result = [1, 2, 4, 1, 2] |
||||
self.assertEqual(list(utils.get_interval(data, center, window_size)), result) |
||||
|
||||
def test_interval_wrong_ws(self): |
||||
data = [1, 2, 4, 1, 2, 4] |
||||
data = pd.Series(data) |
||||
center = 3 |
||||
window_size = 6 |
||||
result = [1, 2, 4, 1, 2, 4] |
||||
self.assertEqual(list(utils.get_interval(data, center, window_size)), result) |
||||
|
||||
def test_subtract_min_without_nan(self): |
||||
segment = [1, 2, 4, 1, 2, 4] |
||||
segment = pd.Series(segment) |
||||
result = [0, 1, 3, 0, 1, 3] |
||||
utils_result = list(utils.subtract_min_without_nan(segment)) |
||||
self.assertEqual(utils_result, result) |
||||
|
||||
def test_subtract_min_with_nan(self): |
||||
segment = [np.nan, 2, 4, 1, 2, 4] |
||||
segment = pd.Series(segment) |
||||
result = [2, 4, 1, 2, 4] |
||||
utils_result = list(utils.subtract_min_without_nan(segment)[1:]) |
||||
self.assertEqual(utils_result, result) |
||||
|
||||
def test_get_convolve(self): |
||||
data = [1, 2, 3, 2, 2, 0, 2, 3, 4, 3, 2, 1, 1, 2, 3, 4, 3, 2, 0] |
||||
data = pd.Series(data) |
||||
pattern_index = [2, 8, 15] |
||||
window_size = 2 |
||||
av_model = [1, 2, 3, 2, 1] |
||||
result = [] |
||||
self.assertNotEqual(utils.get_convolve(pattern_index, av_model, data, window_size), result) |
||||
|
||||
def test_get_convolve_with_nan(self): |
||||
data = [1, 2, 3, 2, np.nan, 0, 2, 3, 4, np.nan, 2, 1, 1, 2, 3, 4, 3, np.nan, 0] |
||||
data = pd.Series(data) |
||||
pattern_index = [2, 8, 15] |
||||
window_size = 2 |
||||
av_model = [1, 2, 3, 2, 1] |
||||
result = utils.get_convolve(pattern_index, av_model, data, window_size) |
||||
for val in result: |
||||
self.assertFalse(np.isnan(val)) |
||||
|
||||
def test_get_convolve_empty_data(self): |
||||
data = [] |
||||
pattern_index = [] |
||||
window_size = 2 |
||||
window_size_zero = 0 |
||||
av_model = [] |
||||
result = [] |
||||
self.assertEqual(utils.get_convolve(pattern_index, av_model, data, window_size), result) |
||||
self.assertEqual(utils.get_convolve(pattern_index, av_model, data, window_size_zero), result) |
||||
|
||||
def test_find_jump_parameters_center(self): |
||||
segment = [1, 1, 1, 1, 1, 1, 1, 1, 1, 1, 1, 3, 5, 5, 5, 5, 5, 5, 5, 5, 5, 5, 5, 5, 5, 5, 5] |
||||
segment = pd.Series(segment) |
||||
jump_center = [10, 11] |
||||
self.assertIn(utils.find_pattern_center(segment, 0, 'jump'), jump_center) |
||||
|
||||
def test_find_jump_parameters_height(self): |
||||
segment = [1, 1, 1, 1, 1, 1, 1, 1, 1, 1, 1, 3, 5, 5, 5, 5, 5, 5, 5, 5, 5, 5, 5, 5, 5, 5, 5] |
||||
segment = pd.Series(segment) |
||||
jump_height = [3.5, 4] |
||||
self.assertGreaterEqual(utils.find_parameters(segment, 0, 'jump')[0], jump_height[0]) |
||||
self.assertLessEqual(utils.find_parameters(segment, 0, 'jump')[0], jump_height[1]) |
||||
|
||||
def test_find_jump_parameters_length(self): |
||||
segment = [1, 1, 1, 1, 1, 1, 1, 1, 1, 1, 1, 3, 5, 5, 5, 5, 5, 5, 5, 5, 5, 5, 5, 5, 5, 5, 5] |
||||
segment = pd.Series(segment) |
||||
jump_length = 2 |
||||
self.assertEqual(utils.find_parameters(segment, 0, 'jump')[1], jump_length) |
||||
|
||||
def test_find_drop_parameters_center(self): |
||||
segment = [5, 5, 5, 5, 5, 5, 5, 5, 5, 5, 5, 5, 5, 5, 5, 3, 1, 1, 1, 1, 1, 1, 1, 1, 1, 1, 1] |
||||
segment = pd.Series(segment) |
||||
drop_center = [14, 15, 16] |
||||
self.assertIn(utils.find_pattern_center(segment, 0, 'drop'), drop_center) |
||||
|
||||
def test_find_drop_parameters_height(self): |
||||
segment = [5, 5, 5, 5, 5, 5, 5, 5, 5, 5, 5, 5, 5, 5, 5, 3, 1, 1, 1, 1, 1, 1, 1, 1, 1, 1, 1] |
||||
segment = pd.Series(segment) |
||||
drop_height = [3.5, 4] |
||||
self.assertGreaterEqual(utils.find_parameters(segment, 0, 'drop')[0], drop_height[0]) |
||||
self.assertLessEqual(utils.find_parameters(segment, 0, 'drop')[0], drop_height[1]) |
||||
|
||||
def test_find_drop_parameters_length(self): |
||||
segment = [5, 5, 5, 5, 5, 5, 5, 5, 5, 5, 5, 5, 5, 5, 5, 3, 1, 1, 1, 1, 1, 1, 1, 1, 1, 1, 1] |
||||
segment = pd.Series(segment) |
||||
drop_length = 2 |
||||
self.assertEqual(utils.find_parameters(segment, 0, 'drop')[1], drop_length) |
||||
|
||||
def test_get_av_model_empty_data(self): |
||||
patterns_list = [] |
||||
result = [] |
||||
self.assertEqual(utils.get_av_model(patterns_list), result) |
||||
|
||||
def test_get_av_model_normal_data(self): |
||||
patterns_list = [[1, 1, 1], [2, 2, 2],[3,3,3]] |
||||
result = [2.0, 2.0, 2.0] |
||||
self.assertEqual(utils.get_av_model(patterns_list), result) |
||||
|
||||
def test_get_distribution_density(self): |
||||
segment = [1, 1, 1, 3, 5, 5, 5] |
||||
segment = pd.Series(segment) |
||||
result = (3, 5, 1) |
||||
self.assertEqual(utils.get_distribution_density(segment), result) |
||||
|
||||
def test_get_distribution_density_right(self): |
||||
data = [1.0, 5.0, 5.0, 4.0] |
||||
data = pd.Series(data) |
||||
median = 3.0 |
||||
max_line = 5.0 |
||||
min_line = 1.0 |
||||
utils_result = utils.get_distribution_density(data) |
||||
self.assertTrue(math.isclose(utils_result[0], median, rel_tol = RELATIVE_TOLERANCE)) |
||||
self.assertTrue(math.isclose(utils_result[1], max_line, rel_tol = RELATIVE_TOLERANCE)) |
||||
self.assertTrue(math.isclose(utils_result[2], min_line, rel_tol = RELATIVE_TOLERANCE)) |
||||
|
||||
def test_get_distribution_density_left(self): |
||||
data = [1.0, 1.0, 2.0, 1.0, 5.0] |
||||
data = pd.Series(data) |
||||
median = 3.0 |
||||
max_line = 5.0 |
||||
min_line = 1.0 |
||||
utils_result = utils.get_distribution_density(data) |
||||
self.assertTrue(math.isclose(utils_result[0], median, rel_tol = RELATIVE_TOLERANCE)) |
||||
self.assertTrue(math.isclose(utils_result[1], max_line, rel_tol = RELATIVE_TOLERANCE)) |
||||
self.assertTrue(math.isclose(utils_result[2], min_line, rel_tol = RELATIVE_TOLERANCE)) |
||||
|
||||
def test_get_distribution_density_short_data(self): |
||||
data = [1.0, 5.0] |
||||
data = pd.Series(data) |
||||
segment = [1.0] |
||||
segment = pd.Series(segment) |
||||
utils_result_data = utils.get_distribution_density(data) |
||||
utils_result_segment = utils.get_distribution_density(segment) |
||||
self.assertEqual(len(utils_result_data), 3) |
||||
self.assertEqual(utils_result_segment, (0, 0, 0)) |
||||
|
||||
def test_get_distribution_density_with_nans(self): |
||||
segment = [np.NaN, 1, 1, 1, np.NaN, 3, 5, 5, 5, np.NaN] |
||||
segment = pd.Series(segment) |
||||
result = (3, 5, 1) |
||||
self.assertEqual(utils.get_distribution_density(segment), result) |
||||
|
||||
def test_find_pattern_jump_center(self): |
||||
data = [1.0, 1.0, 1.0, 5.0, 5.0, 5.0] |
||||
data = pd.Series(data) |
||||
median = 3.0 |
||||
result = 3 |
||||
self.assertEqual(result, utils.find_pattern_center(data, 0, 'jump')) |
||||
|
||||
def test_get_convolve_wrong_index(self): |
||||
data = [1.0, 5.0, 2.0, 1.0, 6.0, 2.0] |
||||
data = pd.Series(data) |
||||
segemnts = [1, 11] |
||||
av_model = [0.0, 4.0, 0.0] |
||||
window_size = 1 |
||||
try: |
||||
utils.get_convolve(segemnts, av_model, data, window_size) |
||||
except ValueError: |
||||
self.fail('Method get_convolve raised unexpectedly') |
||||
|
||||
def test_get_av_model_for_different_length(self): |
||||
patterns_list = [[1.0, 1.0, 2.0], [4.0, 4.0], [2.0, 2.0, 2.0], [3.0, 3.0], []] |
||||
try: |
||||
utils.get_av_model(patterns_list) |
||||
except ValueError: |
||||
self.fail('Method get_convolve raised unexpectedly') |
||||
|
||||
def test_find_nan_indexes(self): |
||||
data = [1, 1, 1, 0, 0, np.nan, None, []] |
||||
data = pd.Series(data) |
||||
result = [5, 6] |
||||
self.assertEqual(utils.find_nan_indexes(data), result) |
||||
|
||||
def test_find_nan_indexes_normal_values(self): |
||||
data = [1, 1, 1, 0, 0, 0, 1, 1] |
||||
data = pd.Series(data) |
||||
result = [] |
||||
self.assertEqual(utils.find_nan_indexes(data), result) |
||||
|
||||
def test_find_nan_indexes_empty_values(self): |
||||
data = [] |
||||
result = [] |
||||
self.assertEqual(utils.find_nan_indexes(data), result) |
||||
|
||||
def test_create_correlation_data(self): |
||||
data = [random.randint(10, 999) for _ in range(10000)] |
||||
data = pd.Series(data) |
||||
pattern_model = [100, 200, 500, 300, 100] |
||||
ws = 2 |
||||
result = 6000 |
||||
corr_data = utils.get_correlation_gen(data, ws, pattern_model) |
||||
corr_data = list(corr_data) |
||||
self.assertGreaterEqual(len(corr_data), result) |
||||
|
||||
def test_inverse_segment(self): |
||||
data = pd.Series([1,2,3,4,3,2,1]) |
||||
result = pd.Series([3,2,1,0,1,2,3]) |
||||
utils_result = utils.inverse_segment(data) |
||||
for ind, val in enumerate(utils_result): |
||||
self.assertEqual(val, result[ind]) |
||||
|
||||
def test_get_end_of_segment_equal(self): |
||||
data = pd.Series([5,4,3,2,1,0,0,0]) |
||||
result_list = [4, 5, 6] |
||||
self.assertIn(utils.get_end_of_segment(data, False), result_list) |
||||
|
||||
def test_get_end_of_segment_greater(self): |
||||
data = pd.Series([5,4,3,2,1,0,1,2,3]) |
||||
result_list = [4, 5, 6] |
||||
self.assertIn(utils.get_end_of_segment(data, False), result_list) |
||||
|
||||
def test_get_borders_of_peaks(self): |
||||
data = pd.Series([1,0,1,2,3,2,1,0,0,1,2,3,4,3,2,2,1,0,1,2,3,4,5,3,2,1,0]) |
||||
pattern_center = [4, 12, 22] |
||||
ws = 3 |
||||
confidence = 1.5 |
||||
result = [(1, 7), (9, 15), (19, 25)] |
||||
self.assertEqual(utils.get_borders_of_peaks(pattern_center, data, ws, confidence), result) |
||||
|
||||
def test_get_borders_of_peaks_for_trough(self): |
||||
data = pd.Series([4,4,5,5,3,1,3,5,5,6,3,2]) |
||||
pattern_center = [5] |
||||
ws = 5 |
||||
confidence = 3 |
||||
result = [(3, 7)] |
||||
self.assertEqual(utils.get_borders_of_peaks(pattern_center, data, ws, confidence, inverse = True), result) |
||||
|
||||
def test_get_start_and_end_of_segments(self): |
||||
segments = [[1, 2, 3, 4], [5, 6, 7], [8], [], [12, 12]] |
||||
result = [[1, 4], [5, 7], [8, 8], [12, 12]] |
||||
utils_result = utils.get_start_and_end_of_segments(segments) |
||||
for got, expected in zip(utils_result, result): |
||||
self.assertEqual(got, expected) |
||||
|
||||
def test_get_start_and_end_of_segments_empty(self): |
||||
segments = [] |
||||
result = [] |
||||
utils_result = utils.get_start_and_end_of_segments(segments) |
||||
self.assertEqual(result, utils_result) |
||||
|
||||
def test_merge_intersecting_segments(self): |
||||
test_cases = [ |
||||
{ |
||||
'index': [Segment(10, 20), Segment(30, 40)], |
||||
'result': [[10, 20], [30, 40]], |
||||
'step': 0, |
||||
}, |
||||
{ |
||||
'index': [Segment(10, 20), Segment(13, 23), Segment(15, 17), Segment(20, 40)], |
||||
'result': [[10, 40]], |
||||
'step': 0, |
||||
}, |
||||
{ |
||||
'index': [], |
||||
'result': [], |
||||
'step': 0, |
||||
}, |
||||
{ |
||||
'index': [Segment(10, 20)], |
||||
'result': [[10, 20]], |
||||
'step': 0, |
||||
}, |
||||
{ |
||||
'index': [Segment(10, 20), Segment(13, 23), Segment(25, 30), Segment(35, 40)], |
||||
'result': [[10, 23], [25, 30], [35, 40]], |
||||
'step': 0, |
||||
}, |
||||
{ |
||||
'index': [Segment(10, 50), Segment(5, 40), Segment(15, 25), Segment(6, 50)], |
||||
'result': [[5, 50]], |
||||
'step': 0, |
||||
}, |
||||
{ |
||||
'index': [Segment(5, 10), Segment(10, 20), Segment(25, 50)], |
||||
'result': [[5, 20], [25, 50]], |
||||
'step': 0, |
||||
}, |
||||
{ |
||||
'index': [Segment(20, 40), Segment(10, 15), Segment(50, 60)], |
||||
'result': [[10, 15], [20, 40], [50, 60]], |
||||
'step': 0, |
||||
}, |
||||
{ |
||||
'index': [Segment(20, 40), Segment(10, 20), Segment(50, 60)], |
||||
'result': [[10, 40], [50, 60]], |
||||
'step': 0, |
||||
}, |
||||
{ |
||||
'index': [Segment(10, 10), Segment(20, 20), Segment(30, 30)], |
||||
'result': [[10, 30]], |
||||
'step': 10, |
||||
}, |
||||
] |
||||
|
||||
for case in test_cases: |
||||
utils_result = utils.merge_intersecting_segments(case['index'], case['step']) |
||||
for got, expected in zip(utils_result, case['result']): |
||||
self.assertEqual(got.from_timestamp, expected[0]) |
||||
self.assertEqual(got.to_timestamp, expected[1]) |
||||
|
||||
def test_serialize(self): |
||||
segment_list = [Segment(100,200)] |
||||
serialize_list = utils.meta.SerializableList(segment_list) |
||||
meta_result = utils.meta.serialize(serialize_list) |
||||
expected_result = [{ 'from': 100, 'to': 200 }] |
||||
self.assertEqual(meta_result, expected_result) |
||||
|
||||
def test_remove_duplicates_and_sort(self): |
||||
a1 = [1, 3, 5] |
||||
a2 = [8, 3, 6] |
||||
expected_result = [1, 3, 5, 6, 8] |
||||
utils_result = utils.remove_duplicates_and_sort(a1+a2) |
||||
self.assertEqual(utils_result, expected_result) |
||||
self.assertEqual([], []) |
||||
|
||||
if __name__ == '__main__': |
||||
unittest.main() |
@ -1,43 +0,0 @@
|
||||
import unittest |
||||
from utils import get_intersected_chunks, get_chunks |
||||
import pandas as pd |
||||
|
||||
|
||||
class TestUtils(unittest.TestCase): |
||||
|
||||
def test_chunks_generator(self): |
||||
intersection = 2 |
||||
chunk_size = 4 |
||||
|
||||
cases = [ |
||||
(list(range(8)), [[0,1,2,3], [2,3,4,5], [4,5,6,7]]), |
||||
([], [[]]), |
||||
(list(range(1)), [[0]]), |
||||
(list(range(4)), [[0,1,2,3]]), |
||||
(list(range(9)), [[0,1,2,3], [2,3,4,5], [4,5,6,7], [6,7,8]]) |
||||
] |
||||
|
||||
for tested, expected in cases: |
||||
tested_chunks = get_intersected_chunks(tested, intersection, chunk_size) |
||||
self.assertSequenceEqual(tuple(tested_chunks), expected) |
||||
|
||||
|
||||
def test_non_intersected_chunks(self): |
||||
chunk_size = 4 |
||||
|
||||
cases = [ |
||||
(tuple(range(12)), [[0,1,2,3], [4,5,6,7], [8,9,10,11]]), |
||||
(tuple(range(9)), [[0,1,2,3], [4,5,6,7], [8]]), |
||||
(tuple(range(10)), [[0,1,2,3], [4,5,6,7], [8,9]]), |
||||
(tuple(range(11)), [[0,1,2,3], [4,5,6,7], [8,9,10]]), |
||||
([], []), |
||||
(tuple(range(1)), [[0]]), |
||||
(tuple(range(4)), [[0,1,2,3]]) |
||||
] |
||||
|
||||
for tested, expected in cases: |
||||
tested_chunks = list(get_chunks(tested, chunk_size)) |
||||
self.assertSequenceEqual(tested_chunks, expected) |
||||
|
||||
if __name__ == '__main__': |
||||
unittest.main() |
@ -1,122 +0,0 @@
|
||||
import sys |
||||
ANALYTICS_PATH = '../analytics' |
||||
TESTS_PATH = '../tests' |
||||
sys.path.extend([ANALYTICS_PATH, TESTS_PATH]) |
||||
|
||||
import pandas as pd |
||||
import numpy as np |
||||
import utils |
||||
import test_dataset |
||||
from analytic_types.segment import Segment |
||||
from detectors import pattern_detector, threshold_detector, anomaly_detector |
||||
|
||||
# TODO: get_dataset |
||||
# TODO: get_segment |
||||
PEAK_DATASETS = [] |
||||
# dataset with 3 peaks |
||||
TEST_DATA = test_dataset.create_dataframe([0, 0, 3, 5, 7, 5, 3, 0, 0, 1, 0, 1, 4, 6, 8, 6, 4, 1, 0, 0, 0, 1, 0, 3, 5, 7, 5, 3, 0, 1, 1]) |
||||
# TODO: more convenient way to specify labeled segments |
||||
POSITIVE_SEGMENTS = [{'from': 1523889000001, 'to': 1523889000007}, {'from': 1523889000022, 'to': 1523889000028}] |
||||
NEGATIVE_SEGMENTS = [{'from': 1523889000011, 'to': 1523889000017}] |
||||
|
||||
class TesterSegment(): |
||||
|
||||
def __init__(self, start: int, end: int, labeled: bool): |
||||
self.start = start |
||||
self.end = end |
||||
self.labeled = labeled |
||||
|
||||
def get_segment(self): |
||||
return { |
||||
'_id': 'q', |
||||
'analyticUnitId': 'q', |
||||
'from': self.start, |
||||
'to': self.end, |
||||
'labeled': self.labeled, |
||||
'deleted': not self.labeled |
||||
} |
||||
|
||||
class Metric(): |
||||
|
||||
def __init__(self, expected_result, detector_result): |
||||
self.expected_result = expected_result |
||||
self.detector_result = detector_result['segments'] |
||||
|
||||
def get_amount(self): |
||||
return len(self.detector_result) / len(self.expected_result) |
||||
|
||||
def get_accuracy(self): |
||||
correct_segment = 0 |
||||
invalid_segment = 0 |
||||
for segment in self.detector_result: |
||||
current_cs = correct_segment |
||||
for pattern in self.expected_result: |
||||
if pattern['from'] <= segment['from'] and pattern['to'] >= segment['to']: |
||||
correct_segment += 1 |
||||
break |
||||
if correct_segment == current_cs: |
||||
invalid_segment += 1 |
||||
non_detected = len(self.expected_result) - correct_segment |
||||
return (correct_segment, invalid_segment, non_detected) |
||||
|
||||
class ModelData(): |
||||
|
||||
def __init__(self, frame: pd.DataFrame, positive_segments, negative_segments, model_type: str): |
||||
self.frame = frame |
||||
self.positive_segments = positive_segments |
||||
self.negative_segments = negative_segments |
||||
self.model_type = model_type |
||||
|
||||
def get_segments_for_detection(self, positive_amount, negative_amount): |
||||
segments = [] |
||||
for idx, bounds in enumerate(self.positive_segments): |
||||
if idx >= positive_amount: |
||||
break |
||||
segments.append(TesterSegment(bounds['from'], bounds['to'], True).get_segment()) |
||||
|
||||
for idx, bounds in enumerate(self.negative_segments): |
||||
if idx >= negative_amount: |
||||
break |
||||
segments.append(TesterSegment(bounds['from'], bounds['to'], False).get_segment()) |
||||
|
||||
return segments |
||||
|
||||
def get_all_correct_segments(self): |
||||
return self.positive_segments |
||||
|
||||
PEAK_DATA_1 = ModelData(TEST_DATA, POSITIVE_SEGMENTS, NEGATIVE_SEGMENTS, 'peak') |
||||
PEAK_DATASETS.append(PEAK_DATA_1) |
||||
|
||||
def main(model_type: str) -> None: |
||||
table_metric = [] |
||||
if model_type == 'peak': |
||||
for data in PEAK_DATASETS: |
||||
dataset = data.frame |
||||
segments = data.get_segments_for_detection(1, 0) |
||||
segments = [Segment.from_json(segment) for segment in segments] |
||||
detector = pattern_detector.PatternDetector('PEAK', 'test_id') |
||||
training_result = detector.train(dataset, segments, {}) |
||||
cache = training_result['cache'] |
||||
detect_result = detector.detect(dataset, cache) |
||||
detect_result = detect_result.to_json() |
||||
peak_metric = Metric(data.get_all_correct_segments(), detect_result) |
||||
table_metric.append((peak_metric.get_amount(), peak_metric.get_accuracy())) |
||||
return table_metric |
||||
|
||||
if __name__ == '__main__': |
||||
''' |
||||
This tool applies the model on datasets and verifies that the detection result corresponds to the correct values. |
||||
sys.argv[1] expects one of the models name -> see correct_name |
||||
''' |
||||
# TODO: use enum |
||||
correct_name = ['peak', 'trough', 'jump', 'drop', 'general'] |
||||
if len(sys.argv) < 2: |
||||
print('Enter one of models name: {}'.format(correct_name)) |
||||
sys.exit(1) |
||||
model_type = str(sys.argv[1]).lower() |
||||
if model_type in correct_name: |
||||
print(main(model_type)) |
||||
else: |
||||
print('Enter one of models name: {}'.format(correct_name)) |
||||
|
||||
|
@ -1,104 +0,0 @@
|
||||
import zmq |
||||
import zmq.asyncio |
||||
import asyncio |
||||
import json |
||||
from uuid import uuid4 |
||||
|
||||
context = zmq.asyncio.Context() |
||||
socket = context.socket(zmq.PAIR) |
||||
socket.connect('tcp://0.0.0.0:8002') |
||||
|
||||
def create_message(): |
||||
message = { |
||||
"method": "DATA", |
||||
"payload": { |
||||
"_id": uuid4().hex, |
||||
"analyticUnitId": uuid4().hex, |
||||
"type": "PUSH", |
||||
"payload": { |
||||
"data": [ |
||||
[ |
||||
1552652025000, |
||||
12.499999999999998 |
||||
], |
||||
[ |
||||
1552652040000, |
||||
12.500000000000002 |
||||
], |
||||
[ |
||||
1552652055000, |
||||
12.499999999999996 |
||||
], |
||||
[ |
||||
1552652070000, |
||||
12.500000000000002 |
||||
], |
||||
[ |
||||
1552652085000, |
||||
12.499999999999998 |
||||
], |
||||
[ |
||||
1552652100000, |
||||
12.5 |
||||
], |
||||
[ |
||||
1552652115000, |
||||
12.83261113785909 |
||||
] |
||||
], |
||||
"from": 1552652025001, |
||||
"to": 1552652125541, |
||||
"analyticUnitType": "GENERAL", |
||||
"detector": "pattern", |
||||
"cache": { |
||||
"pattern_center": [ |
||||
693 |
||||
], |
||||
"pattern_model": [ |
||||
1.7763568394002505e-15, |
||||
5.329070518200751e-15, |
||||
1.7763568394002505e-15, |
||||
1.7763568394002505e-15, |
||||
1.7763568394002505e-15, |
||||
3.552713678800501e-15, |
||||
1.7763568394002505e-15, |
||||
3.552713678800501e-15, |
||||
3.552713678800501e-15, |
||||
1.7763568394002505e-15, |
||||
1.7763568394002505e-15, |
||||
0, |
||||
1.7763568394002505e-15, |
||||
1.7763568394002505e-15, |
||||
0 |
||||
], |
||||
"convolve_max": 7.573064690121713e-29, |
||||
"convolve_min": 7.573064690121713e-29, |
||||
"WINDOW_SIZE": 7, |
||||
"conv_del_min": 7, |
||||
"conv_del_max": 7 |
||||
} |
||||
} |
||||
} |
||||
} |
||||
|
||||
return json.dumps(message) |
||||
|
||||
async def handle_loop(): |
||||
while True: |
||||
received_bytes = await socket.recv() |
||||
text = received_bytes.decode('utf-8') |
||||
|
||||
print(text) |
||||
|
||||
async def send_detect(): |
||||
data = create_message().encode('utf-8') |
||||
await socket.send(data) |
||||
|
||||
if __name__ == "__main__": |
||||
loop = asyncio.get_event_loop() |
||||
socket.send(b'PING') |
||||
detects = [send_detect() for i in range(100)] |
||||
detects_group = asyncio.gather(*detects) |
||||
handle_group = asyncio.gather(handle_loop()) |
||||
common_group = asyncio.gather(handle_group, detects_group) |
||||
loop.run_until_complete(common_group) |
Loading…
Reference in new issue